基本信息
views: 9664
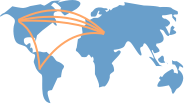
Bio
My primary research interest covers the following areas:
Deep Learning (yes, everyone works on this now). What interests me particularly are algorithms for state updates, invariances and statistical testing.
Scalability of algorithms. This means pushing algorithms to internet scale, distributing them on many (faulty) machines, showing convergence, and modifying models to fit these requirements. For instance, randomized techniques are quite promising in this context. In other words, I'm interested in big data.
Kernels methods are quite an effective means of making linear methods nonlinear and nonparametric. My research interests include support vector Machines, gaussian processes, and conditional random fields. Kernels are very useful also for the representation of distributions, that is two-sample tests, independence tests and many applications to unsupervised learning.
Statistical modeling, primarily with Bayesian Nonparametrics is a great way of addressing many modeling problems. Quite often, the techniques overlap with kernel methods and scalability in rather delightful ways.
Applications, primarily in terms of user modeling, document analysis, temporal models, and modeling data at scale is a great source of inspiration. That is, how can we find principled techniques to solve the problem, what are the underlying concepts, how can we solve things automatically.
Deep Learning (yes, everyone works on this now). What interests me particularly are algorithms for state updates, invariances and statistical testing.
Scalability of algorithms. This means pushing algorithms to internet scale, distributing them on many (faulty) machines, showing convergence, and modifying models to fit these requirements. For instance, randomized techniques are quite promising in this context. In other words, I'm interested in big data.
Kernels methods are quite an effective means of making linear methods nonlinear and nonparametric. My research interests include support vector Machines, gaussian processes, and conditional random fields. Kernels are very useful also for the representation of distributions, that is two-sample tests, independence tests and many applications to unsupervised learning.
Statistical modeling, primarily with Bayesian Nonparametrics is a great way of addressing many modeling problems. Quite often, the techniques overlap with kernel methods and scalability in rather delightful ways.
Applications, primarily in terms of user modeling, document analysis, temporal models, and modeling data at scale is a great source of inspiration. That is, how can we find principled techniques to solve the problem, what are the underlying concepts, how can we solve things automatically.
Research Interests
Papers共 504 篇Author StatisticsCo-AuthorSimilar Experts
By YearBy Citation主题筛选期刊级别筛选合作者筛选合作机构筛选
时间
引用量
主题
期刊级别
合作者
合作机构
ICLR 2023 (2023)
CoRR (2023)
ICLR 2023 (2023)
JOURNAL OF MACHINE LEARNING RESEARCH (2023): 162:1-162:45
Cited11Views0EIBibtex
11
0
ICMLpp.10879-10928, (2023)
CoRR (2023): 4765-4775
Load More
Author Statistics
Co-Author
Co-Institution
D-Core
- 合作者
- 学生
- 导师
Data Disclaimer
The page data are from open Internet sources, cooperative publishers and automatic analysis results through AI technology. We do not make any commitments and guarantees for the validity, accuracy, correctness, reliability, completeness and timeliness of the page data. If you have any questions, please contact us by email: report@aminer.cn