Computer vision distance measurement from endoscopic sequences: prospective evaluation in laparoscopic ventral hernia repair
Surgical endoscopy(2014)
摘要
Background Research in computer vision and mobile robotics has developed a family of popular algorithms known as Visual Simultaneous Localization And Mapping (Visual SLAM). These algorithms can provide 3D models of body cavities using the images obtained from standard monocular endoscopes. The 3D models can be used to estimate hernia defect measurements during laparoscopic ventral hernia repair (LVHR). Methods We conducted a descriptive and comparative prospective study to analyze results from 15 patients who underwent LVHR. Three methods of measurement were used in each patient: two classical methods (needle and tape) and a new visual SLAM measurement (VSM) method. The major and minor axes of the ellipse-shaped hernia defect were measured. Results Both axes could be measured using the VSM method in all patients except one (93 %). The tape method measured 63 % of the axes, but was difficult to perform because of patient comorbidities and because of limited range of motion of the laparoscopic tools. The needle method obtained 73 % of measurements, because of patient comorbidities. The tape method was the most accurate (accuracy up to 0.5 cm because of tape resolution). The needle method was relatively inaccurate, with a mean error of >3 cm. The VSM method was as accurate as the tape method. The mean time taken to perform measurements was 40 s for the VSM method (range 29–60 s), 169 s for the needle method (range 66–300 s), and 186 s for the tape method (range 110–322 s). Conclusions The needle method is relatively inaccurate and invasive. The tape method is accurate, but is not easy to perform and is relatively time consuming. The VSM method is noninvasive and fast and is as accurate as the tape method.
更多查看译文
关键词
LVHR,Computer vision,Visual SLAM,Hernia defect measurement
AI 理解论文
溯源树
样例
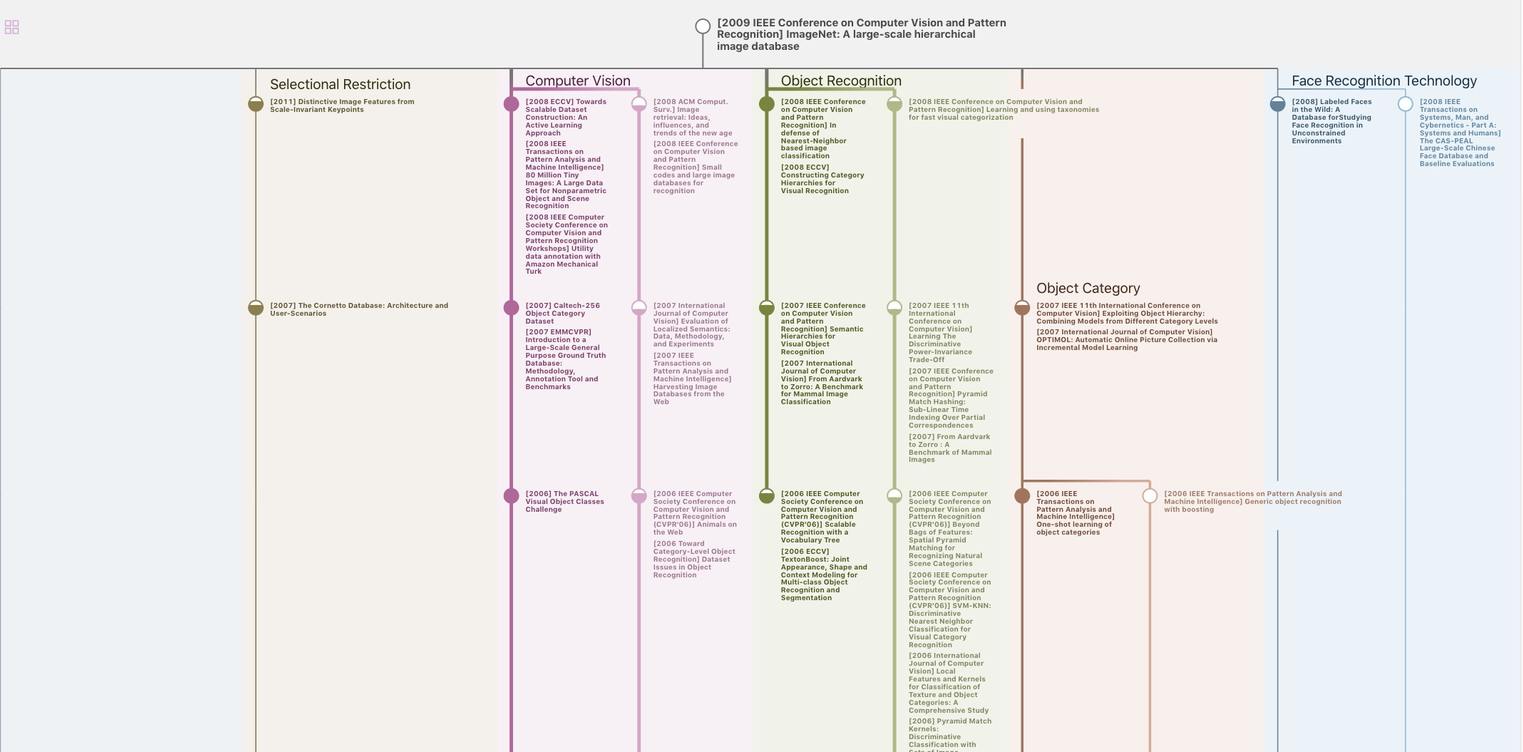
生成溯源树,研究论文发展脉络
Chat Paper
正在生成论文摘要