Deep Learning for Acoustic Modeling in Parametric Speech Generation: A systematic review of existing techniques and future trends
IEEE Signal Process. Mag.(2015)
摘要
Hidden Markov models (HMMs) and Gaussian mixture models (GMMs) are the two most common types of acoustic models used in statistical parametric approaches for generating low-level speech waveforms from high-level symbolic inputs via intermediate acoustic feature sequences. However, these models have their limitations in representing complex, nonlinear relationships between the speech generation inputs and the acoustic features. Inspired by the intrinsically hierarchical process of human speech production and by the successful application of deep neural networks (DNNs) to automatic speech recognition (ASR), deep learning techniques have also been applied successfully to speech generation, as reported in recent literature. This article systematically reviews these emerging speech generation approaches, with the dual goal of helping readers gain a better understanding of the existing techniques as well as stimulating new work in the burgeoning area of deep learning for parametric speech generation.
更多查看译文
关键词
deep neural networks,low-level speech waveforms,gmm,acoustic modeling,human speech production,speech recognition,acoustic features,burgeoning area,acoustic models,hmm,mixture models,parametric speech generation,high-level symbolic inputs,acoustic signal processing,deep learning,asr,gaussian processes,gaussian mixture models,dnn,statistical parametric approach,hidden markov models,neural nets,intermediate acoustic feature sequences,automatic speech recognition,speech processing,speech synthesis
AI 理解论文
溯源树
样例
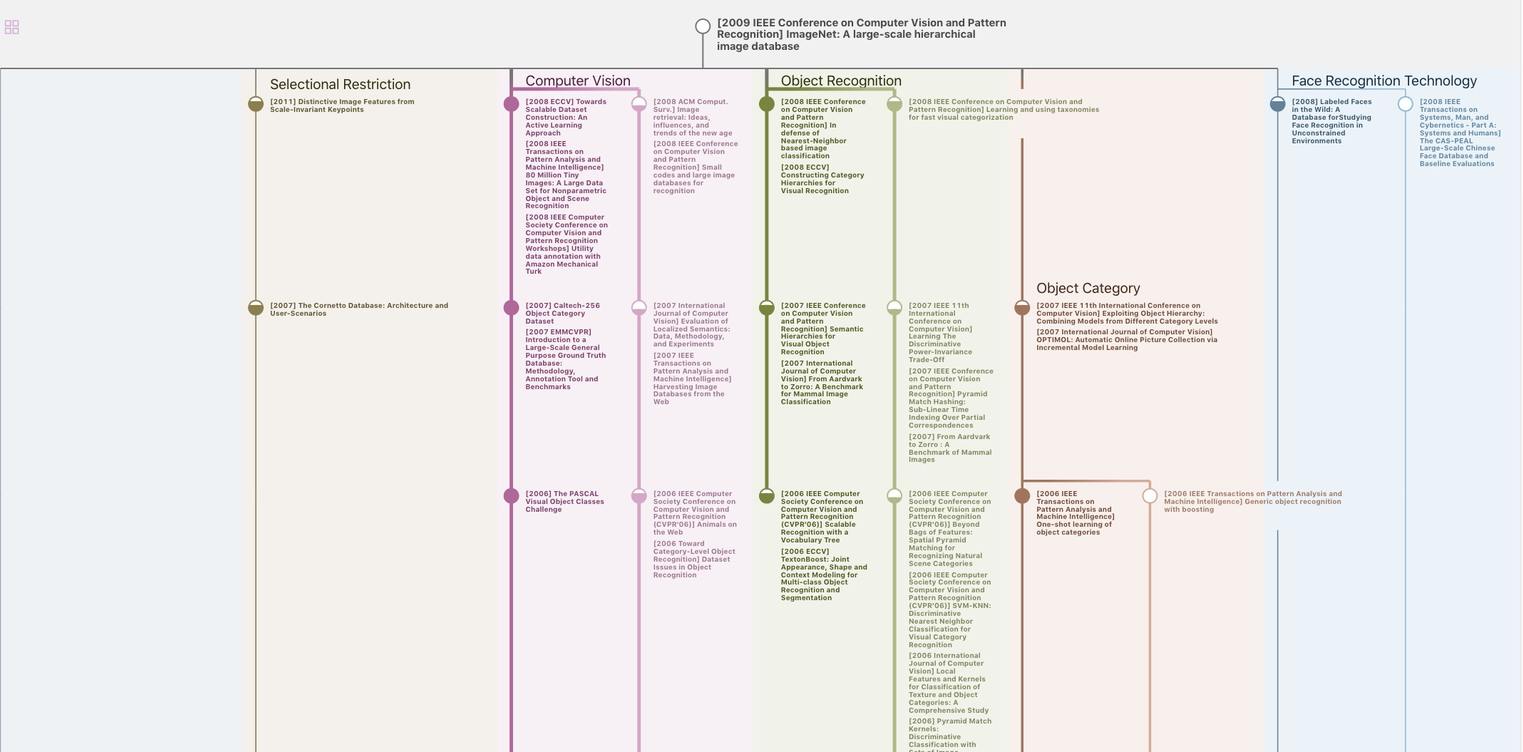
生成溯源树,研究论文发展脉络
Chat Paper
正在生成论文摘要