Comparator Networks
COMPUTER VISION - ECCV 2018, PT XI(2018)
摘要
The objective of this work is set-based verification, e.g. to decide if two sets of images of a face are of the same person or not. The traditional approach to this problem is to learn to generate a feature vector per image, aggregate them into one vector to represent the set, and then compute the cosine similarity between sets. Instead, we design a neural network architecture that can directly learn set-wise verification. Our contributions are: (i) We propose a Deep Comparator Network (DCN) that can ingest a pair of sets (each may contain a variable number of images) as inputs, and compute a similarity between the pair--this involves attending to multiple discriminative local regions (landmarks), and comparing local descriptors between pairs of faces; (ii) To encourage high-quality representations for each set, internal competition is introduced for recalibration based on the landmark score; (iii) Inspired by image retrieval, a novel hard sample mining regime is proposed to control the sampling process, such that the DCN is complementary to the standard image classification models. Evaluations on the IARPA Janus face recognition benchmarks show that the comparator networks outperform the previous state-of-the-art results by a large margin.
更多查看译文
关键词
Network Comparison, Weight Templates, Verification Template, ResNet, Front Mouth
AI 理解论文
溯源树
样例
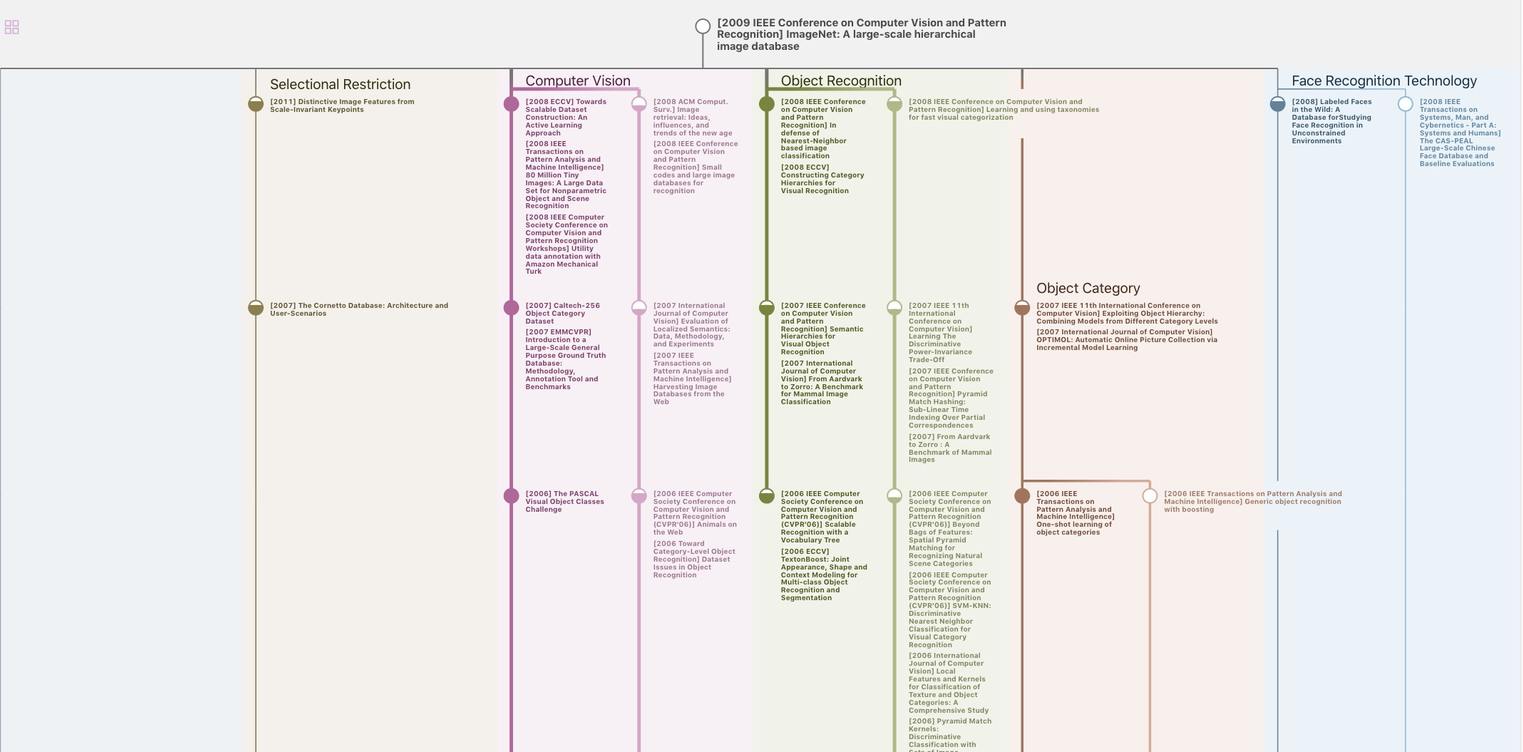
生成溯源树,研究论文发展脉络
Chat Paper
正在生成论文摘要