Online parameter tuning for object tracking algorithms
Image and Vision Computing(2014)
摘要
Object tracking quality usually depends on video scene conditions (e.g. illumination, density of objects, object occlusion level). In order to overcome this limitation, this article presents a new control approach to adapt the object tracking process to the scene condition variations. More precisely, this approach learns how to tune the tracker parameters to cope with the tracking context variations. The tracking context, or context, of a video sequence is defined as a set of six features: density of mobile objects, their occlusion level, their contrast with regard to the surrounding background, their contrast variance, their 2D area and their 2D area variance. In an offline phase, training video sequences are classified by clustering their contextual features. Each context cluster is then associated to satisfactory tracking parameters. In the online control phase, once a context change is detected, the tracking parameters are tuned using the learned values. The approach has been experimented with three different tracking algorithms and on long, complex video datasets. This article brings two significant contributions: (1) a classification method of video sequences to learn offline tracking parameters and (2) a new method to tune online tracking parameters using tracking context.
更多查看译文
关键词
object tracking process,online parameter,online tracking parameter,tracking context variation,occlusion level,tracking context,different tracking algorithm,offline tracking parameter,tracking parameter,satisfactory tracking parameter,video sequence,machine learning,controller,object tracking
AI 理解论文
溯源树
样例
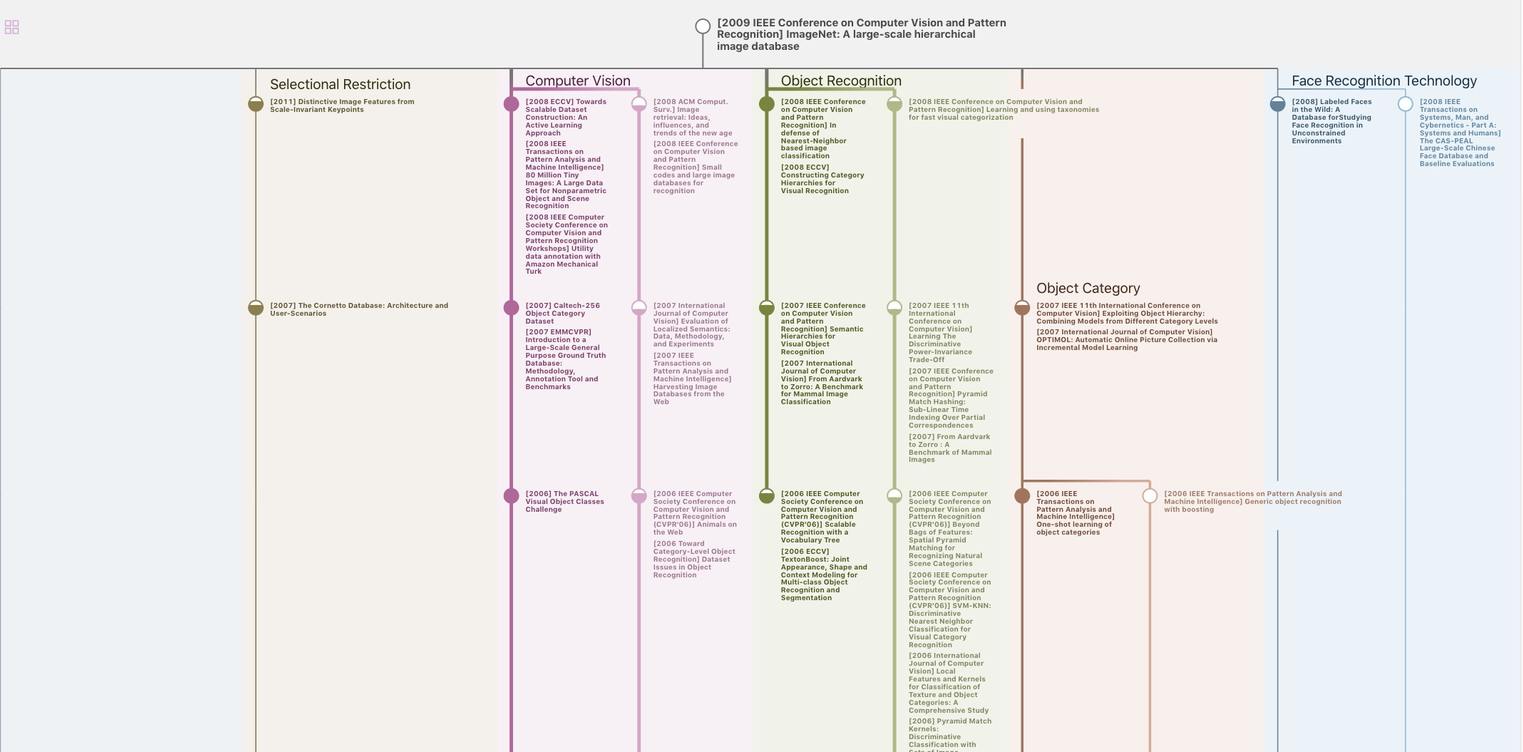
生成溯源树,研究论文发展脉络
Chat Paper
正在生成论文摘要