Mixtures of inverse covariances
IEEE Transactions on Speech and Audio Processing(2004)
摘要
We describe a model which approximates full covariances in a Gaussian mixture while reducing significantly both the number of parameters to estimate and the computations required to evaluate the Gaussian likelihoods. In this model, the inverse covariance of each Gaussian in the mixture is expressed as a linear combination of a small set of prototype matrices that are shared across components. In addition, we demonstrate the benefits of a subspace-factored extension of this model when representing independent or near-independent product densities. We present a maximum likelihood estimation algorithm for these models, as well as a practical method for implementing it. We show through experiments performed on a variety of speech recognition tasks that this model significantly outperforms a diagonal covariance model, while using far fewer Gaussian-specific parameters. Experiments also demonstrate that a better speed/accuracy tradeoff can be achieved on a real-time speech recognition system.
更多查看译文
关键词
gaussian mixture model,maximum-likelihood estimation algorithm,parameters estimation,subspace-factored extension,diagonal covariance model,gaussian processes,acoustic modeling,speech recognition,inverse covariances,prototype matrices,covariance analysis,parameter estimation,block-diagonal covariance,maximum likelihood estimation,mixture weights estimation,covariance matrices,inverse covariances mixture,full covariance,acoustic signal processing,gaussian likelihoods,matrix inversion,gaussian-dependent parameters,automatic speech recognition,mle,distributed computing,prototypes,vectors,covariance matrix,speech processing,maximum likelihood estimate
AI 理解论文
溯源树
样例
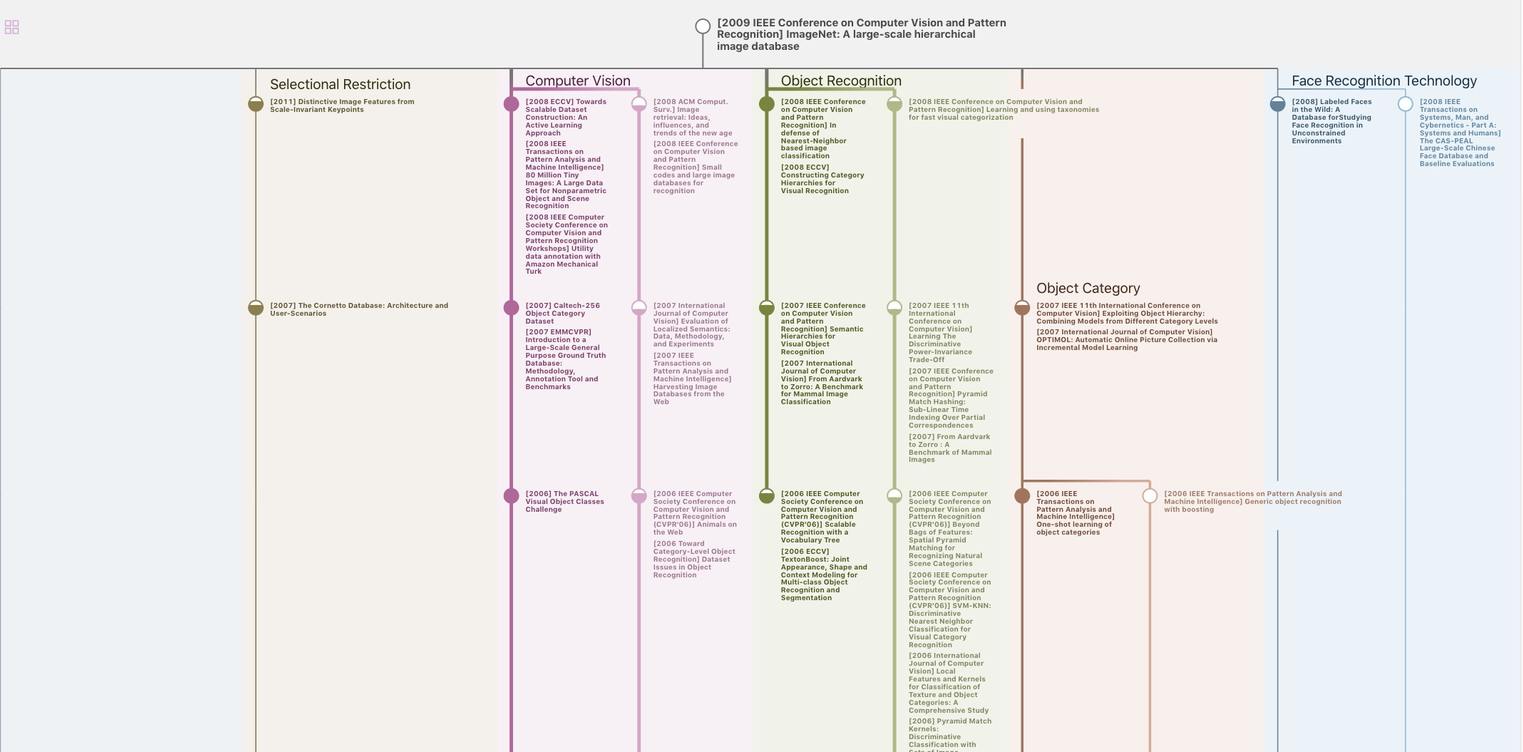
生成溯源树,研究论文发展脉络
Chat Paper
正在生成论文摘要