Semantic mapping and navigation: A Bayesian approach
IROS(2013)
摘要
We propose Bayesian approaches for semantic mapping, active localization and local navigation with affordable vision sensors. We develop Bayesian model of egocentric semantic map which consists of spatial object relationships and spatial node relationships. Our topological-semantic-metric (TSM) map has characteristic that a node is one of the components of a general topological map that contains information about spatial relationships. In localization part, view dependent place recognition, reorientation and active search are used for robot localization. A robot estimates its location by Bayesian filtering which leverages spatial relationships among observed objects. Then a robot can infer the head direction to reach a goal in the semantic map. In navigation part, a robot perceives navigable space with Kinect sensor and then moves to goal location while preserving reference head direction. If obstacles are founded in front, then a robot changes the head direction to avoid them. After avoiding obstacles, a robot performs active localization and finds new head direction to goal location. Our Bayesian navigation program provides how a robot should select either an action for following line of moving direction or action for avoiding obstacles. We show that a mobile robot successfully navigates from starting position to goal node while avoiding obstacles by our proposed semantic navigation system with TSM map.
更多查看译文
关键词
spatial node relationship,goal node,bayesian navigation program,view-dependent place recognition,head direction,bayes methods,egocentric semantic mapping,active localization,robot localization,mobile robots,reorientation,topological-semantic-metric map,active search,bayesian filtering,tsm map,obstacle avoidance,filtering theory,kinect sensor,mobile robot,semantic navigation system,location estimation,vision sensors,bayesian approach,starting position,collision avoidance,local navigation,spatial object relationship
AI 理解论文
溯源树
样例
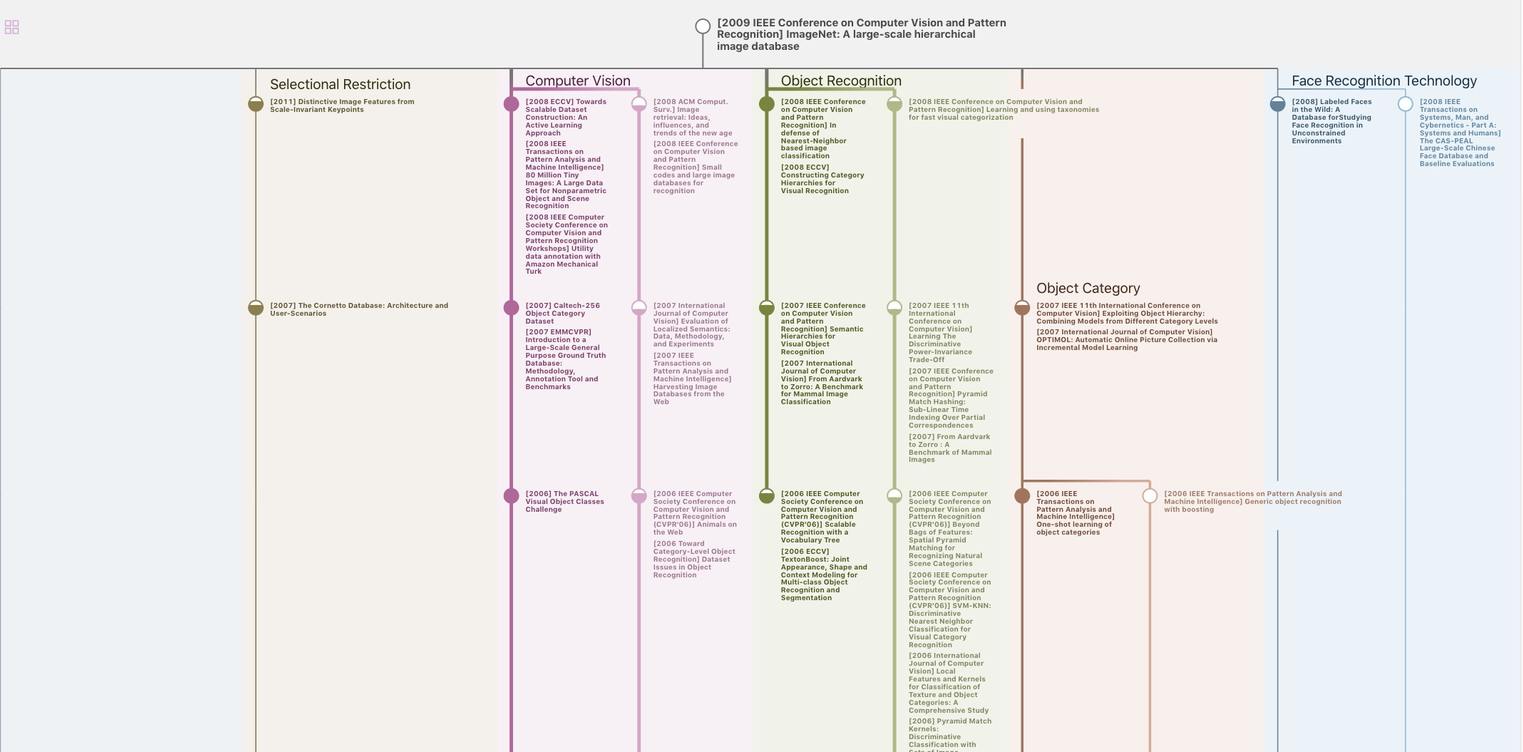
生成溯源树,研究论文发展脉络
Chat Paper
正在生成论文摘要