Learning Compact Hash Codes for Multimodal Representations using Orthogonal Deep Structure
Multimedia, IEEE Transactions(2015)
摘要
As large-scale multimodal data are ubiquitous in many real-world applications, learning multimodal representations for efficient retrieval is a fundamental problem. Most existing methods adopt shallow structures to perform multimodal representation learning. Due to a limitation of learning ability of shallow structures, they fail to capture the correlation of multiple modalities. Recently, multimodal deep learning was proposed and had proven its superiority in representing multimodal data due to its high nonlinearity. However, in order to learn compact and accurate representations, how to reduce the redundant information lying in the multimodal representations and incorporate different complexities of different modalities in the deep models is still an open problem. In order to address the aforementioned problem, we propose a hashing-based orthogonal deep model to learn accurate and compact multimodal representations in this paper. The method can better capture the intra-modality and inter-modality correlations to learn accurate representations. Meanwhile, in order to make the representations compact, the hashing-based model can generate compact hash codes and the proposed orthogonal structure can reduce the redundant information lying in the codes by imposing orthogonal regularizer on the weighting matrices. We also theoretically prove that in this case the learned codes are guaranteed to be approximately orthogonal. Moreover, considering the different characteristics of different modalities, effective representations can be attained with different number of layers for different modalities. Comprehensive experiments on three real-world datasets demonstrate a substantial gain of our method on retrieval tasks compared with existing algorithms.
更多查看译文
关键词
Deep learning,Multimodal hashing,Similarity Search
AI 理解论文
溯源树
样例
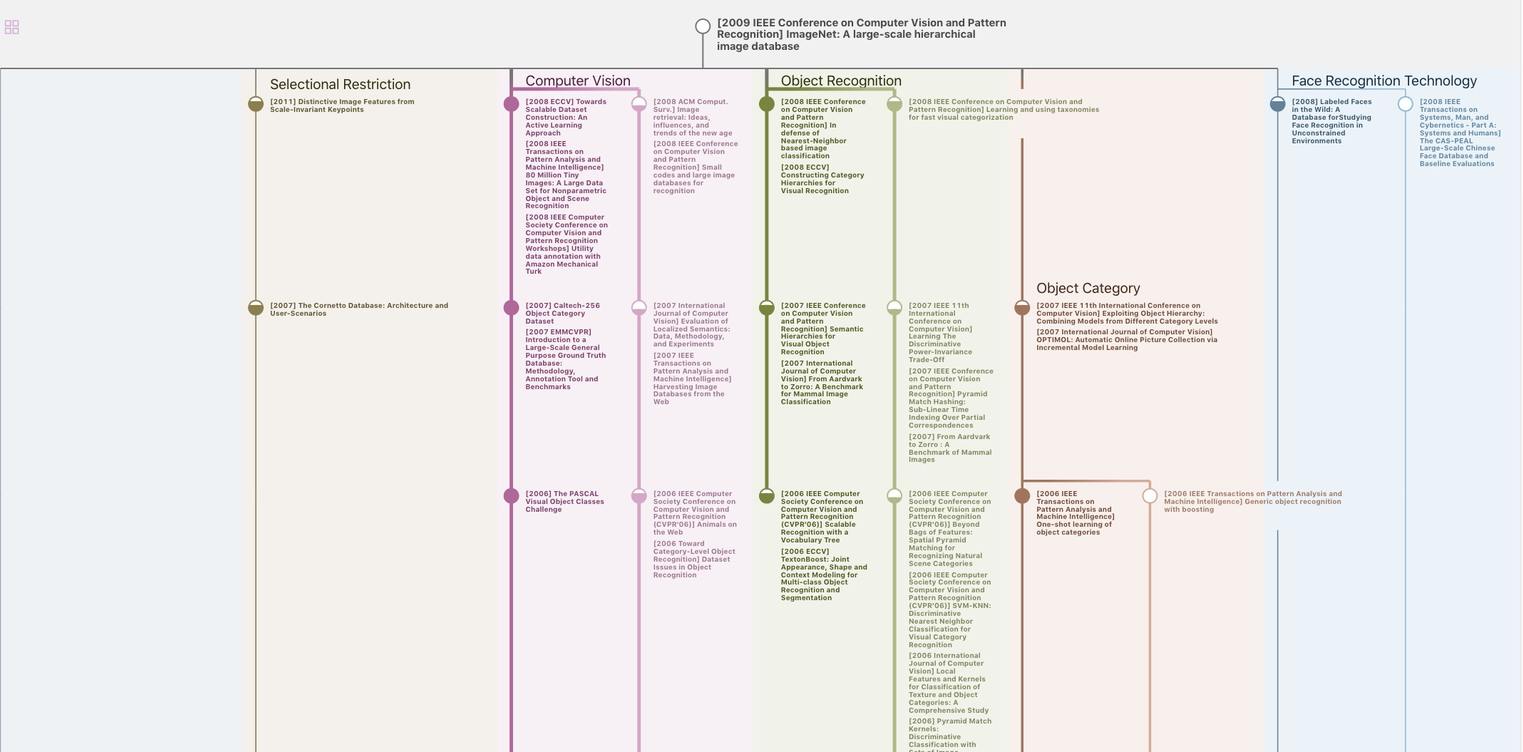
生成溯源树,研究论文发展脉络
Chat Paper
正在生成论文摘要