General Knowledge Embedded Image Representation Learning.
IEEE Trans. Multimedia(2018)
摘要
Image representation learning is a fundamental problem in understanding semantics of images. However, traditional classification-based representation learning methods face the noisy and incomplete problem of the supervisory labels. In this paper, we propose a General Knowledge Base Embedded Image Representation Learning approach, which uses general knowledge graph, which is a multi-type relational knowledge graph consisting of human commonsense beyond image space, as external semantic resource to capture the relations of concepts in image representation learning. A Relational Regularized Regression CNN (R $^{3}$ CNN) model is designed to jointly optimize the image representation learning problem and knowledge graph embedding problem. In this manner, the learnt representation can capture not only labeled tags but also related concepts of images, which involves more precise and complete semantics. Comprehensive experiments are conducted to investigate the effectiveness and transferability of our approach in tag prediction task, zero-shot tag inference task, and content based image retrieval task. The experimental results demonstrate that the proposed approach performs significantly better than the existing representation learning methods. Finally, observation of the learnt relations show that our approach can somehow refine the knowledge base to describe images and label the images with structured tags.
更多查看译文
关键词
Image representation,Semantics,Visualization,Knowledge based systems,Learning systems,Noise measurement,Bridges
AI 理解论文
溯源树
样例
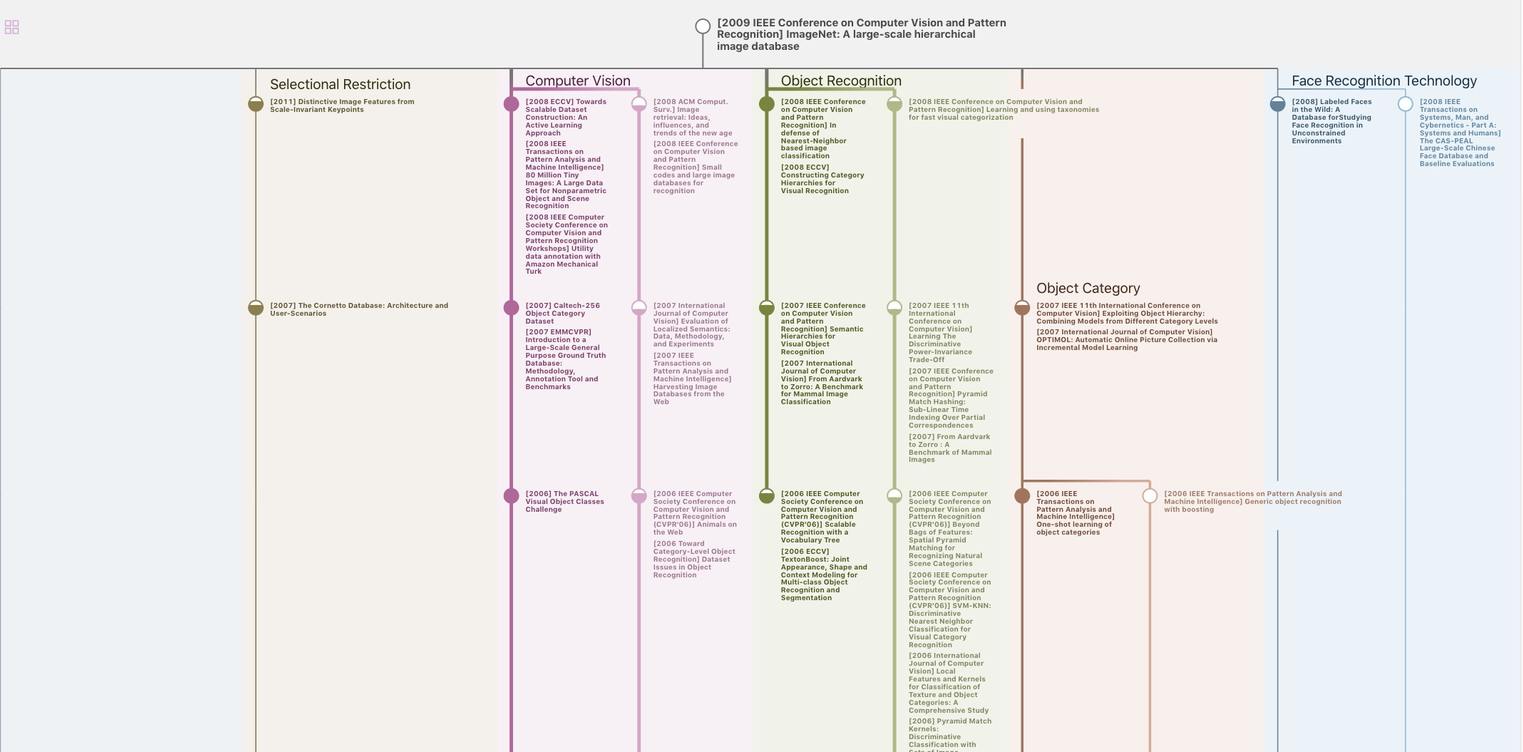
生成溯源树,研究论文发展脉络
Chat Paper
正在生成论文摘要