Generalization Bounds for Supervised Dimensionality Reduction.
FE@NIPS(2015)
摘要
We introduce and study the learning scenario of supervised dimensionality reduction, which couples dimensionality reduction and a subsequent supervised learning step. We present new generalization bounds for this scenario based on a careful analysis of the empirical Rademacher complexity of the relevant hypothesis set. In particular, we show an upper bound on the Rademacher complexity that is in O( √ Λ(r)/m), where m is the sample size and Λ(r) the upper bound on the Ky-Fan r-norm of the operator that defines the dimensionality reduction projection. We give both upper and lower bounds in terms of that Ky-Fan r-norm, which strongly justifies the definition of our hypothesis set. To the best of our knowledge, these are the first learning guarantees for the problem of supervised dimensionality reduction with a learned kernel-based mapping. Our analysis and learning guarantees further apply to several special cases, such as that of using a fixed kernel with supervised dimensionality reduction or that of unsupervised learning of a kernel for dimensionality reduction followed by a supervised learning algorithm.
更多查看译文
AI 理解论文
溯源树
样例
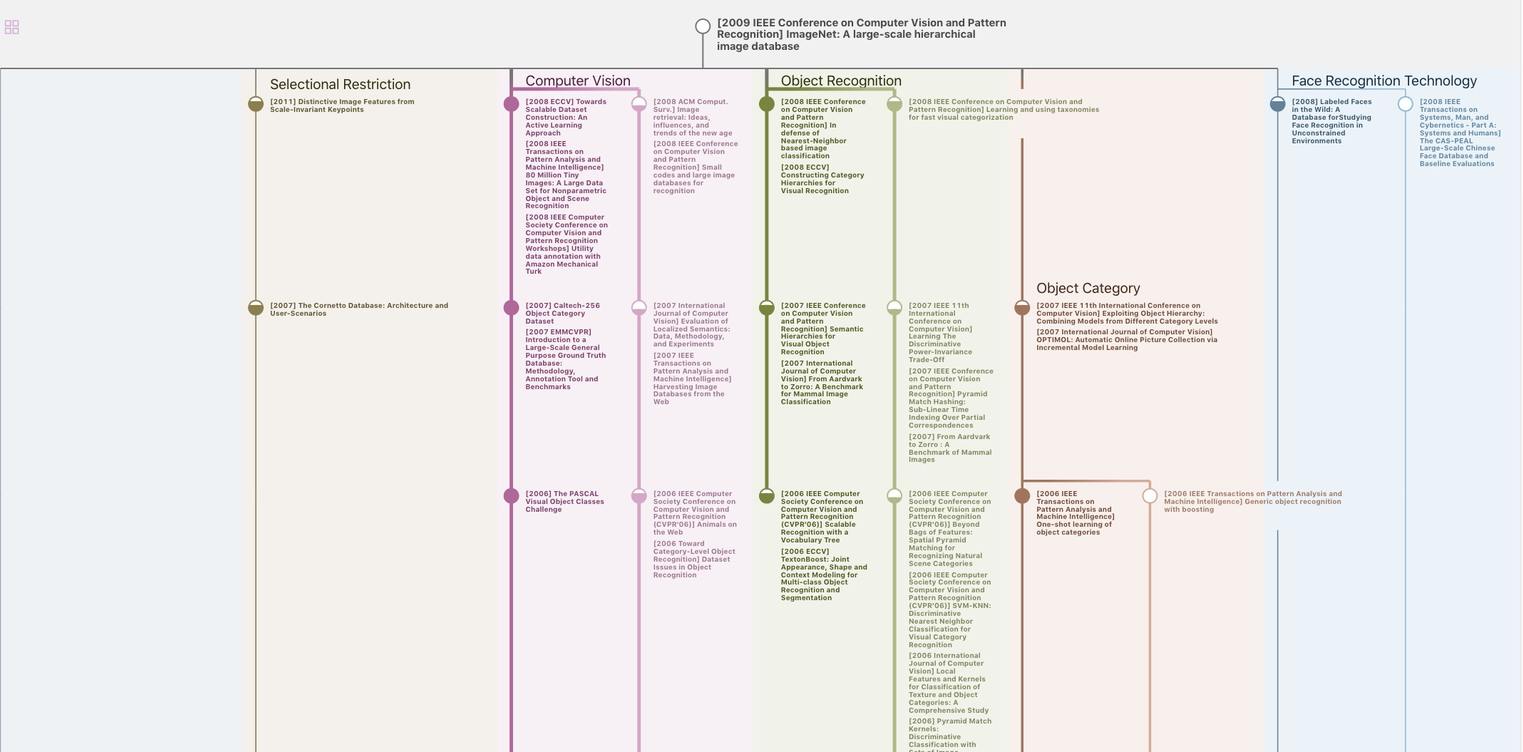
生成溯源树,研究论文发展脉络
Chat Paper
正在生成论文摘要