A BiLSTM and CTC Based Multi-Sensor Information Fusion Frame for Continuous Sign Language Recognition
2024 10TH INTERNATIONAL CONFERENCE ON ELECTRICAL ENGINEERING, CONTROL AND ROBOTICS, EECR 2024(2024)
关键词
continuous sign language recognition,multi-mode fusion,bi-directional long short-term memory,connection-ist temporary classification
AI 理解论文
溯源树
样例
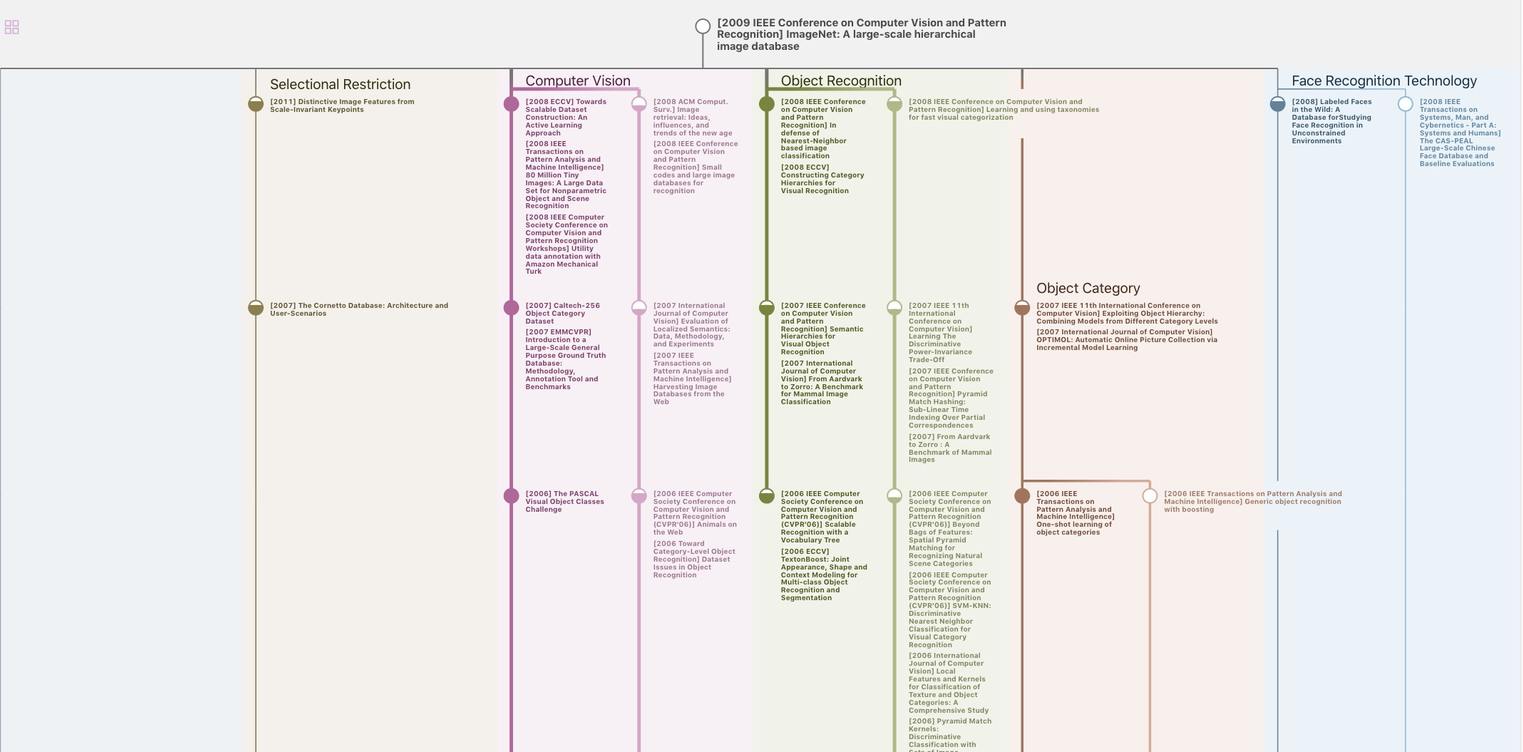
生成溯源树,研究论文发展脉络
Chat Paper
正在生成论文摘要