基本信息
浏览量:239
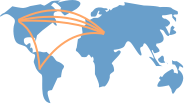
个人简介
Research
My research has three intertwined goals: (i) develop theory and models that capture the fundamental limits of estimation and learning from data, (ii) construct fair and private learning algorithms with performance guarantees based on these limits, and (iii) use this methodology as a design driver for future information processing and content distribution systems. In order to achieve these goals I use theoretical tools from information theory, statistics, cryptography and machine learning.
I consider myself a scientist who is an engineer at heart, so I enjoy doing fundamental research that serves as a design driver for practical applications. I have a broad set of interests which include information theory, statistics, communications and optimization. You can find more details in the publications below.
My research has three intertwined goals: (i) develop theory and models that capture the fundamental limits of estimation and learning from data, (ii) construct fair and private learning algorithms with performance guarantees based on these limits, and (iii) use this methodology as a design driver for future information processing and content distribution systems. In order to achieve these goals I use theoretical tools from information theory, statistics, cryptography and machine learning.
I consider myself a scientist who is an engineer at heart, so I enjoy doing fundamental research that serves as a design driver for practical applications. I have a broad set of interests which include information theory, statistics, communications and optimization. You can find more details in the publications below.
研究兴趣
论文共 124 篇作者统计合作学者相似作者
按年份排序按引用量排序主题筛选期刊级别筛选合作者筛选合作机构筛选
时间
引用量
主题
期刊级别
合作者
合作机构
Jamelle Watson-Daniels,Flavio du Pin Calmon, Alexander D'Amour,Carol Long,David C. Parkes,Berk Ustun
CoRR (2024)
引用0浏览0EI引用
0
0
CoRR (2024)
引用0浏览0EI引用
0
0
ICML 2023pp.508-528, (2023)
引用1浏览0EI引用
1
0
JOURNAL OF MACHINE LEARNING RESEARCHno. 1 (2023): 26:1-26:43
引用0浏览0EI引用
0
0
NeurIPS (2023)
引用1浏览0EI引用
1
0
ISITpp.2195-2200, (2023)
引用1浏览0EIWOS引用
1
0
加载更多
作者统计
合作学者
合作机构
D-Core
- 合作者
- 学生
- 导师
数据免责声明
页面数据均来自互联网公开来源、合作出版商和通过AI技术自动分析结果,我们不对页面数据的有效性、准确性、正确性、可靠性、完整性和及时性做出任何承诺和保证。若有疑问,可以通过电子邮件方式联系我们:report@aminer.cn