Multi-robot active SLAM with relative entropy optimization
American Control Conference(2013)
摘要
This paper presents a new approach for Active Simultaneous Localization and Mapping that uses the Relative Entropy(RE) optimization method to select trajectories which minimize both the localization error and the corresponding uncertainty bounds. To that end we construct a planning cost function which includes, besides the state and control cost, a term that encapsulates the uncertainty of the state. This term is the trace of the state covariance matrix produced by the estimator, in this case an Extended Kalman Filter. The role of the RE method is to iteratively guide the selection of the trajectories towards the ones minimizing the aforementioned cost. Once the method has converged, the result is a near-optimal path in terms of achieving the pre-defined goal in the state space while also improving the localization error and the total uncertainty. In essence the method integrates motion planning with robot localization. To evaluate the approach we consider scenarios with single and multiple robots navigating in presence of obstacles and various conditions of landmark densities. The results show a behavior consistent with our expectations.
更多查看译文
关键词
Kalman filters,SLAM (robots),covariance matrices,multi-robot systems,nonlinear filters,optimisation,path planning,RE optimization method,active simultaneous localization and mapping,behavior consistent,extended Kalman Filter,landmark densities,localization error,motion planning,multirobot active SLAM,relative entropy optimization,robot localization,state covariance matrix
AI 理解论文
溯源树
样例
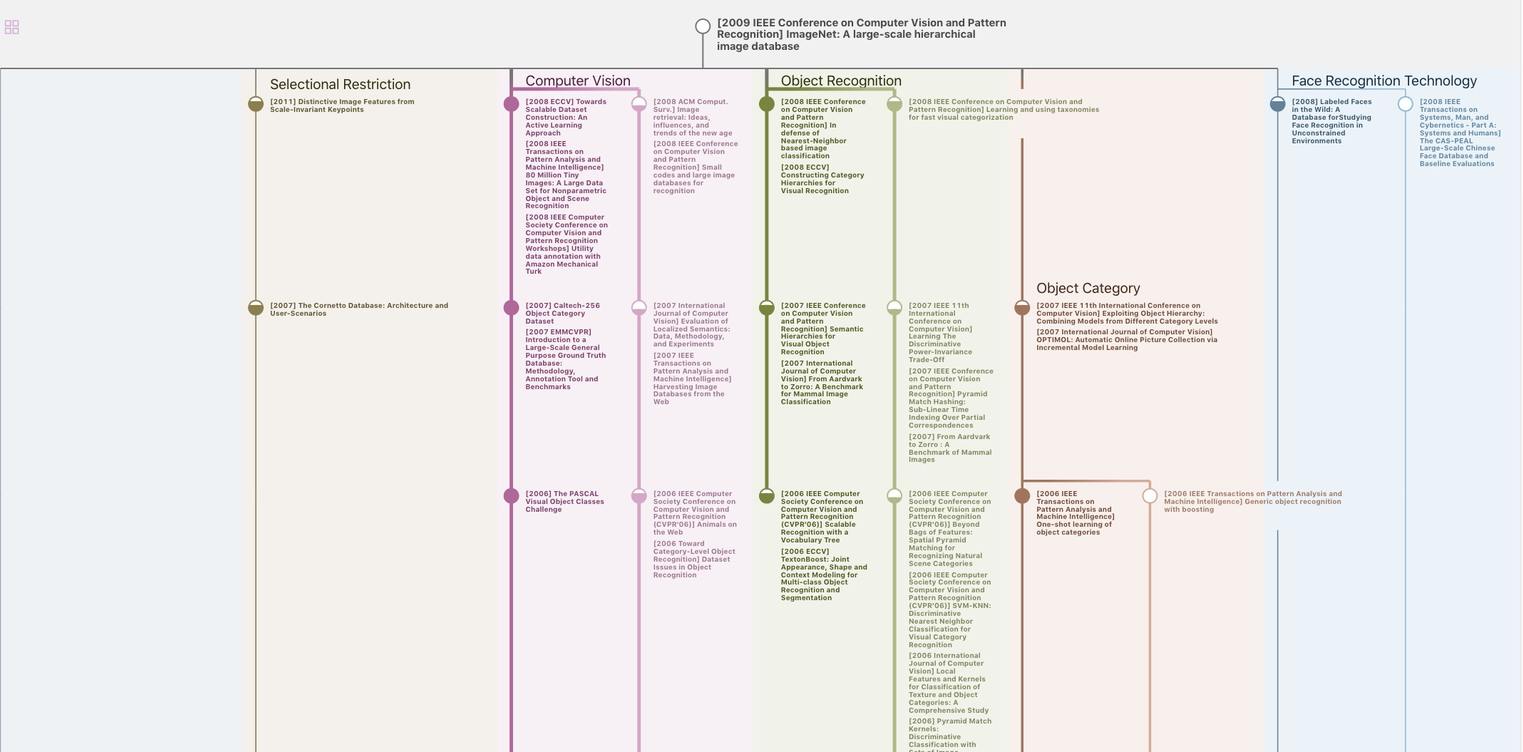
生成溯源树,研究论文发展脉络
Chat Paper
正在生成论文摘要