基本信息
浏览量:810
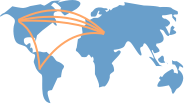
个人简介
Multi-Agent Research
Most of my recent research revolves around multi-agent learning - possibly the most complex AI setting under investigation. Over the past 20 years machine learning has evolved to become the leading paradigm in artificial intelligence. In my work, I explore how we can build more and more intelligent systems or agents that learn from experience.
Multi-Agent learning systems are of fundamental importance for this endeavour four three reasons:
Much of the world’s complexity can be understood in terms of multiple agents interacting - ranging from cells in our bodies to interactions among people to interactions of organisations as large as nation states. Succeeding in such a multi-agent world requires specific skills. Most importantly, artificial agents need to be able to cooperate with other agents - including humans, other artificial agents, and entire organisations. How can we train artificial agents to acquire these social skills?
Many entities that we think of as intelligent agents are composed of subagents. This includes ourselves as being composed of many different cells, but also teams or organisations that are composed of many different people, yet act as as coherent agents. Even the global market economy can be viewed as an agent composed of many firms that interact through supply chains. How can we harness the power of multi-agent architectures for artificial intelligence?
Human intelligence - arguably the highest level of intelligence observed in Nature - did not evolve in isolation but as part of a rich evolving eco-system in an interplay of cooperation and competition at the level of species, tribes and individuals. If we wish to design learning agents that acquire their abilities through interaction and by learning from experience, we not only need to design powerful learning algorithms, but also create rich multi-agent worlds in which those learning agents can interact. What will multi-agent training systems look like that can create automatic learning curricula to foster ever greater intelligence in artificial agents?
Most of my recent research revolves around multi-agent learning - possibly the most complex AI setting under investigation. Over the past 20 years machine learning has evolved to become the leading paradigm in artificial intelligence. In my work, I explore how we can build more and more intelligent systems or agents that learn from experience.
Multi-Agent learning systems are of fundamental importance for this endeavour four three reasons:
Much of the world’s complexity can be understood in terms of multiple agents interacting - ranging from cells in our bodies to interactions among people to interactions of organisations as large as nation states. Succeeding in such a multi-agent world requires specific skills. Most importantly, artificial agents need to be able to cooperate with other agents - including humans, other artificial agents, and entire organisations. How can we train artificial agents to acquire these social skills?
Many entities that we think of as intelligent agents are composed of subagents. This includes ourselves as being composed of many different cells, but also teams or organisations that are composed of many different people, yet act as as coherent agents. Even the global market economy can be viewed as an agent composed of many firms that interact through supply chains. How can we harness the power of multi-agent architectures for artificial intelligence?
Human intelligence - arguably the highest level of intelligence observed in Nature - did not evolve in isolation but as part of a rich evolving eco-system in an interplay of cooperation and competition at the level of species, tribes and individuals. If we wish to design learning agents that acquire their abilities through interaction and by learning from experience, we not only need to design powerful learning algorithms, but also create rich multi-agent worlds in which those learning agents can interact. What will multi-agent training systems look like that can create automatic learning curricula to foster ever greater intelligence in artificial agents?
研究兴趣
论文共 219 篇作者统计合作学者相似作者
按年份排序按引用量排序主题筛选期刊级别筛选合作者筛选合作机构筛选
时间
引用量
主题
期刊级别
合作者
合作机构
International Conference on Learning Representations (ICLR) (2022)
INTERNATIONAL CONFERENCE ON MACHINE LEARNING, VOL 139 (2021): 7480-7491
加载更多
作者统计
合作学者
合作机构
D-Core
- 合作者
- 学生
- 导师
数据免责声明
页面数据均来自互联网公开来源、合作出版商和通过AI技术自动分析结果,我们不对页面数据的有效性、准确性、正确性、可靠性、完整性和及时性做出任何承诺和保证。若有疑问,可以通过电子邮件方式联系我们:report@aminer.cn