Packet Duplication for URLLC in 5G: Architectural Enhancements and Performance Analysis.
IEEE Network(2018)SCI 1区SCI 2区
Huawei Technol Canada
Abstract
URLLC use cases demand a new paradigm in cellular networks to contend with the extreme requirements with complex trade-offs. In general, it is exceptionally challenging and, resource usage-wise, prohibitively expensive to satisfy the URLLC requirements using the existing approaches in LTE. To address these challenges 3GPP has recently agreed to adopt PD of both UP and CP packets as a fundamental technique in 5G NR. This article investigates the theoretic framework behind PD and provides a primer on the recent enhancements applied in the NR RAN architecture for supporting URLLC. It is shown that PD enables jointly satisfying the latency and reliability requirements without increasing the complexity in the RAN. With dynamic control capability, PD can be used not only for URLLC but also to increase the transmission robustness during mobility and against radio link failures. The article also provides numerical results comparing the performance of PD in various deployment scenarios. The numerical results reveal that in certain scenarios, performing PD over multiple links results in lower usage of radio resources than using a single highly reliable link. It is also found that to improve radio resource utilization while satisfying URLLC requirements, enabling PD in scenarios such as cell edge is crucial where the average SNR of the best (primary) link and the variation in SNR between all accessible links is typically low. In essence, the PD technique provides a cost-effective solution for satisfying the URLLC requirements without requiring major modifications to the RAN deployments.
MoreTranslated text
Key words
Long Term Evolution,Protocols,Reliability theory,5G mobile communication,Signal to noise ratio,Quality of service
PDF
View via Publisher
AI Read Science
AI Summary
AI Summary is the key point extracted automatically understanding the full text of the paper, including the background, methods, results, conclusions, icons and other key content, so that you can get the outline of the paper at a glance.
Example
Background
Key content
Introduction
Methods
Results
Related work
Fund
Key content
- Pretraining has recently greatly promoted the development of natural language processing (NLP)
- We show that M6 outperforms the baselines in multimodal downstream tasks, and the large M6 with 10 parameters can reach a better performance
- We propose a method called M6 that is able to process information of multiple modalities and perform both single-modal and cross-modal understanding and generation
- The model is scaled to large model with 10 billion parameters with sophisticated deployment, and the 10 -parameter M6-large is the largest pretrained model in Chinese
- Experimental results show that our proposed M6 outperforms the baseline in a number of downstream tasks concerning both single modality and multiple modalities We will continue the pretraining of extremely large models by increasing data to explore the limit of its performance
Try using models to generate summary,it takes about 60s
Must-Reading Tree
Example
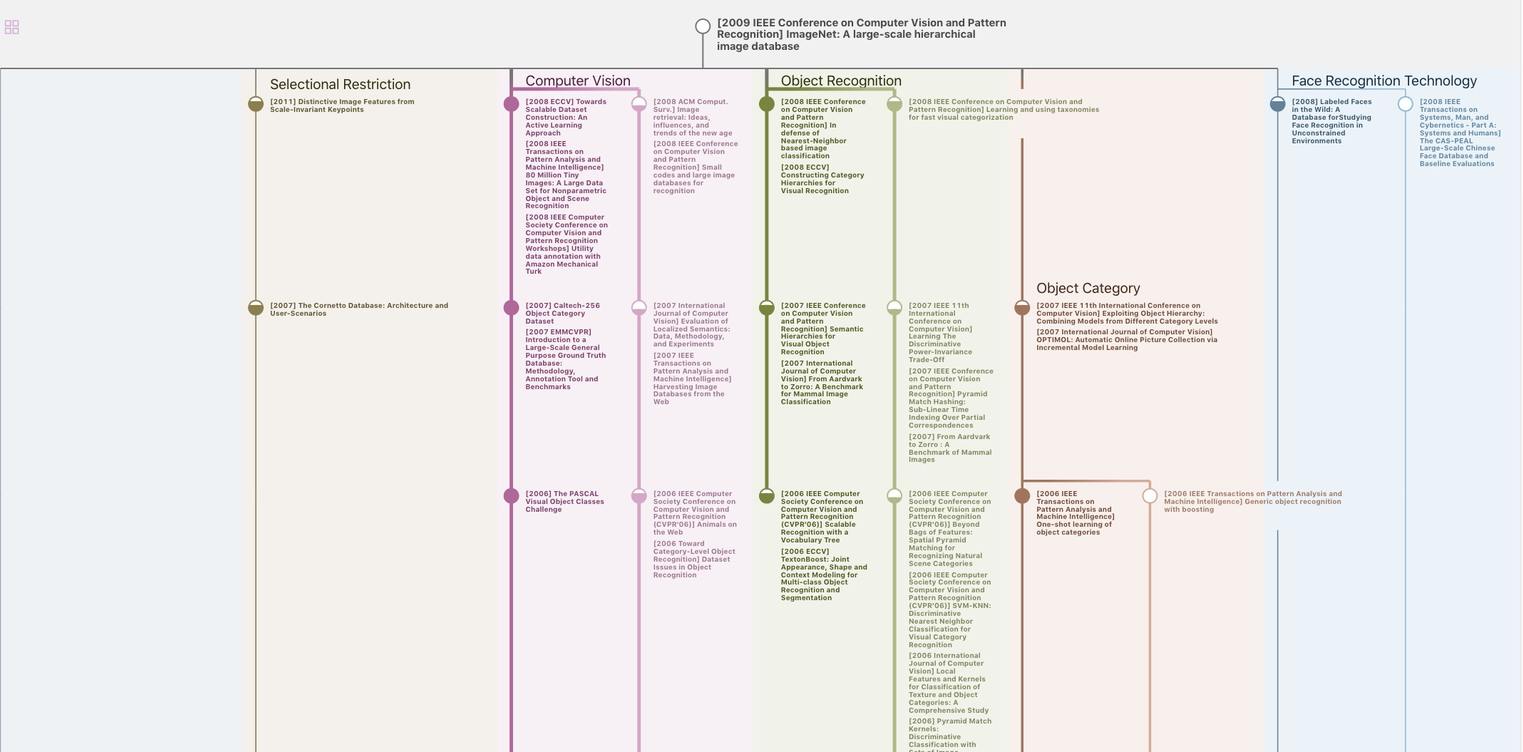
Generate MRT to find the research sequence of this paper
Related Papers
Opportunistic Spatial Preemptive Scheduling for URLLC and Embb Coexistence in Multi-User 5G Networks
2018
被引用87 | 浏览
2019
被引用11 | 浏览
2019
被引用10 | 浏览
2019
被引用5 | 浏览
2019
被引用1 | 浏览
2019
被引用0 | 浏览
2020
被引用3 | 浏览
2020
被引用6 | 浏览
2021
被引用18 | 浏览
2022
被引用10 | 浏览
2021
被引用3 | 浏览
2021
被引用7 | 浏览
2022
被引用0 | 浏览
Data Disclaimer
The page data are from open Internet sources, cooperative publishers and automatic analysis results through AI technology. We do not make any commitments and guarantees for the validity, accuracy, correctness, reliability, completeness and timeliness of the page data. If you have any questions, please contact us by email: report@aminer.cn
Chat Paper
去 AI 文献库 对话