Quantifying and Visualizing Uncertainties in Molecular Models.
arXiv: Computational Engineering, Finance, and Science(2015)
摘要
Computational molecular modeling and visualization has seen significant progress in recent years with sev- eral molecular modeling and visualization software systems in use today. Nevertheless the molecular biology community lacks techniques and tools for the rigorous analysis, quantification and visualization of the associated errors in molecular structure and its associated properties. This paper attempts at filling this vacuum with the introduction of a systematic statistical framework where each source of structural uncertainty is modeled as a ran- dom variable (RV) with a known distribution, and properties of the molecules are defined as dependent RVs. The framework consists of a theoretical basis, and an empirical implementation where the uncertainty quantification (UQ) analysis is achieved by using Chernoff-like bounds. The framework enables additionally the propagation of input structural data uncertainties, which in the molecular protein world are described as B-factors, saved with almost all X-ray models deposited in the Protein Data Bank (PDB). Our statistical framework is also able and has been applied to quantify and visualize the uncertainties in molecular properties, namely solvation interfaces and solvation free energy estimates. For each of these quantities of interest (QOI) of the molecular models we provide several novel and intuitive visualizations of the input, intermediate, and final propagated uncertainties. These methods should enable the end user achieve a more quantitative and visual evaluation of various molecular PDB models for structural and property correctness, or the lack thereof.
更多查看译文
AI 理解论文
溯源树
样例
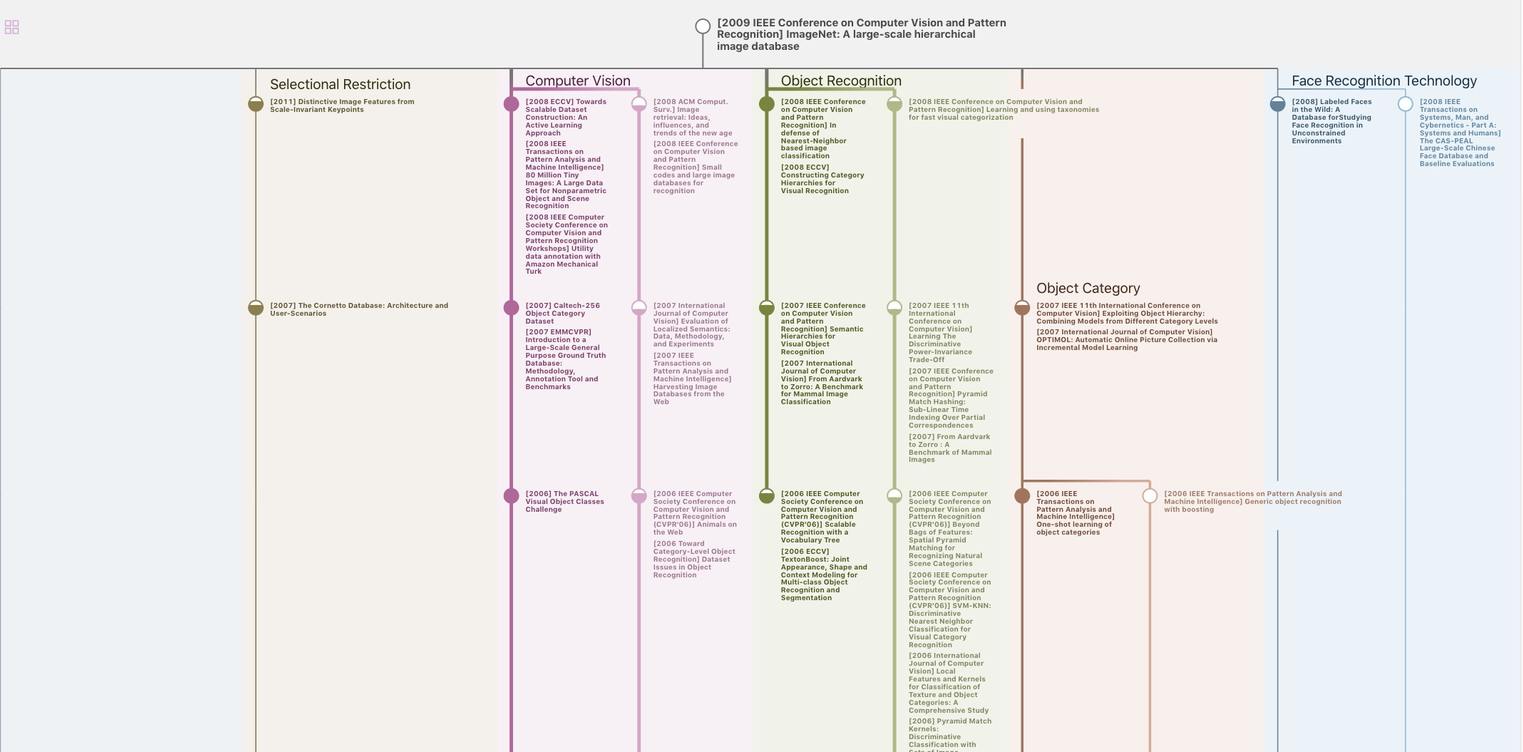
生成溯源树,研究论文发展脉络
Chat Paper
正在生成论文摘要