Multi-View Semi-Supervised Learning For Web Image Annotation
MM '15: ACM Multimedia Conference Brisbane Australia October, 2015(2015)
摘要
With the explosive increasing of web image data, image annotation has become a critical research issue for image semantic index and search. In this work, we propose a novel model, termed as multi-view semi-supervised learning (MVSSL), for robust image annotation task. Specifically, we exploit both labeled images and unlabeled images to uncover the intrinsic data structural information. Meanwhile, to comprehensively describe an individual datum, we take advantage of the correlated and complemental information derived from multiple facets of image data (i.e., multiple views or features). We devise a robust pair-wise constraint on outcomes of different views to achieve annotation consistency. Furthermore, we integrate a robust classifier learning component via l(2,1) loss, which can provide effective noise identification power during the learning process. Finally, we devise an efficient iterative algorithm to solve the optimization problem in MVSSL. We conduct extensive experiments on the NUS-WIDE dataset, and the results illustrate that our proposed approach is promising for large scale web image annotation task.
更多查看译文
关键词
multi-view learning,semi-supervised learning,image annotation
AI 理解论文
溯源树
样例
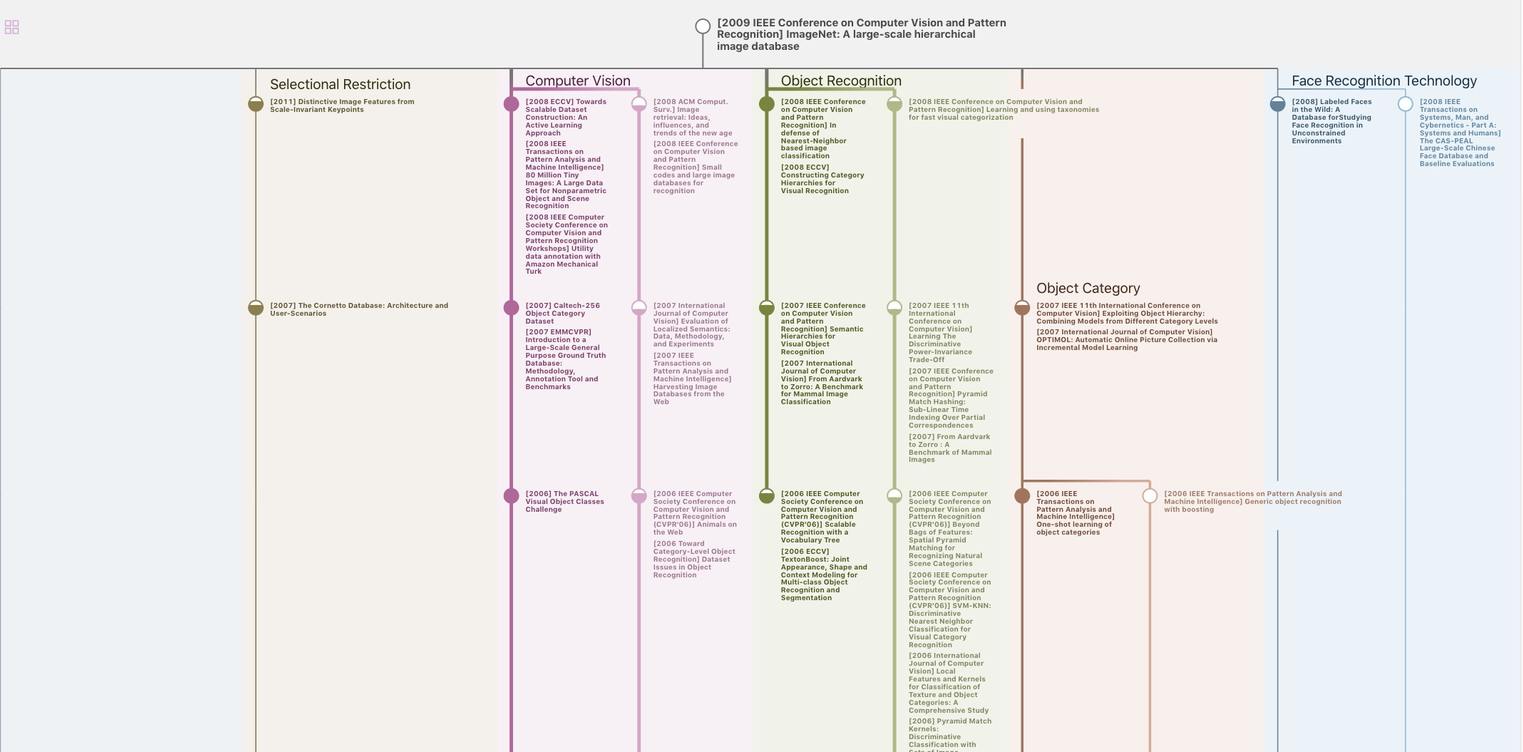
生成溯源树,研究论文发展脉络
Chat Paper
正在生成论文摘要