Kernel Topic Models
AISTATS(2012)
摘要
Latent Dirichlet Allocation models discrete data as a mixture of discrete distributions, using Dirichlet beliefs over the mixture weights. We study a variation of this concept, in which the documents' mixture weight beliefs are replaced with squashed Gaussian distributions. This allows documents to be associated with elements of a Hilbert space, admitting kernel topic models (KTM), modelling temporal, spatial, hierarchical, social and other structure between documents. The main challenge is efficient approximate inference on the latent Gaussian. We present an approximate algorithm cast around a Laplace approximation in a transformed basis. The KTM can also be interpreted as a type of Gaussian process latent variable model, or as a topic model conditional on document features, uncovering links between earlier work in these areas.
更多查看译文
关键词
information retrieval,laplace approximation,gaussian distribution,latent dirichlet allocation,gaussian process,hilbert space,discrete distribution,machine learning,latent variable model
AI 理解论文
溯源树
样例
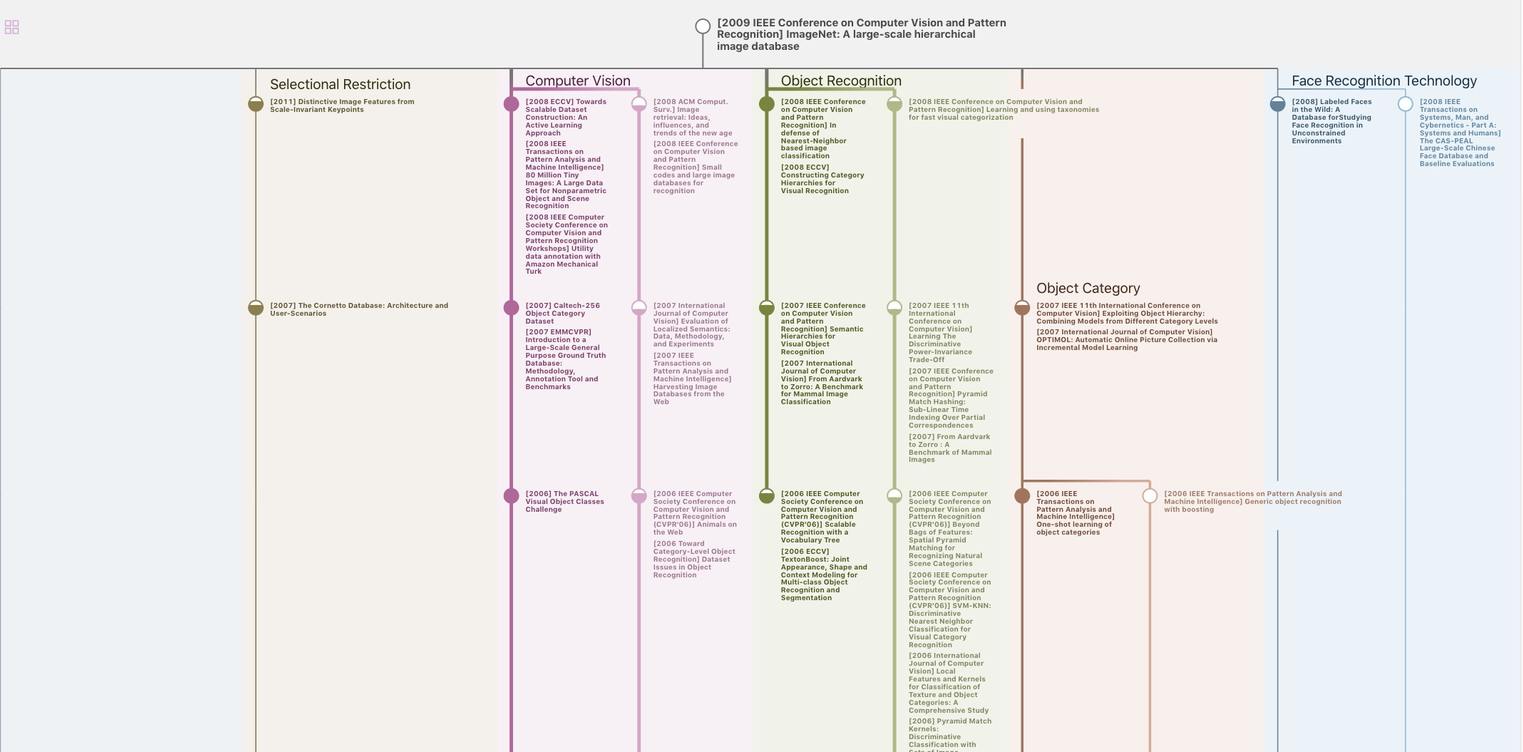
生成溯源树,研究论文发展脉络
Chat Paper
正在生成论文摘要