Efficient Online Subspace Learning With an Indefinite Kernel for Visual Tracking and Recognition.
IEEE Transactions on Neural Networks and Learning Systems(2012)
摘要
We propose an exact framework for online learning with a family of indefinite (not positive) kernels. As we study the case of nonpositive kernels, we first show how to extend kernel principal component analysis (KPCA) from a reproducing kernel Hilbert space to Krein space. We then formulate an incremental KPCA in Krein space that does not require the calculation of preimages and therefore is both ...
更多查看译文
关键词
Kernel,Hilbert space,Visualization,Robustness,Eigenvalues and eigenfunctions,Principal component analysis,Vectors
AI 理解论文
溯源树
样例
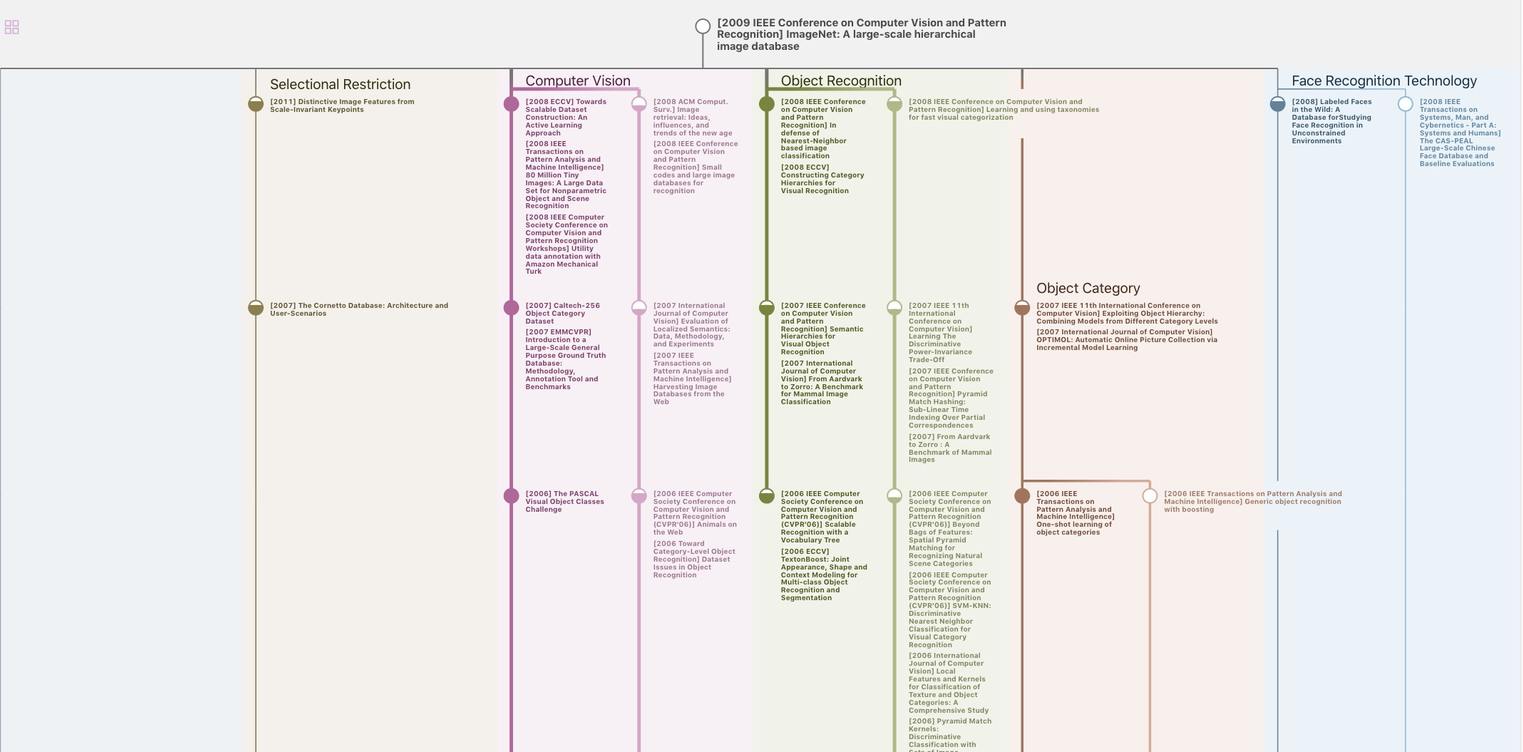
生成溯源树,研究论文发展脉络
Chat Paper
正在生成论文摘要