Improved Testing Of Low Rank Matrices
KDD '14: The 20th ACM SIGKDD International Conference on Knowledge Discovery and Data Mining New York New York USA August, 2014(2014)
摘要
We study the problem of determining if an input matrix A is an element of R(mxn )can be well-approximated by a low rank matrix. Specifically, we study the problem of quickly estimating the rank or stable rank of A, the latter often providing a more robust measure of the rank. Since we seek significantly sublinear time algorithms, we cast these problems in the property testing framework. In this framework, A either has low rank or stable rank, or is far from having this property. The algorithm should read only a small number of entries or rows of A and decide which case A is in with high probability. If neither case occurs, the output is allowed to be arbitrary. We consider two notions of being far: (1) A requires changing at least an epsilon-fraction of its entries, or (2) A requires changing at least an epsilon-fraction of its rows. We call the former the "entry model" and the latter the "row model". We show:For testing if a matrix has rank at most d in the entry model, we improve the previous number of entries of A that need to be read from O(d(2)/epsilon(2)) (Krauthgamer and Sasson, SODA 2003) to O(d(2)/epsilon). Our algorithm is the first to adaptively query the entries of A, which for constant d we show is necessary to achieve O(1/epsilon) queries. For the important case of d = 1 we also give a new non-adaptive algorithm, improving the previous O(1/epsilon(2)) queries to O(log(2)(1/epsilon)/epsilon).For testing if a matrix has rank at most d in the row model, we prove an Omega(d/epsilon) lower bound on the number of rows that need to be read, even for adaptive algorithms. Our lower bound matches a non-adaptive upper bound of Krauthgamer and Sasson.For testing if a matrix has stable rank at most d in the row model or requires changing an epsilon/d-fraction of its rows in order to have stable rank at most d, we prove that reading (Theta) over tilde (d/epsilon(2)) rows is necessary and sufficient.
更多查看译文
关键词
dimensionality reduction,principal component analysis,property testing,robustness,stable rank
AI 理解论文
溯源树
样例
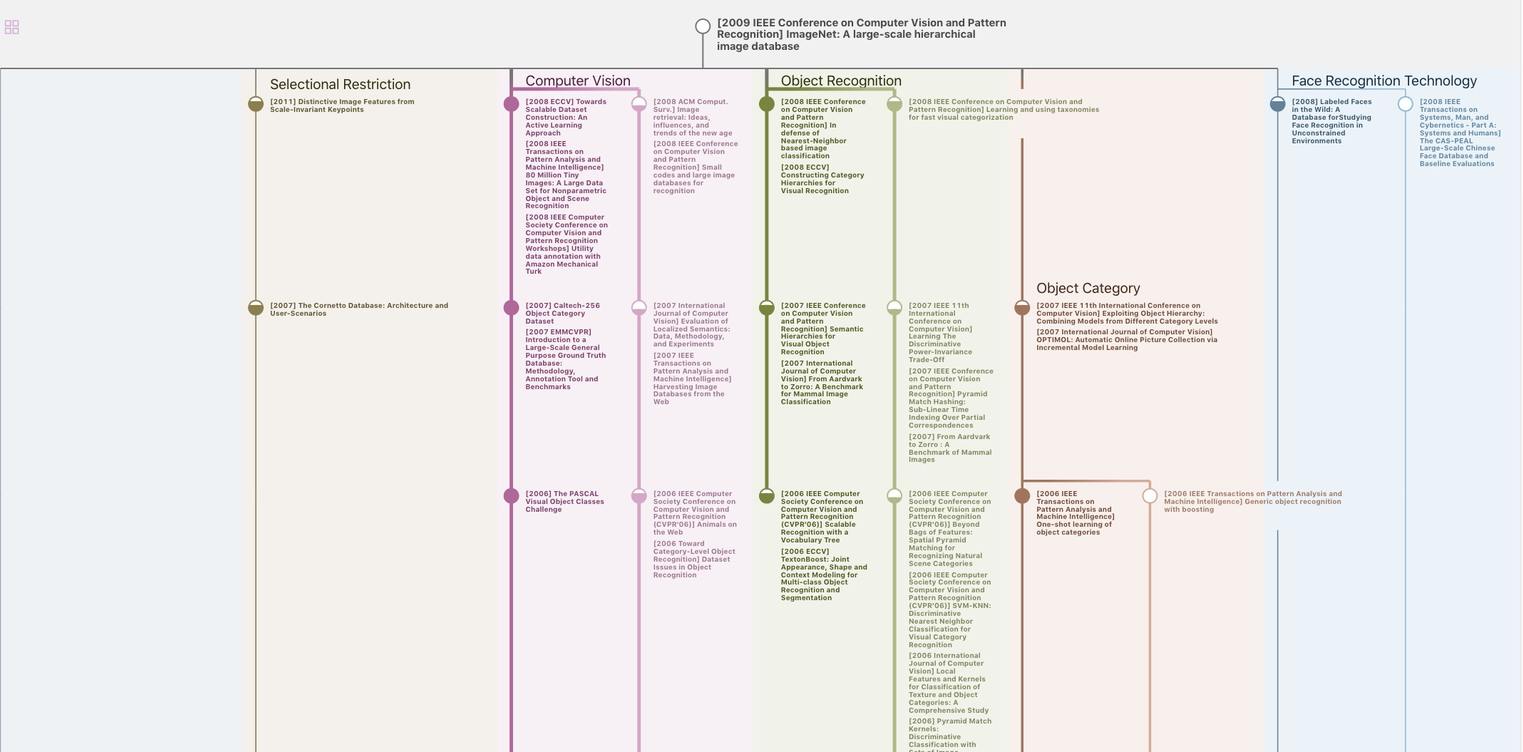
生成溯源树,研究论文发展脉络
Chat Paper
正在生成论文摘要