A dual coordinate descent method for large-scale linear SVM
ICML(2008)
摘要
In many applications, data appear with a huge number of instances as well as features. Linear Support Vector Machines (SVM) is one of the most popular tools to deal with such large-scale sparse data. This paper presents a novel dual coordinate descent method for linear SVM with L1-and L2-loss functions. The proposed method is simple and reaches an ε-accurate solution in O(log(1/ε)) iterations. Experiments indicate that our method is much faster than state of the art solvers such as Pegasos, TRON, SVMperf, and a recent primal coordinate descent implementation.
更多查看译文
关键词
l1-and l2-loss function,accurate solution,descent implementation,large-scale linear svm,linear svm,huge number,large-scale sparse data,vector machines,descent method,linear support,coordinate descent,sparse data,loss function,support vector machine
AI 理解论文
溯源树
样例
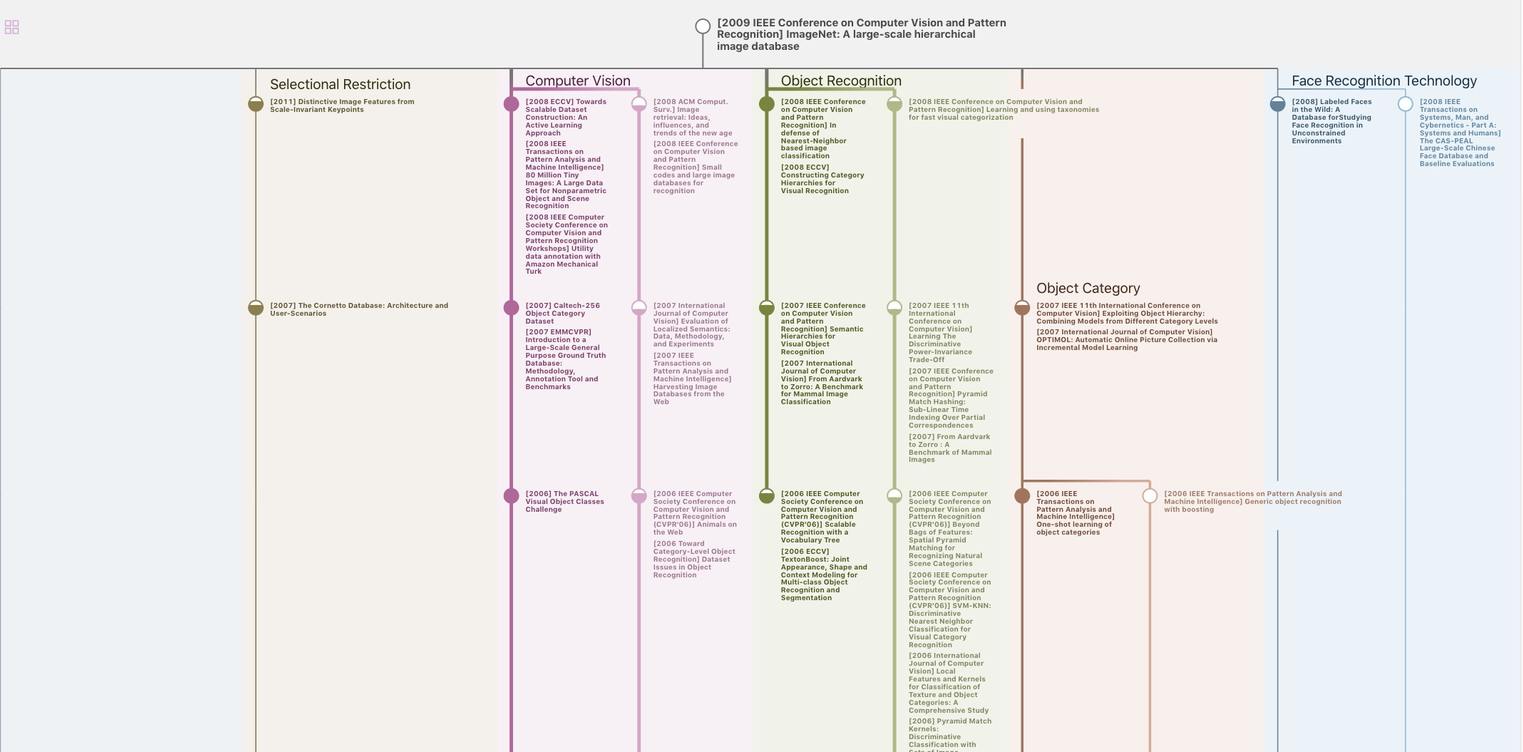
生成溯源树,研究论文发展脉络
Chat Paper
正在生成论文摘要