Enhanced email spam filtering through combining similarity graphs.
WSDM(2011)
摘要
ABSTRACTOver the last decade Email Spam has evolved from being just an irritant to users to being truly dangerous. This has led web-mail providers and academic researchers to dedicate considerable resources towards tackling this problem [9, 21, 22, 24, 26]. However, we argue that some aspects of the spam filtering problem are not handled appropriately in existing work. Principal among these are adversarial spammer efforts -- spammers routinely tune their spam emails to bypass spam-filters, and contaminate ground truth via fake HAM/SPAM votes -- and the scale and sparsity of the problem, which essentially precludes learning with a very large set of parameters. In this paper we propose an approach that learns to filter spam by striking a balance between generalizing HAM/SPAM votes across users and emails (to alleviate sparsity) and learning local models for each user (to limit effect of adversarial votes); votes are shared only amongst users and emails that are "similar" to one another. Moreover, we define user-user and email-email similarities using spam-resilient features that are extremely difficult for spammers to fake. We give a methodology that learns to combine multiple features into similarity values while directly optimizing the objective of better spam filtering. A useful side effect of this methodology is that the number of parameters that need to be estimated is very small: this helps us use off-the-shelf learning algorithms to achieve good accuracy while preventing over-training to the adversarial noise in the data. Finally, our approach gives a systematic way to incorporate existing spam-fighting technologies such as IP blacklists, keyword based classifiers, etc into one framework. Experiments on a real-world email dataset show that our approach leads to significant improvements compared to two state-of-the-art baselines.
更多查看译文
AI 理解论文
溯源树
样例
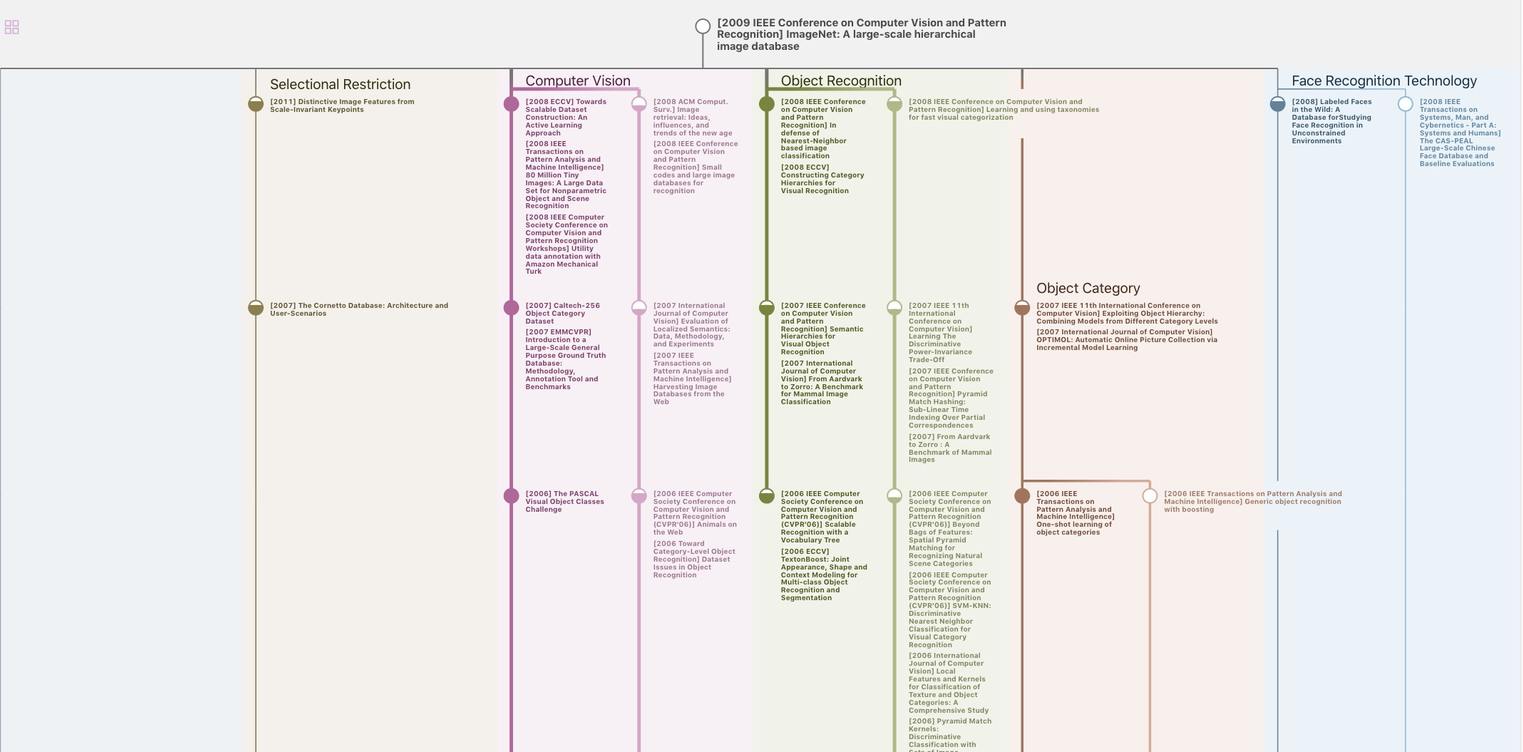
生成溯源树,研究论文发展脉络
Chat Paper
正在生成论文摘要