Event-triggered Model Predictive Controller with Neural Network for Autonomous Rendezvous and Proximity on Elliptical Orbits
IEEE TRANSACTIONS ON AEROSPACE AND ELECTRONIC SYSTEMS(2024)
Abstract
Autonomous rendezvous and proximity operation (ARPO) is the basis for various on-orbit services. However, aiming for high-value targets and space debris located on elliptical orbits, ARPO becomes challenging for conventional guidance and control methods, due to the complexity and strong nonlinearity of dynamics. This article proposes an encoder-decoder network with an interconnecting branch between layers to enhance the ability of the neural network to operate ARPO on elliptical orbits. The neural network is trained through behavioral cloning, with expert trajectories collected from the optimization results of model predictive control. The performance of the proposed network architecture is effectively improved compared with the existing network architectures while the computational overhead is greatly reduced compared with model predictive control. We further propose an event-triggered neural network controller. It uses the neural network to calculate control inputs under normal circumstances to save computing resources and switches to model predictive control to ensure safety and improve control accuracy when events are triggered. To prevent the control input provided by the neural network from exceeding predefined boundaries, constraint violation corrections are added to ensure the safety of the transfer process. Adaptive performance enhancement is implemented to optimize the steady-state relative distance. This mechanism adaptively determines the control thresholds for model predictive controllers and neural network controllers. The proposed approach selects the appropriate controller according to the designed event-triggered conditions, thereby achieving a balance between efficiency and accuracy. Simulation with environment perturbations and sensor measurement noise demonstrates the effectiveness and robustness of the proposed controller.
MoreTranslated text
Key words
Orbits,Neural networks,Space vehicles,Predictive models,Predictive control,Satellites,Mathematical models,Behavioral cloning,imitation learning,model predictive control (MPC),neural network architecture,spacecraft rendezvous
求助PDF
上传PDF
View via Publisher
AI Read Science
AI Summary
AI Summary is the key point extracted automatically understanding the full text of the paper, including the background, methods, results, conclusions, icons and other key content, so that you can get the outline of the paper at a glance.
Example
Background
Key content
Introduction
Methods
Results
Related work
Fund
Key content
- Pretraining has recently greatly promoted the development of natural language processing (NLP)
- We show that M6 outperforms the baselines in multimodal downstream tasks, and the large M6 with 10 parameters can reach a better performance
- We propose a method called M6 that is able to process information of multiple modalities and perform both single-modal and cross-modal understanding and generation
- The model is scaled to large model with 10 billion parameters with sophisticated deployment, and the 10 -parameter M6-large is the largest pretrained model in Chinese
- Experimental results show that our proposed M6 outperforms the baseline in a number of downstream tasks concerning both single modality and multiple modalities We will continue the pretraining of extremely large models by increasing data to explore the limit of its performance
Upload PDF to Generate Summary
Must-Reading Tree
Example
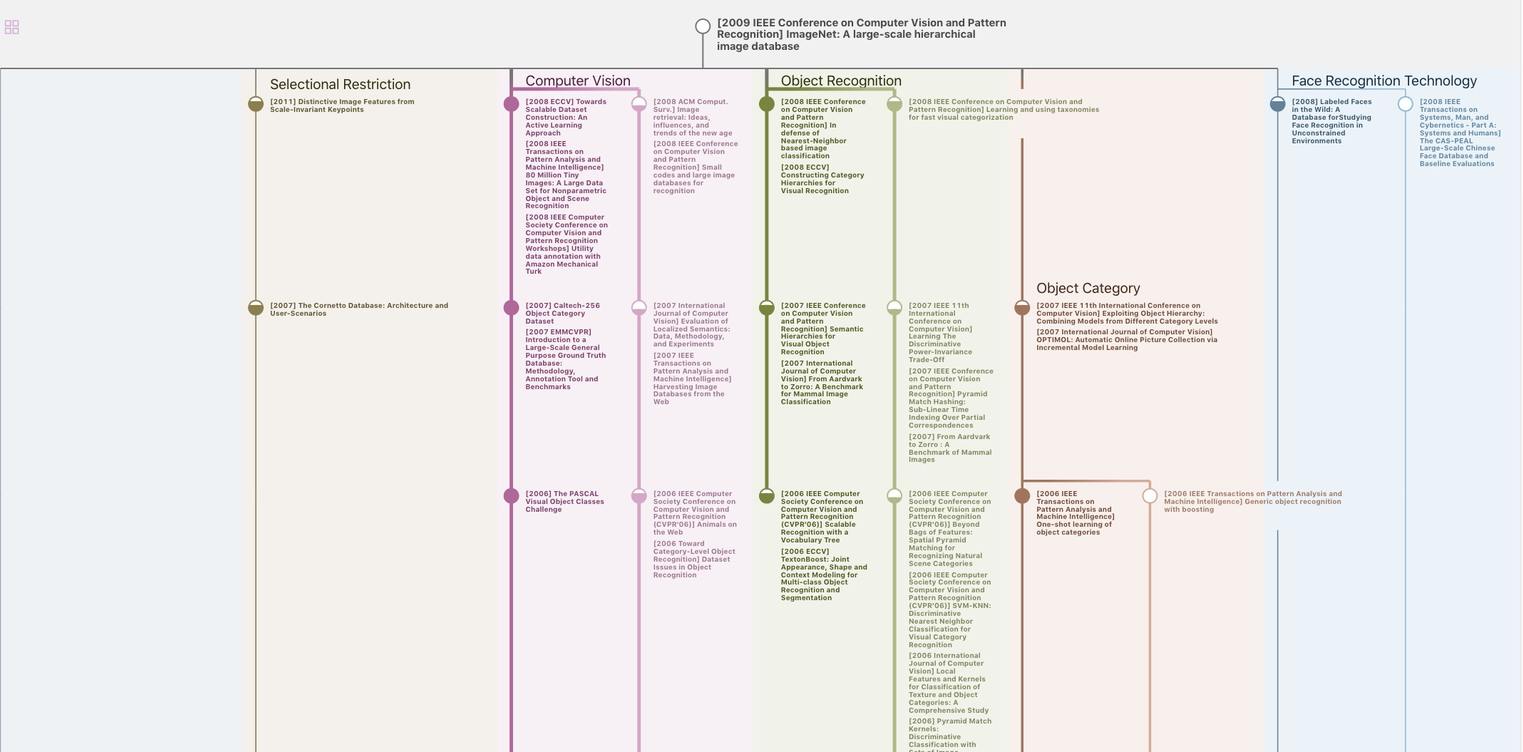
Generate MRT to find the research sequence of this paper
Data Disclaimer
The page data are from open Internet sources, cooperative publishers and automatic analysis results through AI technology. We do not make any commitments and guarantees for the validity, accuracy, correctness, reliability, completeness and timeliness of the page data. If you have any questions, please contact us by email: report@aminer.cn
Chat Paper
GPU is busy, summary generation fails
Rerequest