Belief Space Stochastic Control Under Unknown Dynamics
2017 AMERICAN CONTROL CONFERENCE (ACC)(2017)
摘要
We present a sampling-based stochastic optimal control (SOC) framework for systems with unknown dynamics based on the path integral formulation and probabilistic inference. This work is motivated by three major limitations of related SOC methods: first, full knowledge of the dynamics model is usually required. Second, model uncertainty is neglected. Third, convergence of the iterative scheme is quite slow. In order to cope with these issues, our method performs sampling in belief space using approximate inference in probabilistic models. When performing probability-weighted averaging, each sample is weighted by its predictive uncertainty. In addition, our method leverages covariance matrix adaptation to achieve faster convergence. We demonstrate the effectiveness and efficiency of the proposed method using a simulated cart-pole swing up task.
更多查看译文
关键词
sampling-based stochastic optimal control framework,belief space stochastic control,probabilistic inference,path integral formulation,SOC methods,iterative scheme,approximate inference,probability-weighted averaging,covariance matrix adaptation,simulated cart-pole swing up task
AI 理解论文
溯源树
样例
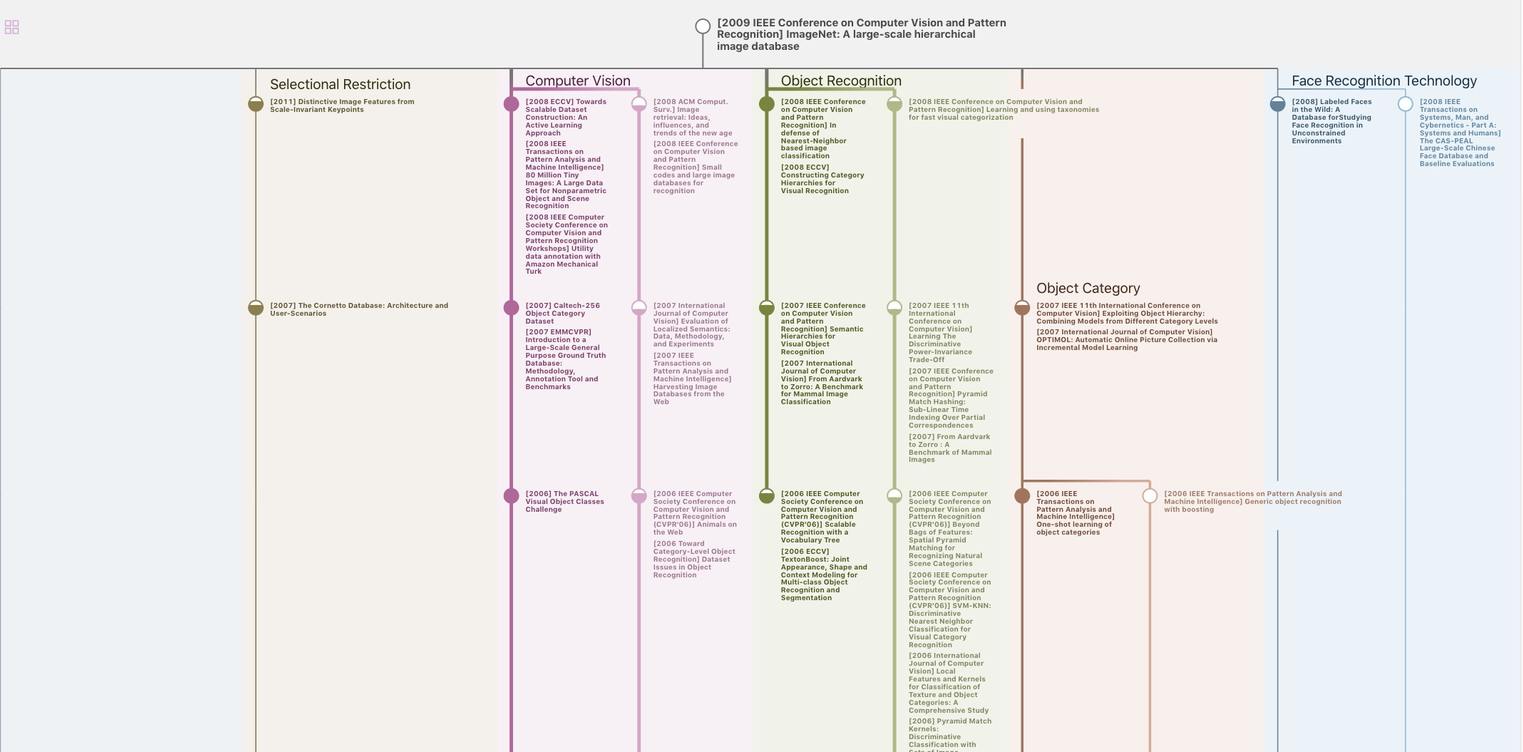
生成溯源树,研究论文发展脉络
Chat Paper
正在生成论文摘要