STEPS: Predicting place attributes via spatio-temporal analysis
arXiv: Social and Information Networks(2016)
摘要
In recent years, a vast amount of research has been conducted on learning people's interests from their actions. Yet their collective actions also allow us to learn something about the world, in particular, infer attributes of places people visit or interact with. Imagine classifying whether a hotel has a gym or a swimming pool, or whether a restaurant has a romantic atmosphere without ever asking its patrons. Algorithms we present can do just that. Many web applications rely on knowing attributes of places, for instance, whether a particular restaurant has WiFi or offers outdoor seating. Such data can be used to support a range of user experiences, from explicit query-driven search to personalized place recommendations. However, obtaining these attributes is generally difficult, with existing approaches relying on crowdsourcing or parsing online reviews, both of which are noisy, biased, and have limited coverage. Here we present a novel approach to classifying place attributes, which learns from patrons' visit patterns based on anonymous observational data. Our method, STEPS, learns from aggregated sequences of place visits. For example, if many people visit the restaurant on a Saturday evening, coming from a luxury hotel or theater, and stay for a long time, then this restaurant is more likely to have a romantic atmosphere. On the other hand, if most people visit the restaurant on weekdays, coming from work or a grocery store, then the restaurant is less likely to be romantic. We show that such transition features are highly predictive of place attributes. In an extensive empirical evaluation, STEPS nearly doubled the coverage of a state of the art approach thanks to learning from observational location data, which allowed our method to reason about many more places.
更多查看译文
AI 理解论文
溯源树
样例
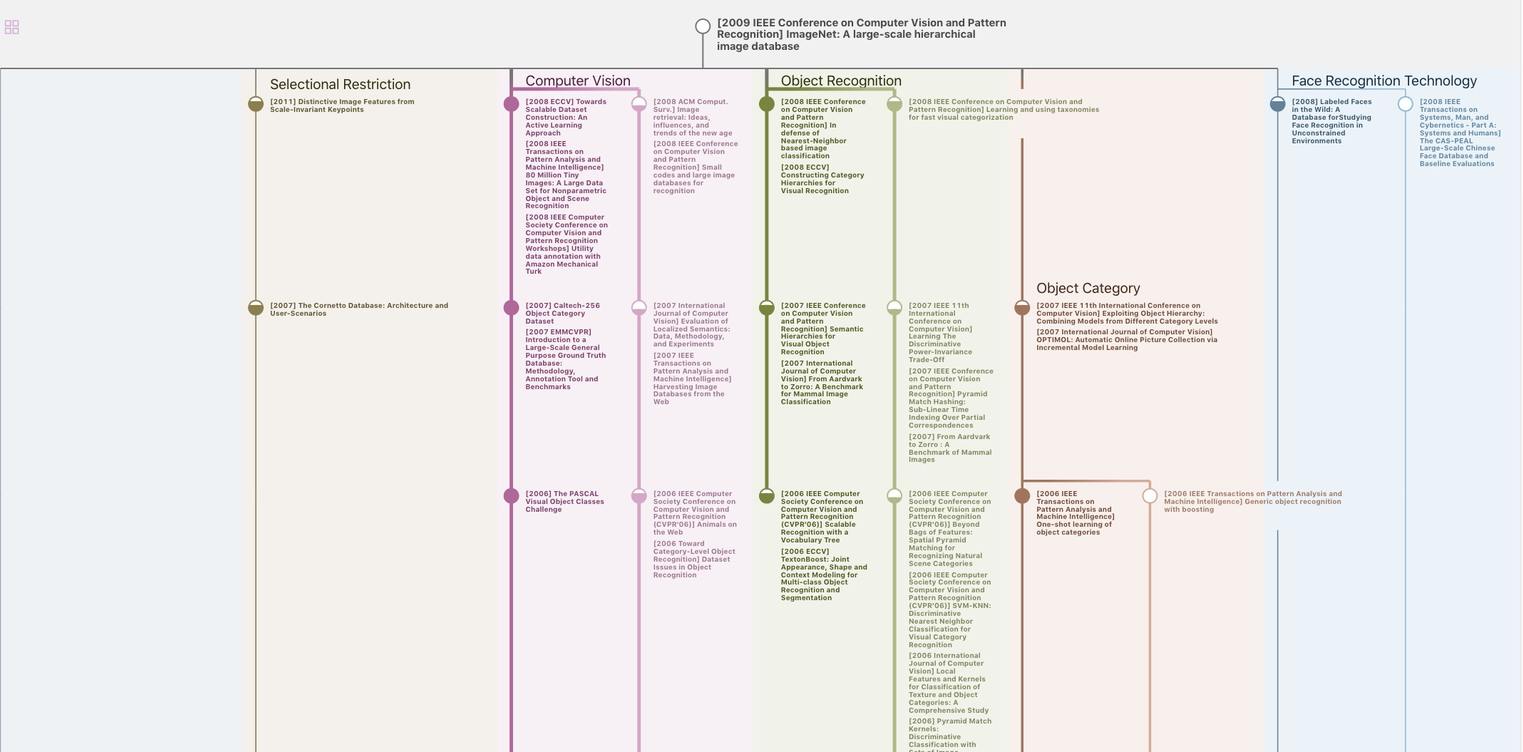
生成溯源树,研究论文发展脉络
Chat Paper
正在生成论文摘要