基本信息
浏览量:254
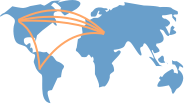
个人简介
Research:
2015-2020: Explaining machine learning decisions
The goal of this project is to develop methods to explain the decisions (e.g. classifications) of machine learning models in terms of input variables (i.e. which input variables are relevant for the model's decision). Techniques that have been developed include the deep Taylor decomposition, that operates by performing a first-order Taylor expansion at each neuron of a deep network. These expansions are then recombined to produce a relevance propagation algorithm, where the model's decision is redistributed from layer to layer until the input is reached.
2012-2013: Machine learning in the chemical compound space
The project consists of using machine learning to dramatically accelerate the calculation of molecular properties (e.g. atomization energy or polarizability) for large collections of molecules. These properties are usually obtained from complex physics simulations, that must be performed for each molecule individually. The machine learning speedup is mainly based on exploiting similarity between molecules, and on automatically extracting a more compact task representation.
2015-2020: Explaining machine learning decisions
The goal of this project is to develop methods to explain the decisions (e.g. classifications) of machine learning models in terms of input variables (i.e. which input variables are relevant for the model's decision). Techniques that have been developed include the deep Taylor decomposition, that operates by performing a first-order Taylor expansion at each neuron of a deep network. These expansions are then recombined to produce a relevance propagation algorithm, where the model's decision is redistributed from layer to layer until the input is reached.
2012-2013: Machine learning in the chemical compound space
The project consists of using machine learning to dramatically accelerate the calculation of molecular properties (e.g. atomization energy or polarizability) for large collections of molecules. These properties are usually obtained from complex physics simulations, that must be performed for each molecule individually. The machine learning speedup is mainly based on exploiting similarity between molecules, and on automatically extracting a more compact task representation.
研究兴趣
论文共 95 篇作者统计合作学者相似作者
按年份排序按引用量排序主题筛选期刊级别筛选合作者筛选合作机构筛选
时间
引用量
主题
期刊级别
合作者
合作机构
Frederick Klauschen, Jonas Dippel,Philipp Keyl,Philipp Jurmeister,Michael Bockmayr,Andreas Mock, Oliver Buchstab,Maximilian Alber,Lukas Ruff,Grégoire Montavon,Klaus-Robert Müller
Pathologie (Heidelberg, Germany)no. 2 (2024): 133-139
Frederick Klauschen, Jonas Dippel, Philipp Keyl,Philipp Jurmeister,Michael Bockmayr,Andreas Mock, Oliver Buchstab,Maximilian Alber,Lukas Ruff,Gregoire Montavon,Klaus-Robert Mueller
ANNUAL REVIEW OF PATHOLOGY-MECHANISMS OF DISEASEno. 1 (2024): 541-570
IEEE transactions on pattern analysis and machine intelligenceno. 99 (2024): 1-18
Pattern Recognition (2024): 110309
Frederick Klauschen, Jonas Dippel,Philipp Keyl,Philipp Jurmeister,Michael Bockmayr,Andreas Mock, Oliver Buchstab,Maximilian Alber,Lukas Ruff,Grégoire Montavon,Klaus-Robert Müller
Die Pathologieno. 2 (2024): 133-139
CoRR (2024)
引用0浏览0EI引用
0
0
INFORMATION FUSION (2024): 102094-102094
加载更多
作者统计
合作学者
合作机构
D-Core
- 合作者
- 学生
- 导师
数据免责声明
页面数据均来自互联网公开来源、合作出版商和通过AI技术自动分析结果,我们不对页面数据的有效性、准确性、正确性、可靠性、完整性和及时性做出任何承诺和保证。若有疑问,可以通过电子邮件方式联系我们:report@aminer.cn