Predicting Home and Work Locations Using Public Transport Smart Card Data by Spectral Analysis
ITSC(2015)
摘要
Knowledge of home and work locations of population is essential for transport planning and urban development. We present a method to predict and validate home and work locations of commuters using their public transport smart card data. Existing works rely on statistical techniques to interpret public transport transactions. Our method goes one step further by exploring the underlying repeated travelling patterns between home and work place. It first uses several features extracted from a card holder's ride records (i.e., the length of time that he/she stays at a location, the times he/she visits a place, etc.) to evaluate the likelihood of a location is associated with the card holder's home/work places. Rank aggregation technique is then applied to derive a comprehensive location ranking list. A few top ranked locations are picked out for further analysis, where the travel records between any two of them are arranged as a time series. Spectral analysis is applied on the time series in attempt to identify periodic travelling patterns. The most likely home/work pair is selected based on a proper period and dominant frequency which implies regular travelling activities between the card holder's home and work place. Finally, home and work locations are discriminated based on the assumption that a person goes to his/her work place mostly on weekdays and rarely on weekends. We apply our method on a three-month Singapore smart card dataset and evaluate the result against a ground truth obtained from Singapore urban planning programme. Validation results show that the proposed method is able to predict meaningful home and work places.
更多查看译文
关键词
feature extraction,public transport,smart cards,time series,town and country planning,Singapore smart card dataset,Singapore urban planning programme,feature extraction,home location prediction,public transport smart card data,rank aggregation technique,repeated travelling patterns,spectral analysis,time series,transport planning,urban development,work location prediction
AI 理解论文
溯源树
样例
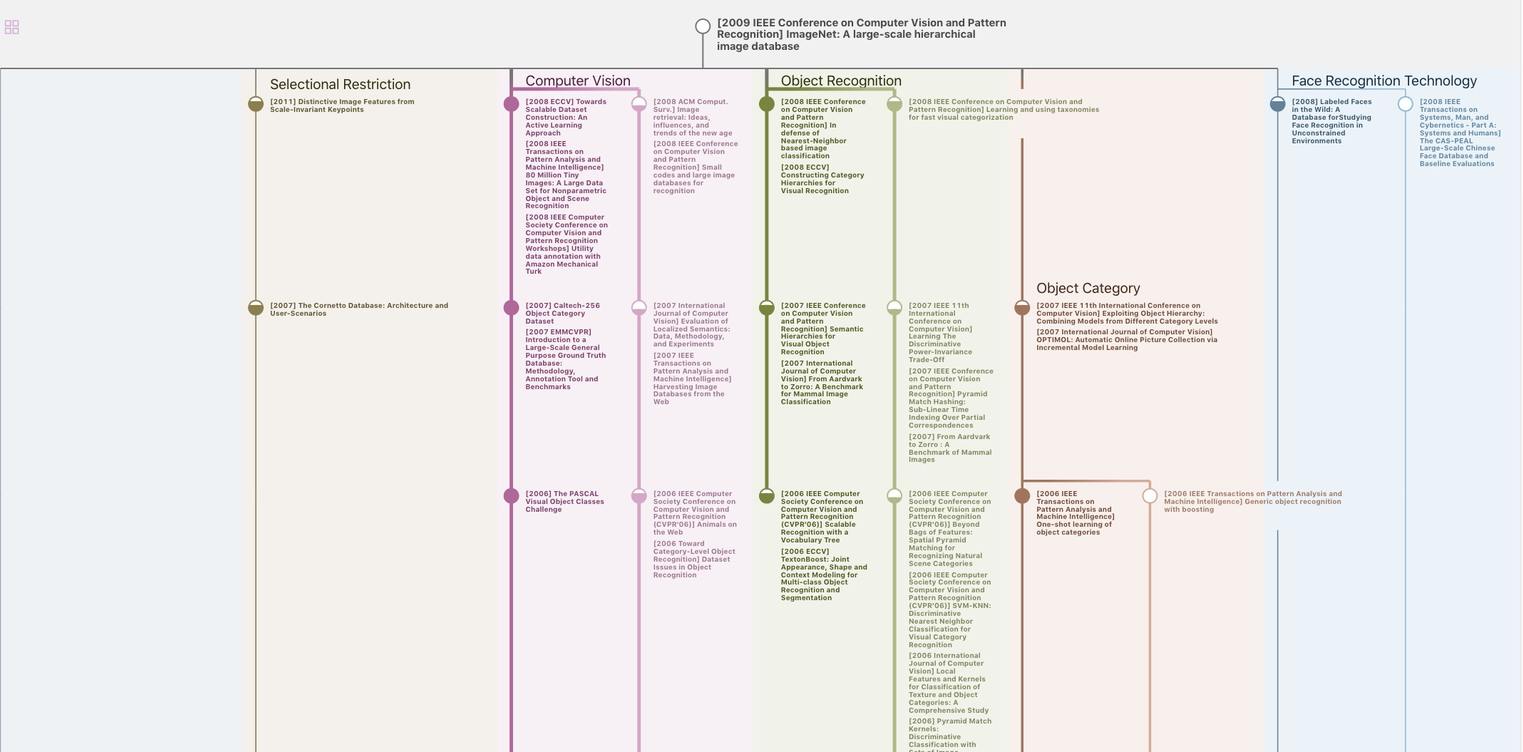
生成溯源树,研究论文发展脉络
Chat Paper
正在生成论文摘要