Mechanism and Barrier Modulation of Pt/TaO /HfO /TiN Self-Rectifying Devices
IEEE Transactions on Electron Devices(2024)
School of Integrated Circuit Science and Engineering | School of Materials Science and Engineering | TCL Huanxin Semiconductor (Tianjin) Company | Shanghai Institute of Microsystem and Information Technology
Abstract
In order to solve the crosstalk issue in a high-density memristor integrated array, the sneak path current in crossbar array should be effectively reduced. The self-rectifying memristor (SRM) becomes the best choice because of suppressing leakage current while exhibiting resistive characteristics without the need for external equipment, in which can improve array integration at a lower cost. In this article, an SRM with Pt/TaO
/HfO
/TiN structure is proposed. By modulating the thickness of the HfO
rectifying layer, the rectifying ratio of device can achieve as 3743 under
3 V with a sneak current below 10 nA and compliance current under 10
A. The self-rectification mechanism based on Schottky barrier modulation and oxygen vacancy defect traps is demonstrated by fitting analysis and interfacial barrier energy bands model. The maximum array size can reach 12 570 at the premise of 10% read margin with the device parameters. Our results provide a potential solution for the future application of memristor in the field of high-density memory integration.
MoreTranslated text
Key words
Crossbar array,interfacial barrier,memristor,self-rectifying,sneak current
求助PDF
上传PDF
View via Publisher
AI Read Science
AI Summary
AI Summary is the key point extracted automatically understanding the full text of the paper, including the background, methods, results, conclusions, icons and other key content, so that you can get the outline of the paper at a glance.
Example
Background
Key content
Introduction
Methods
Results
Related work
Fund
Key content
- Pretraining has recently greatly promoted the development of natural language processing (NLP)
- We show that M6 outperforms the baselines in multimodal downstream tasks, and the large M6 with 10 parameters can reach a better performance
- We propose a method called M6 that is able to process information of multiple modalities and perform both single-modal and cross-modal understanding and generation
- The model is scaled to large model with 10 billion parameters with sophisticated deployment, and the 10 -parameter M6-large is the largest pretrained model in Chinese
- Experimental results show that our proposed M6 outperforms the baseline in a number of downstream tasks concerning both single modality and multiple modalities We will continue the pretraining of extremely large models by increasing data to explore the limit of its performance
Upload PDF to Generate Summary
Must-Reading Tree
Example
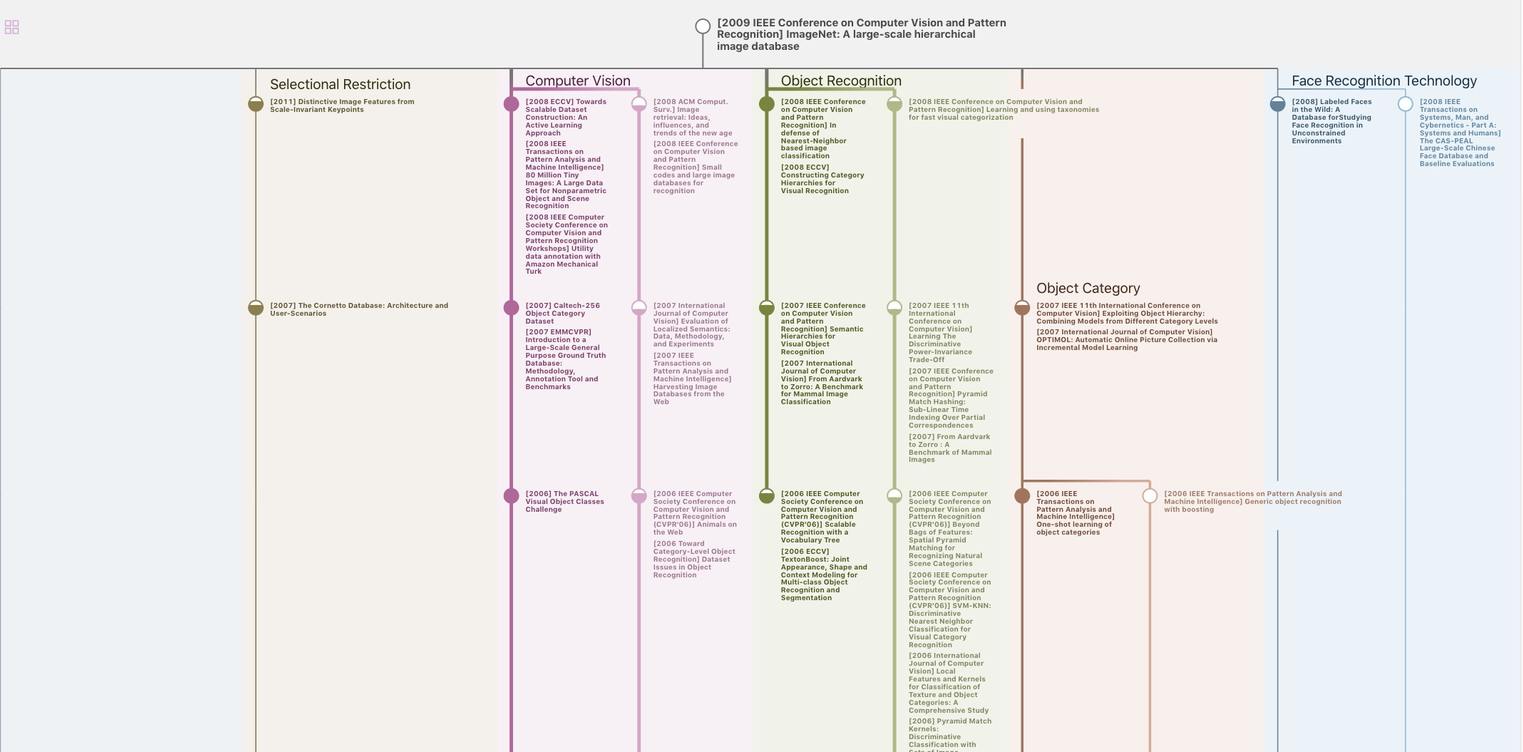
Generate MRT to find the research sequence of this paper
Data Disclaimer
The page data are from open Internet sources, cooperative publishers and automatic analysis results through AI technology. We do not make any commitments and guarantees for the validity, accuracy, correctness, reliability, completeness and timeliness of the page data. If you have any questions, please contact us by email: report@aminer.cn
Chat Paper