Data-Driven Theory Refinement Algorithms for Bioinformatics
IJCNN(1999)
摘要
Bioinformatics and related applications call for efficient algorithms for knowledgeintensive learning and data-driven knowledge refinement. Knowledge based arti- tending or modifying incomplete knowledge bases or do- main theories. we present results of experiments with sev- eral such algorithms for data-driven knowledge discovery and theory refinement in some simple bioinformatics appli- cations. Results of experiments on the ribosome binding site and promoter site identification problems indicate that the performance of KBDistAl and Tiling-Pyramid algorithms com- pares quite favorably with those of substantially more com- putationally demanding techniques. either augmenting it with new knowledge or by refining the &sting knowledge are called theory refinement systems. the following categories. ficial neural offer an attractive approach to ex- Theory refinement systems be , A~~~~~&~~ based on Rule Induction which use decision tree or rule learning algorithms for theory re vision. Examples of such systems include RTLS (6), EITHER (7), PTR (8), and TGCl (9). A~~~~~&~~ based on Inductive ~~~i~ program-
更多查看译文
关键词
computational complexity,computational biology,data mining,computer science,artificial intelligence,neural nets,neural networks,bioinformatics,artificial neural network,knowledge base,network topology,artificial neural networks,ribosome binding site,domain theory,knowledge based systems
AI 理解论文
溯源树
样例
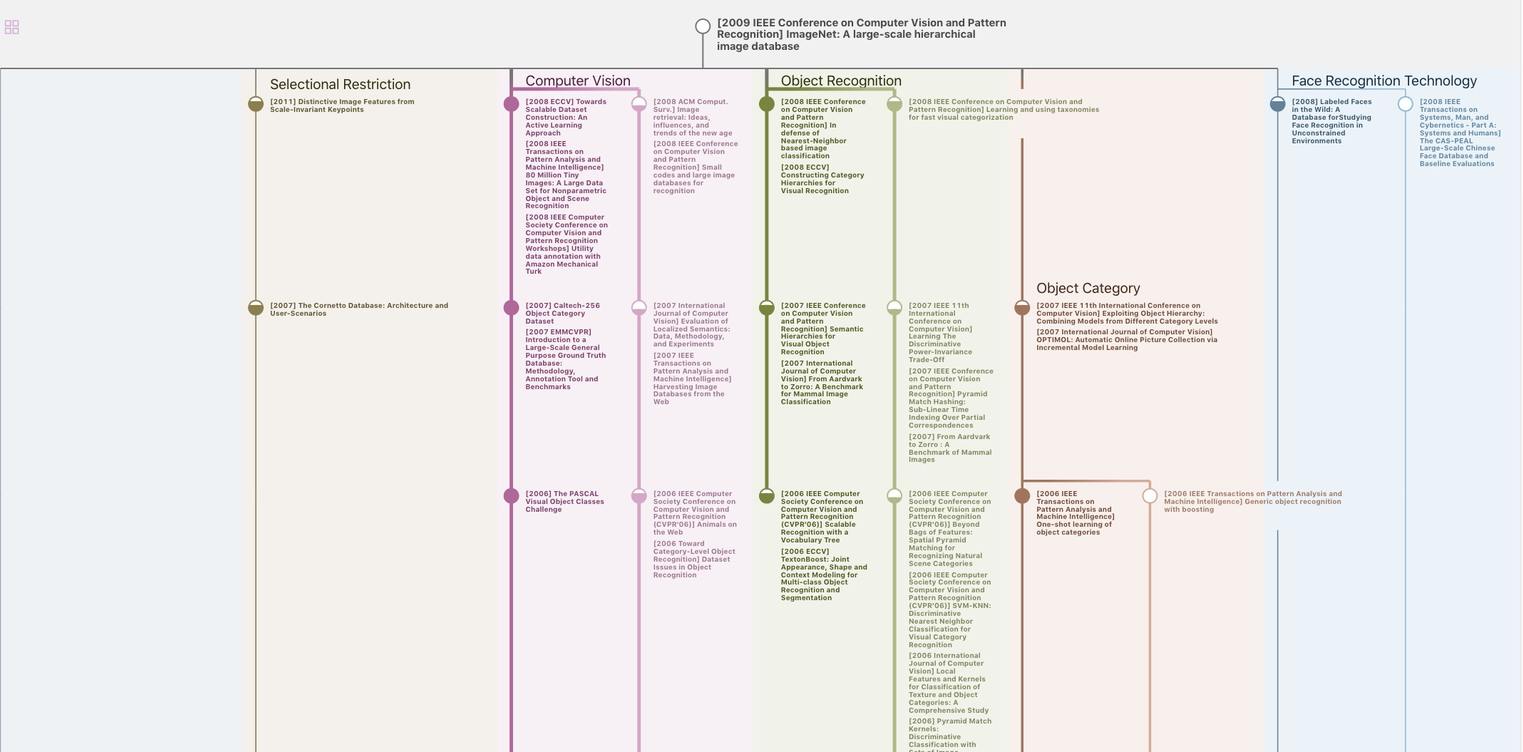
生成溯源树,研究论文发展脉络
Chat Paper
正在生成论文摘要