Mining Human Periodic Behaviors Using Mobility Intention and Relative Entropy.
ADVANCES IN KNOWLEDGE DISCOVERY AND DATA MINING, PAKDD 2018, PT I(2018)
摘要
Human periodic behaviors is essential to many applications, and many research work show that human behaviors are periodic. However, existing human periodic works are reported with limited improvements in using periodicity of locations and unsatisfactory accuracy for oscillation of human periodic behaviors. To address these challenges, in this paper we propose a Mobility Intention and Relative Entropy (MIRE) model. We use mobility intentions extracting from dataset by tensor decomposition to characterize users' history records, and use subsequence of same mobility intention to mine human periodic behaviors. A new periodicity detection algorithm based on relative entropy is then proposed. The experimental results on real-world datasets demonstrate that the proposed MIRE model can properly mining human periodic behaviors. The comparison results also indicate that MIRE model significantly outperforms state-of-the-art periodicity detection algorithms.
更多查看译文
AI 理解论文
溯源树
样例
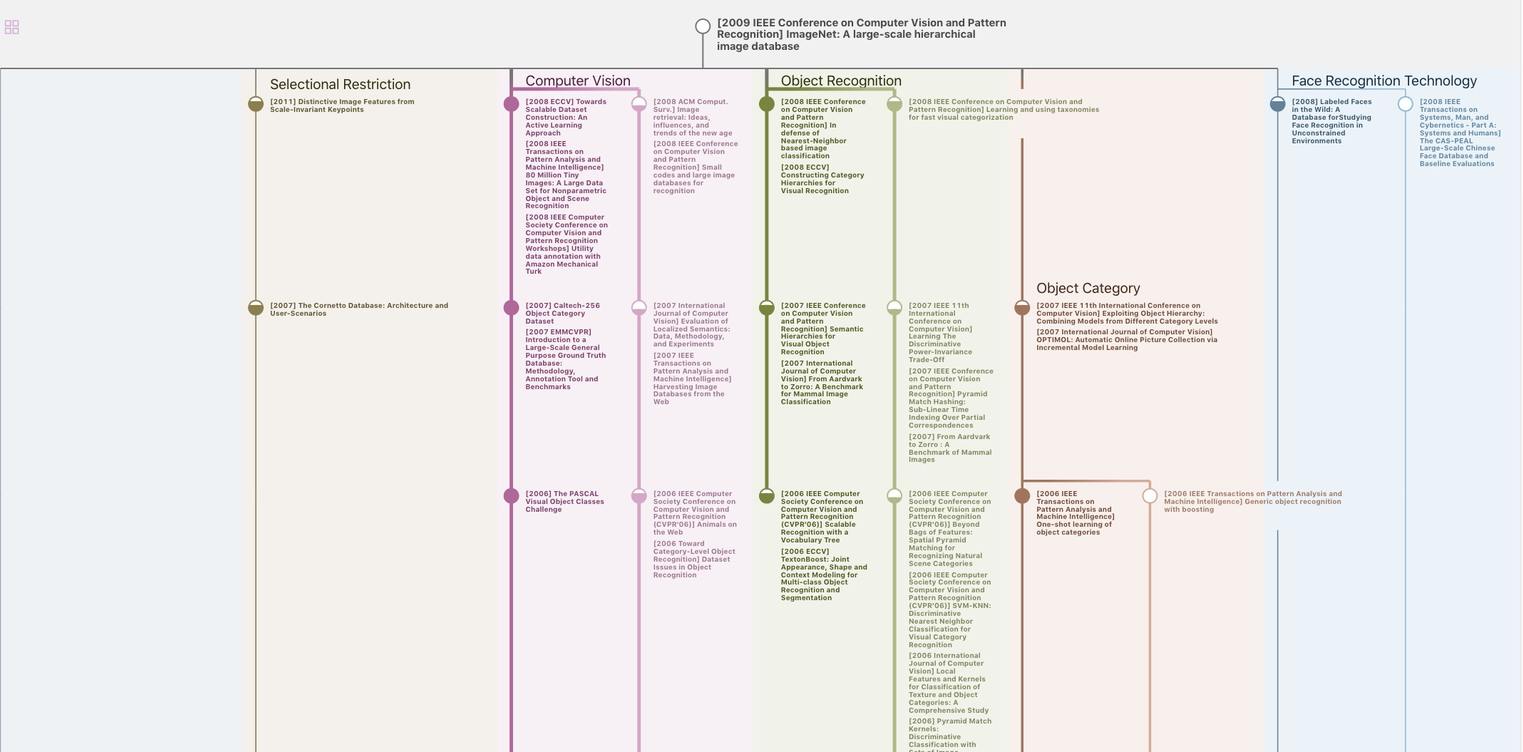
生成溯源树,研究论文发展脉络
Chat Paper
正在生成论文摘要