Multi-Channel Apollo Mission Speech Transcripts Calibration
18TH ANNUAL CONFERENCE OF THE INTERNATIONAL SPEECH COMMUNICATION ASSOCIATION (INTERSPEECH 2017), VOLS 1-6: SITUATED INTERACTION(2017)
摘要
NASA's Apollo program is a great achievement of mankind in the 20th century. Previously we had introduced UTD-CRSS Apollo data digitization initiative where we proposed to digitize Apollo mission speech data (-100,000 hours) and develop Spoken Language Technology based algorithms to analyze and understand various aspects of conversational speech[1]. A new 30 track analog audio decoder is designed to decode 30 track Apollo analog tapes and is mounted on to the NASA Sound-scriber analog audio decoder (in place of single channel decoder). Using the new decoder all 30 channels of data can be decoded simultaneously thereby reducing the digitization time significantly. We have digitized 19,000 hours of data from Apollo missions (including entire Apollo-11, most of Apollo-13. Apollo-1, and Gemini-8 missions). Each audio track corresponds to a specific personnel/position in NASA mission control room or astronauts in space. Since many of the planned Apollo related spoken language technology approaches need transcripts we have developed an Apollo mission specific custom Deep Neural Networks (DNN) based Automatic Speech Recognition (ASR) system. Apollo specific language models are developed. Most audio channels are degraded due to high channel noise, system noise. attenuated signal bandwidth, transmission noise, cosmic noise, analog tape static noise, noise due to tape aging, etc,. In this paper we propose a novel method to improve the transcript quality by using Signal-to-Noise ratio of channels and N-Gram sentence similarity metrics across data channels. The proposed method shows significant improvement in transcript quality of noisy channels. The Word Error Rate (WER) analysis of transcripts across channels shows significant reduction.
更多查看译文
关键词
Speech recognition, Apollo mission, Multi-Track speech, Transcript enhancement
AI 理解论文
溯源树
样例
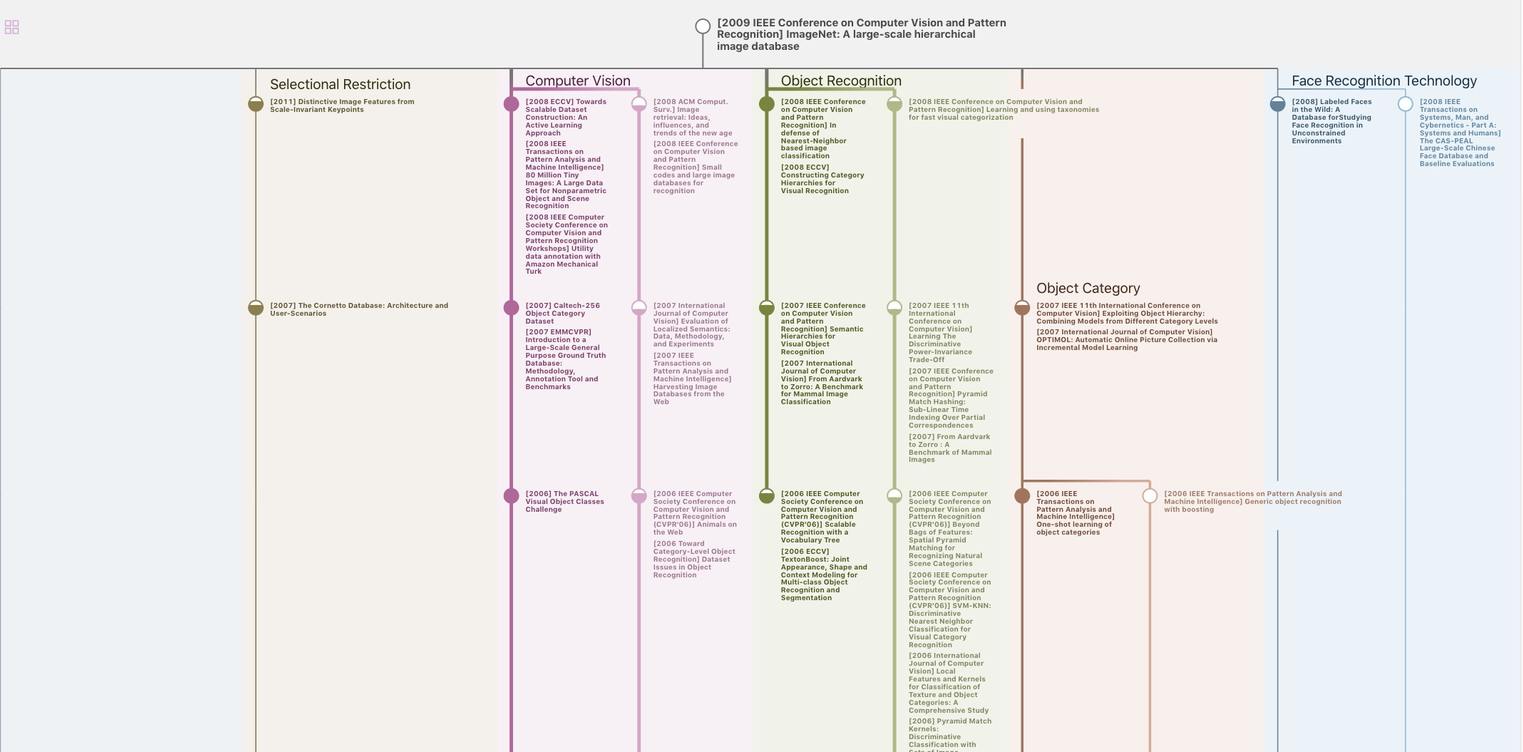
生成溯源树,研究论文发展脉络
Chat Paper
正在生成论文摘要