Identifying Relevant Data for a Biological Database: Handcrafted Rules versus Machine Learning
IEEE/ACM Trans. Comput. Biology Bioinform.(2011)
摘要
With well over 1,000 specialized biological databases in use today, the task of automatically identifying novel, relevant data for such databases is increasingly important. In this paper, we describe practical machine learning approaches for identifying MEDLINE documents and Swiss-Prot/TrEMBL protein records, for incorporation into a specialized biological database of transport proteins named TCDB. We show that both learning approaches outperform rules created by hand by a human expert. As one of the first case studies involving two different approaches to updating a deployed database, both the methods compared and the results will be of interest to curators of many specialized databases.
更多查看译文
关键词
data mining,text mining,association rules,information retrieval,proteins,biological databases,data analysis,learning artificial intelligence,biological database,indexing terms,protein sequence,databases,molecular biophysics,clustering,bioinformatics,association rule,machine learning,classification,computer science,genomics
AI 理解论文
溯源树
样例
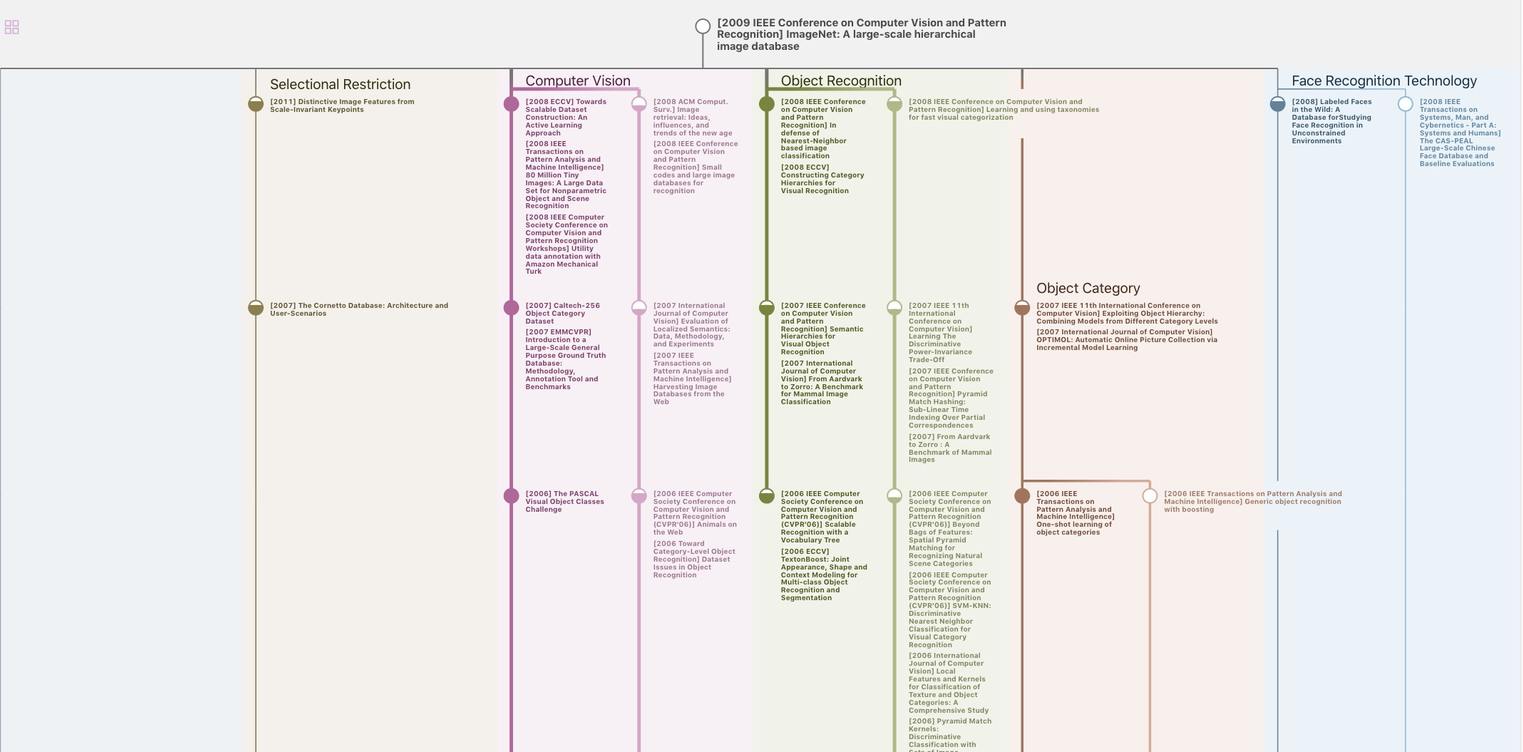
生成溯源树,研究论文发展脉络
Chat Paper
正在生成论文摘要