Backward Reduction of CNN Models with Information Flow Analysis.
arXiv: Learning(2018)
摘要
This paper proposes backward reduction, an algorithm that explores the compact CNN design from the information flow perspective. This algorithm can remove substantial non-zero weighting parameters (redundant neural channels) by considering the network dynamic behavior, which the traditional model compaction techniques cannot achieve, to reduce the size of a model. With the aid of our proposed algorithm, we achieve significant model reduction results of ResNet-34 in ImageNet scale (32.3% reduction), which is 3X better than the state-of-the-art result (10.8%). Even for highly optimized models like SqueezeNet and MobileNet, we still achieve additional 10.81% and 37.56% reduction, respectively, with negligible performance degradation.
更多查看译文
AI 理解论文
溯源树
样例
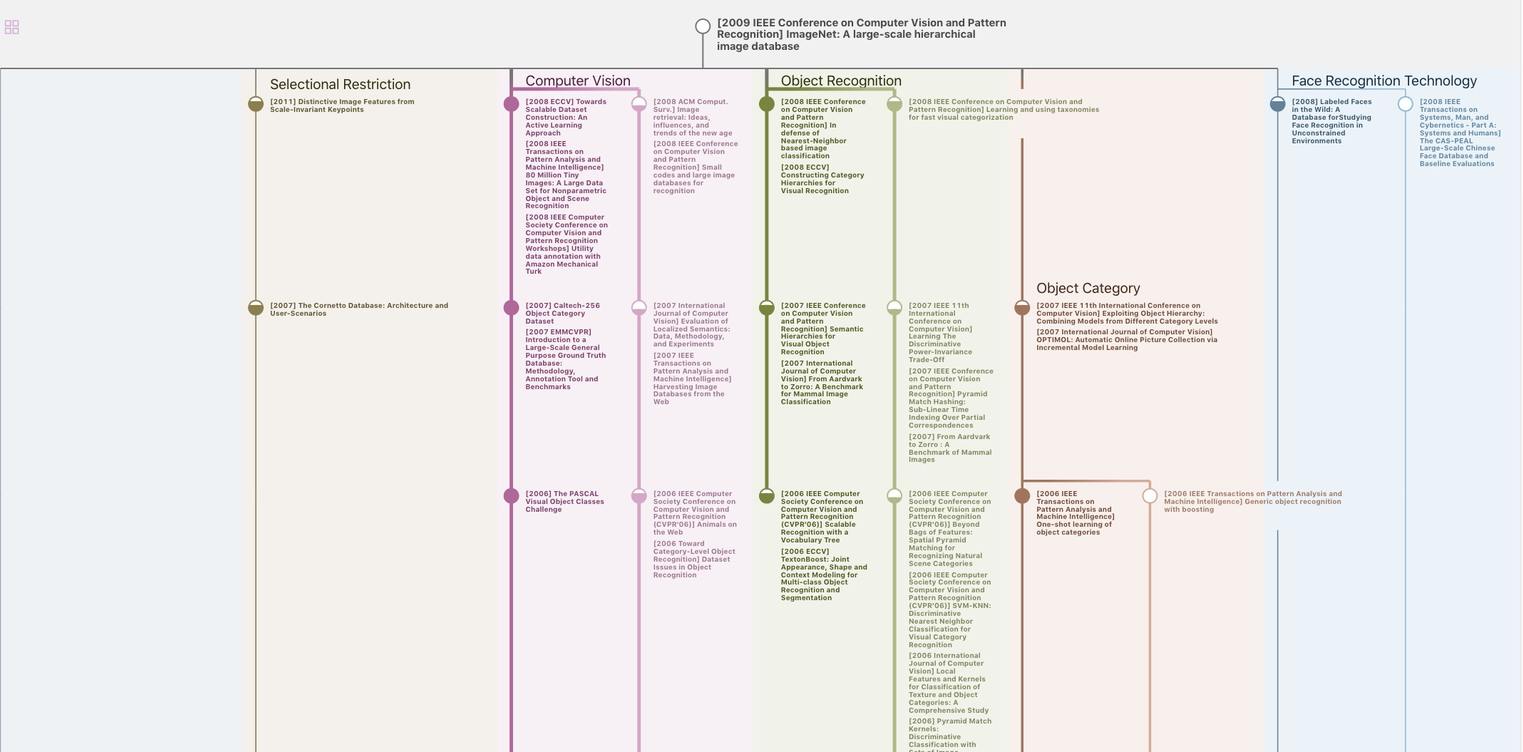
生成溯源树,研究论文发展脉络
Chat Paper
正在生成论文摘要