Learning binary codes with local and inner data structure.
Neurocomputing(2018)
摘要
Recent years have witnessed the promising capacity of hashing techniques in tackling nearest neighbor search because of the high efficiency in storage and retrieval. Data-independent approaches (e.g., Locality Sensitive Hashing) normally construct hash functions using random projections, which neglect intrinsic data properties. To compensate this drawback, learning-based approaches propose to explore local data structure and/or supervised information for boosting hashing performance. However, due to the construction of Laplacian matrix, existing methods usually suffer from the unaffordable training cost. In this paper, we propose a novel supervised hashing scheme, which has the merits of (1) exploring the inherent neighborhoods of samples; (2) significantly saving training cost confronted with massive training data by employing approximate anchor graph; as well as (3) preserving semantic similarity by leveraging pair-wise supervised knowledge. Besides, we integrate discrete constraint to significantly eliminate accumulated errors in learning reliable hash codes and hash functions. We devise an alternative algorithm to efficiently solve the optimization problem. Extensive experiments on various image datasets demonstrate that our proposed method is superior to the state-of-the-arts.
更多查看译文
关键词
Supervised hashing,Anchor graph,Nearest neighbor search
AI 理解论文
溯源树
样例
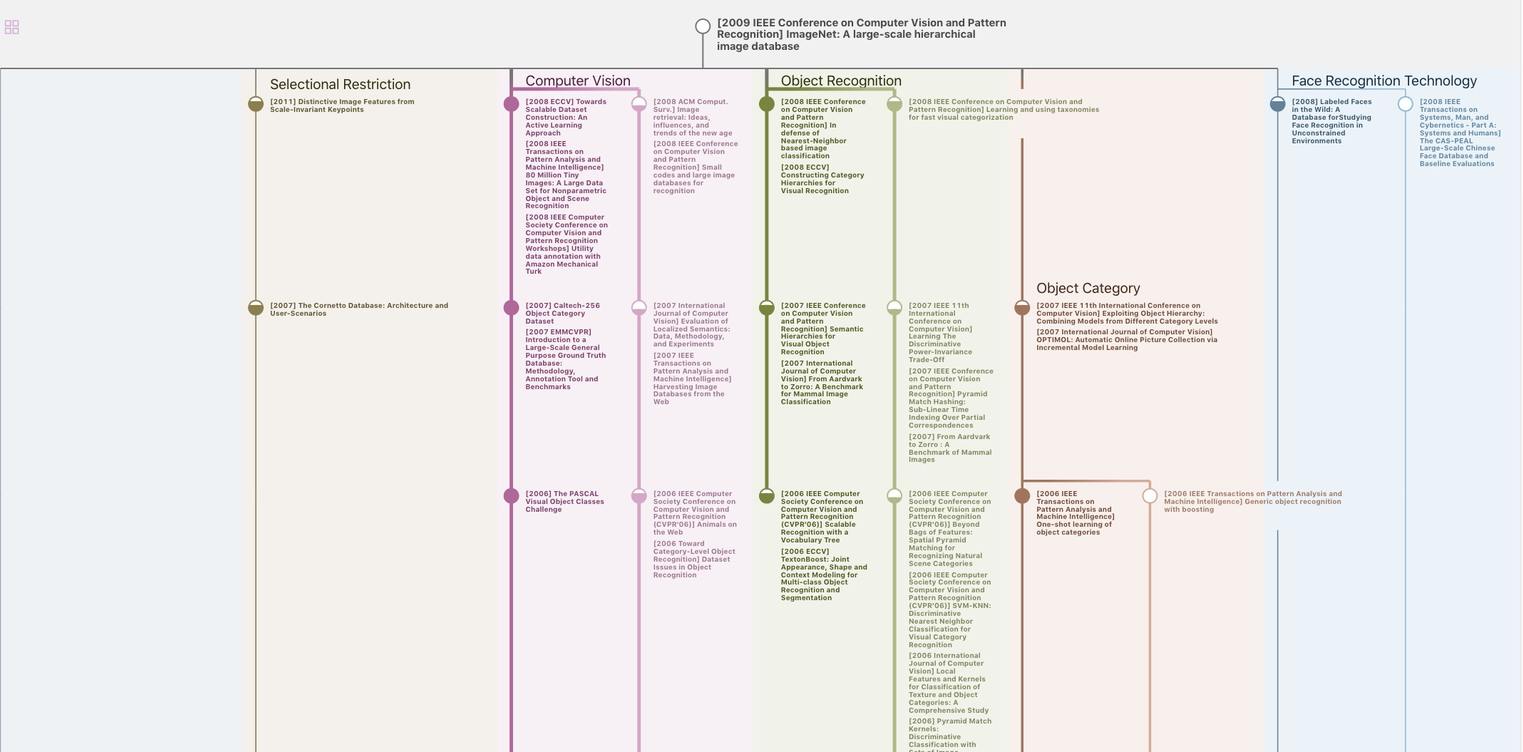
生成溯源树,研究论文发展脉络
Chat Paper
正在生成论文摘要