Learning Task-Specific Dynamics to Improve Whole-Body Control
2018 IEEE-RAS 18th International Conference on Humanoid Robots (Humanoids)(2018)
摘要
In task-based inverse dynamics control, reference accelerations used to follow a desired plan can be broken down into feedforward and feedback trajectories. The feedback term accounts for tracking errors that are caused from inaccurate dynamic models or external disturbances. On underactuated, free-floating robots, such as humanoids, good tracking accuracy often necessitates high feedback gains, which leads to undesirable stiff behaviors. The magnitude of these gains is anyways often strongly limited by the control bandwidth. In this paper, we show how to reduce the required contribution of the feedback controller by incorporating learned task-space reference accelerations. Thus, we i) improve the execution of the given specific task, and ii) offer the means to reduce feedback gains, providing for greater compliance of the system. In contrast to learning task-specific joint-torques, which might produce a similar effect but can lead to poor generalization, our approach directly learns the task-space dynamics of the center of mass of a humanoid robot. Simulated and real-world results on the lower part of the Sarcos Hermes humanoid robot demonstrate the applicability of the approach.
更多查看译文
关键词
task-specific dynamics learning,stiff behaviors,task-specific joint-torques learning,Sarcos Hermes humanoid robot,task-space dynamics,learned task-space reference accelerations,feedback controller,control bandwidth,feedback term accounts,feedback trajectories,feedforward,task-based inverse dynamics control,whole-body control
AI 理解论文
溯源树
样例
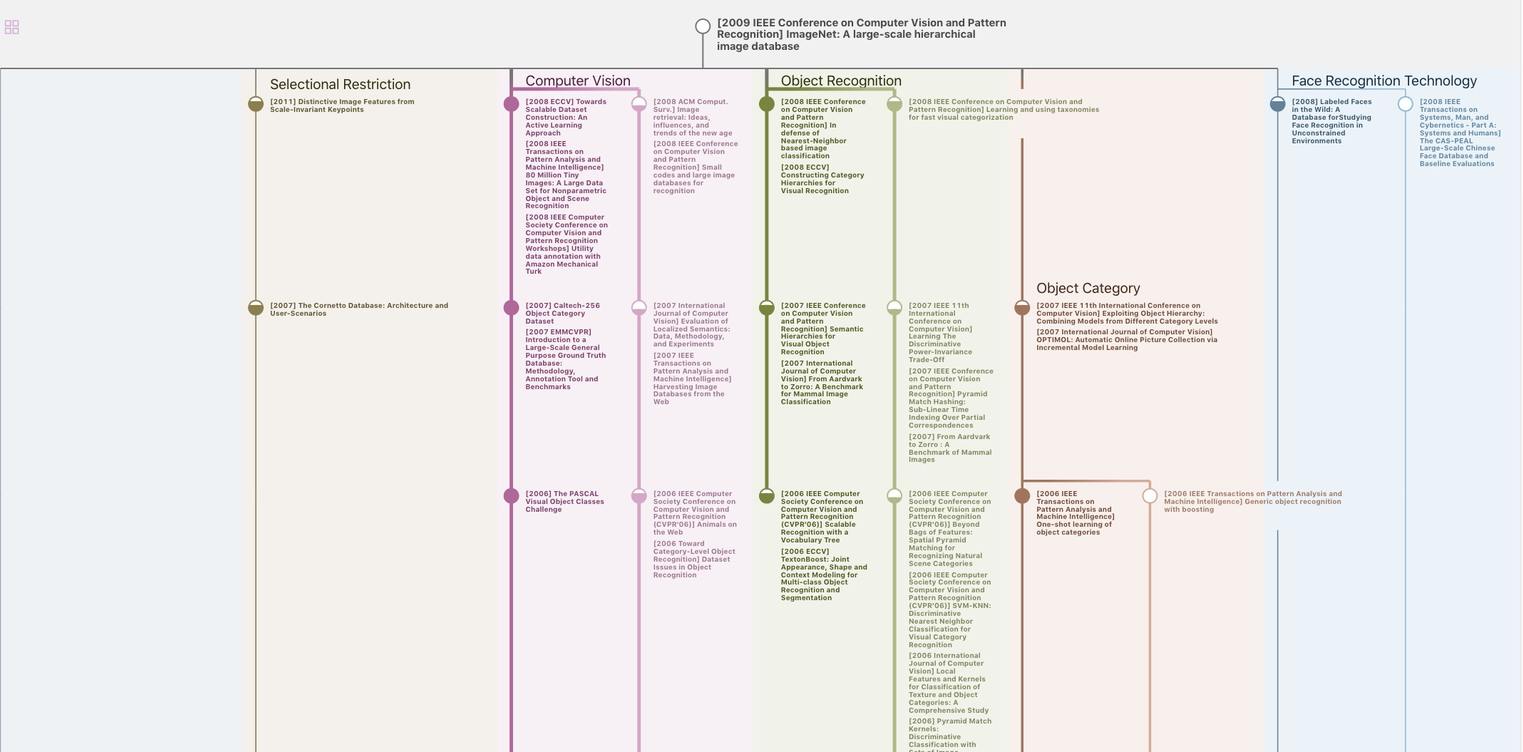
生成溯源树,研究论文发展脉络
Chat Paper
正在生成论文摘要