End-to-End Learning for Structured Prediction Energy Networks.
ICML(2017)
摘要
Structured Prediction Energy Networks (Belanger and McCallum, 2016) (SPENs) are a simple, yet expressive family of structured prediction models. An energy function over candidate structured outputs is given by a deep network, and predictions are formed by gradient-based optimization. Unfortunately, we have struggled to apply the structured SVM (SSVM) learning method of Belanger and McCallum, 2016 to applications with more complex structure than multi-label classification. In general, SSVMs are unreliable whenever exact energy minimization is intractable. In response, we present end-to-end learning for SPENs, where the energy function is discriminatively trained by back-propagating through gradient-based prediction. This paper presents a collection of methods necessary to apply the technique to problems with complex structure. For example, we avoid vanishing gradients when learning SPENs for convex relaxations of discrete prediction problems and explicitly train models such that energy minimization converges quickly in practice. Using end-to-end learning, we demonstrate the power of SPENs on 7-Scenes depth image denoising and CoNLL-2005 semantic role labeling tasks. In both, we outperform competitive baselines that employ more simplistic energy functions, but perform exact energy minimization. In particular, for denoising we achieve 40 PSNR, outperforming the previous state-of-the-art of 36.
更多查看译文
AI 理解论文
溯源树
样例
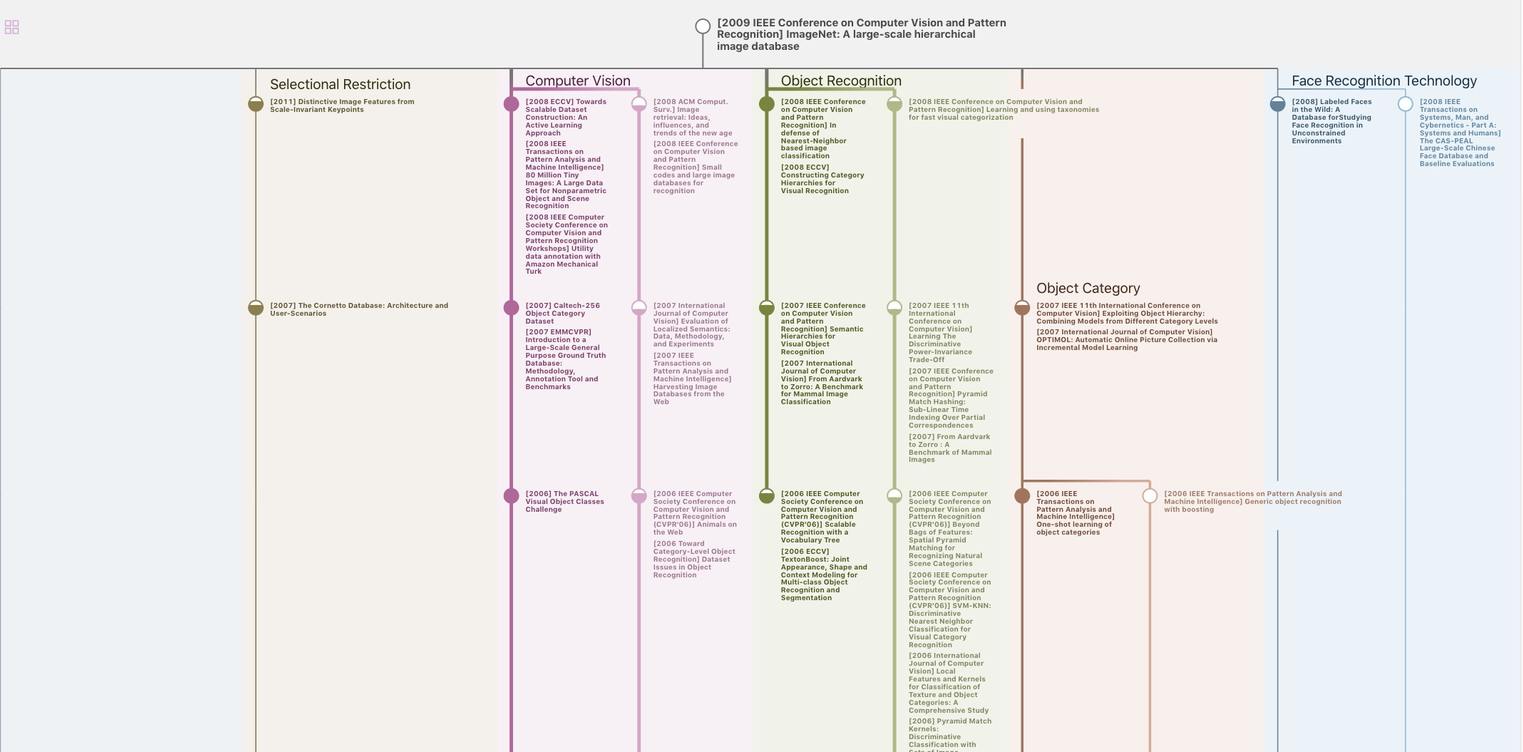
生成溯源树,研究论文发展脉络
Chat Paper
正在生成论文摘要