Unsupervised Learning Of Spatial-Temporal Models Of Objects In A Long-Term Autonomy Scenario
2015 IEEE/RSJ International Conference on Intelligent Robots and Systems (IROS)(2015)
摘要
We present a novel method for clustering segmented dynamic parts of indoor RGB-D scenes across repeated observations by performing an analysis of their spatial-temporal distributions. We segment areas of interest in the scene using scene differencing for change detection. We extend the Meta-Room method and evaluate the performance on a complex dataset acquired autonomously by a mobile robot over a period of 30 days. We use an initial clustering method to group the segmented parts based on appearance and shape, and we further combine the clusters we obtain by analyzing their spatial-temporal behaviors. We show that using the spatial-temporal information further increases the matching accuracy.
更多查看译文
关键词
spatial-temporal information,mobile robot,Meta-Room method,change detection,scene differencing,red-green-blue-delta scene,indoor RGB-D scene clustering,long-term autonomy scenario,object spatial-temporal model,unsupervised learning
AI 理解论文
溯源树
样例
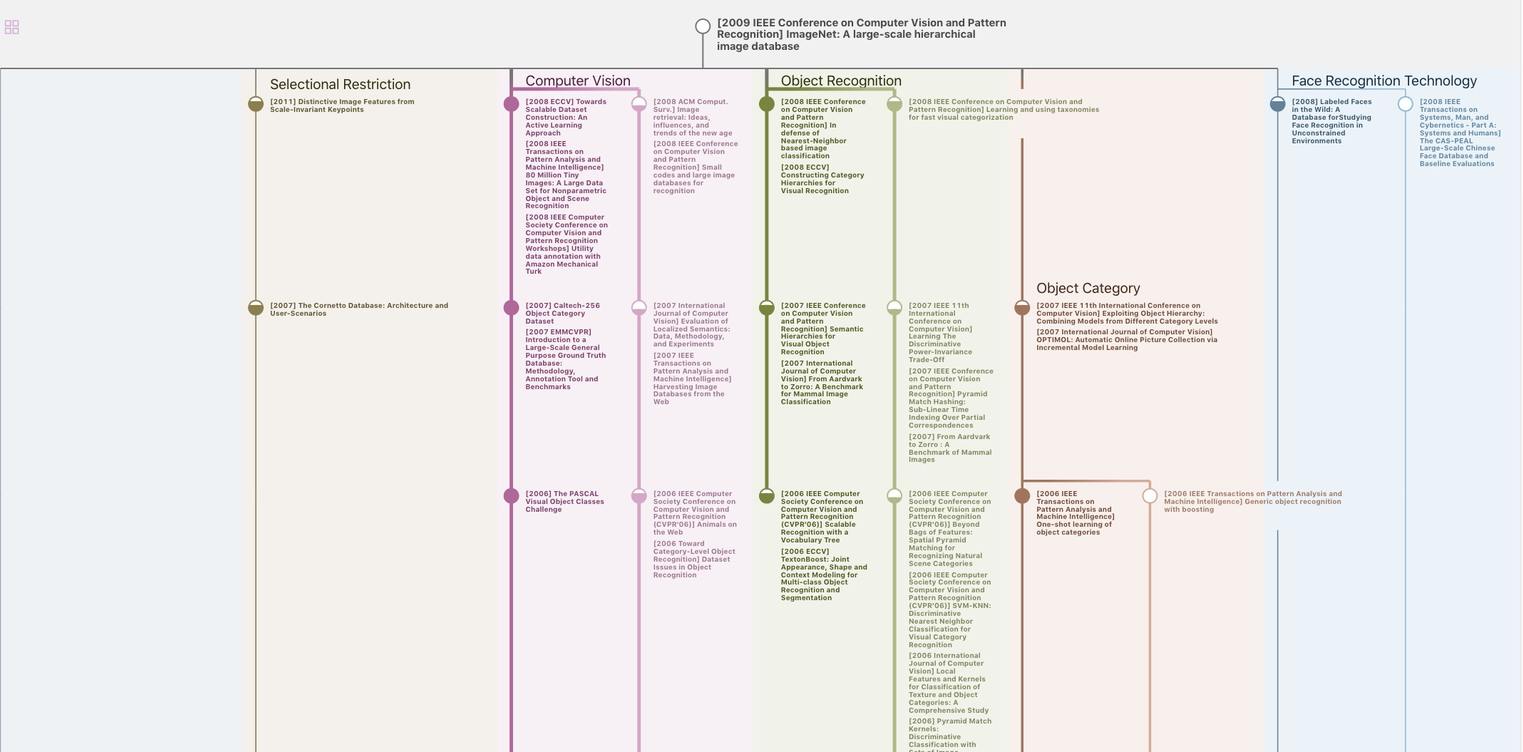
生成溯源树,研究论文发展脉络
Chat Paper
正在生成论文摘要