Locating Self-Collection Points for Last-Mile Logistics Using Public Transport Data.
ADVANCES IN KNOWLEDGE DISCOVERY AND DATA MINING, PART I(2015)
摘要
Delivery failure and re-scheduling cause the delay of services and increase the operation costs for logistics companies. Setting up self-collection points is an effective solution that is attracting attentions from many companies. One challenge for this model is how to choose the locations for self-collection points. In this work, we design a methodology for locating self-collection points. We consider both the distribution of a company's potential customers and the people's gathering pattern in the city. We leverage on citizens' public transport riding records to simulate how the crowds emerge for particular hours. We reasonably assume that a place near to a people crowd is more convenient for customers than a place far away for self parcel collection. Based on this, we propose a kernel transformation method to re-evaluate the pairwise positions of customers, and then do a clustering.
更多查看译文
关键词
Kernel Function, Public Transport, Gaussian Mixture Model, Gaussian Component, Facility Location Problem
AI 理解论文
溯源树
样例
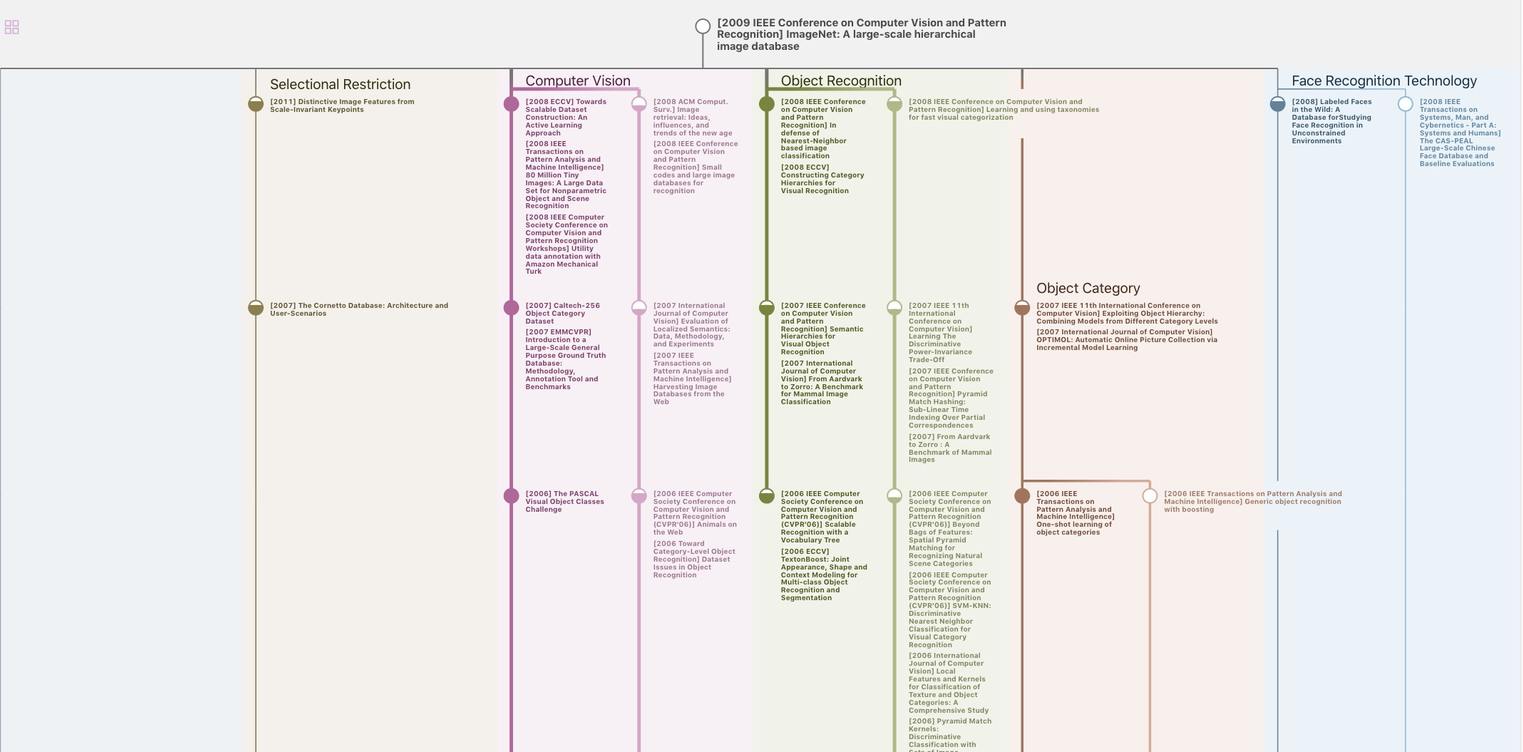
生成溯源树,研究论文发展脉络
Chat Paper
正在生成论文摘要