Using Mobile Daily Mood and Anxiety Self-ratings to Predict Depression Symptom Improvement
2024 IEEE/ACM CONFERENCE ON CONNECTED HEALTH APPLICATIONS, SYSTEMS AND ENGINEERING TECHNOLOGIES, CHASE 2024(2024)
Univ Connecticut
Abstract
Effective treatment of depression is important for the well-being of individuals and the overall health of the society. The current treatment approach calls for monitoring and assessing depression symptoms using self-administered or clinician-administered questionnaires, which are burdensome, costly, and may suffer from recall and desirability bias. In this study, we explore using daily 5-point Likert-scale mood and anxiety survey in place of burdensome clinical depression questionnaires for monitoring depression treatment. Specifically, we collect daily mood and anxiety surveys using a smartphone app, and use them to predict depression symptom improvement in a clinical depressed population. Using a dataset from 67 participants, we show that both mood and anxiety features obtained from the daily survey have significant correlation with clinical questionnaire scores. We then develop a family of machine learning models that use mood and anxiety features (separately or in combination) to predict symptom improvement status on a weekly basis. The best prediction F 1 score achieved by these models is 0.65. While this accuracy is lower than what is achieved by clinical questionnaires (best F 1 score being 0.71), daily survey is much less burdensome, and hence we believe that it provides a promising direction in monitoring depression symptom improvement. We further show that the prediction accuracy is not sensitive to missing data, allowing not very regular responses in practice. Last, we show that adding more historical data beyond the current week does not provide much benefits in improving prediction accuracy, and daily mood/anxiety self-ratings can predict improvement status accurately one or two weeks in the future.
MoreTranslated text
Key words
Depressive Symptoms,Improvement In Depressive Symptoms,Daily Mood,Machine Learning,Prediction Accuracy,Recall Bias,Machine Learning Models,F1 Score,Diagnosis Of Depression,Treatment Of Depression,Improve Prediction Accuracy,Day Of The Survey,Clinical Questionnaire,Assessment Of Depressive Symptoms,Discourage,Learning Algorithms,Support Vector Machine,Random Forest,Weeks Of Data,Mood Ratings,XGBoost,Radial Basis Function Kernel,Daily Data,Precision And Recall,Prediction Scenarios,Patient Health Questionnaire-9 Scores,Sequence Prediction,Sensor Data
求助PDF
上传PDF
View via Publisher
AI Read Science
AI Summary
AI Summary is the key point extracted automatically understanding the full text of the paper, including the background, methods, results, conclusions, icons and other key content, so that you can get the outline of the paper at a glance.
Example
Background
Key content
Introduction
Methods
Results
Related work
Fund
Key content
- Pretraining has recently greatly promoted the development of natural language processing (NLP)
- We show that M6 outperforms the baselines in multimodal downstream tasks, and the large M6 with 10 parameters can reach a better performance
- We propose a method called M6 that is able to process information of multiple modalities and perform both single-modal and cross-modal understanding and generation
- The model is scaled to large model with 10 billion parameters with sophisticated deployment, and the 10 -parameter M6-large is the largest pretrained model in Chinese
- Experimental results show that our proposed M6 outperforms the baseline in a number of downstream tasks concerning both single modality and multiple modalities We will continue the pretraining of extremely large models by increasing data to explore the limit of its performance
Upload PDF to Generate Summary
Must-Reading Tree
Example
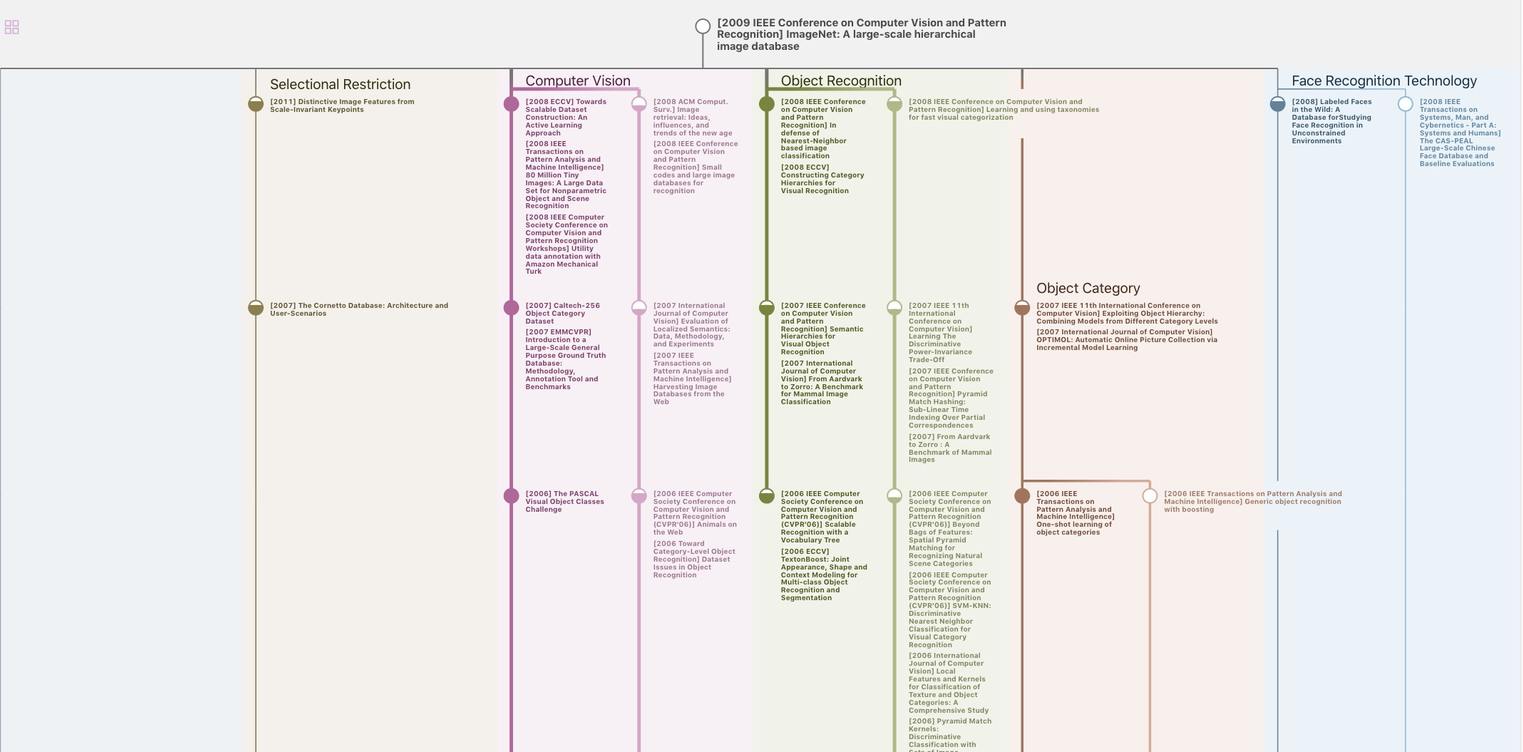
Generate MRT to find the research sequence of this paper
Data Disclaimer
The page data are from open Internet sources, cooperative publishers and automatic analysis results through AI technology. We do not make any commitments and guarantees for the validity, accuracy, correctness, reliability, completeness and timeliness of the page data. If you have any questions, please contact us by email: report@aminer.cn
Chat Paper