Learn to wipe: A case study of structural bootstrapping from sensorimotor experience
Robotics and Automation(2014)
摘要
In this paper, we address the question of generative knowledge construction from sensorimotor experience, which is acquired by exploration. We show how actions and their effects on objects, together with perceptual representations of the objects, are used to build generative models which then can be used in internal simulation to predict the outcome of actions. Specifically, the paper presents an experiential cycle for learning association between object properties (softness and height) and action parameters for the wiping task and building generative models from sensorimotor experience resulting from wiping experiments. Object and action are linked to the observed effect to generate training data for learning a non-parametric continuous model using Support Vector Regression. In subsequent iterations, this model is grounded and used to make predictions on the expected effects for novel objects which can be used to constrain the parameter exploration. The cycle and skills have been implemented on the humanoid platform ARMAR-IIIb. Experiments with set of wiping objects differing in softness and height demonstrate efficient learning and adaptation behavior of action of wiping.
更多查看译文
关键词
manipulators,regression analysis,support vector machines,ARMAR-IIIb,generative knowledge construction,learning association,parameter exploration,perceptual representations,sensorimotor experience,structural bootstrapping,support vector regression
AI 理解论文
溯源树
样例
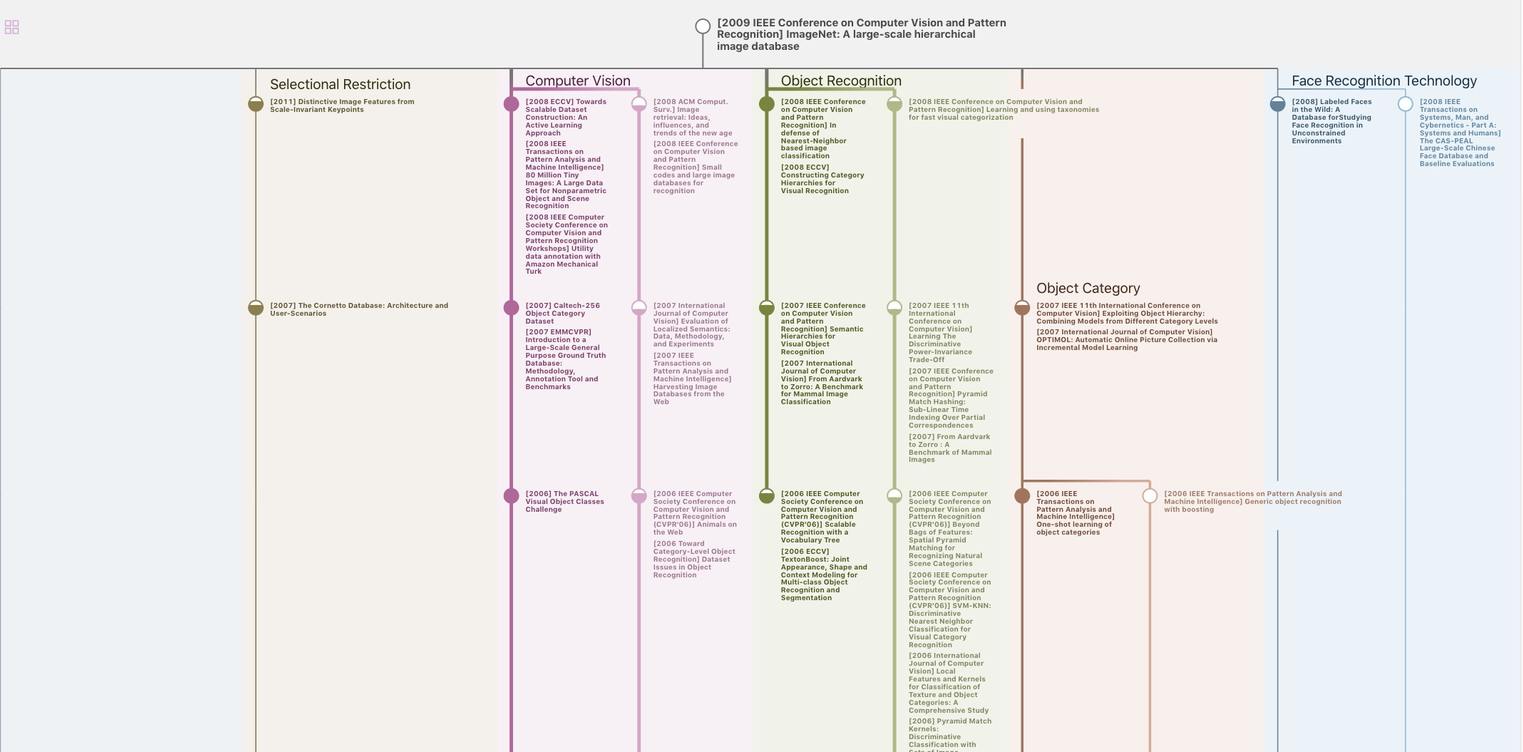
生成溯源树,研究论文发展脉络
Chat Paper
正在生成论文摘要