Mintin: Maxout-Based And Input-Normalized Transformation Invariant Neural Network
2018 25TH IEEE INTERNATIONAL CONFERENCE ON IMAGE PROCESSING (ICIP)(2018)
摘要
Convolutional Neural Network (CNN) is a powerful model for image classification, but it is insufficient to deal with the spatial variance of the input. This paper presents a Maxout-based and input-normalized transformation invariant neural network (MINTIN), which aims at addressing the nuisance variation of images and accumulating transformation invariance. We introduce an innovative module, the Normalization, and combine it with the Maxout operator. While the former focuses on each image itself, the latter pays attention to augmented versions of input, resulting in fully-utilized information. This combination, which can be inserted into existing CNN architectures, enables the network to learn invariance to rotation and scaling. While the authors of TI-POOLING acclaimed that they reached state-of-the-art results, ours reach a maximum decrease of 0.71%, 0.23% and 0.51% in error rate on MNIST-rot-12k, half-rotated MNIST and scaling MNIST, respectively. The size of the network is also significantly reduced, leading to high computational efficiency.
更多查看译文
关键词
transformation invariant, Normalization, Maxout
AI 理解论文
溯源树
样例
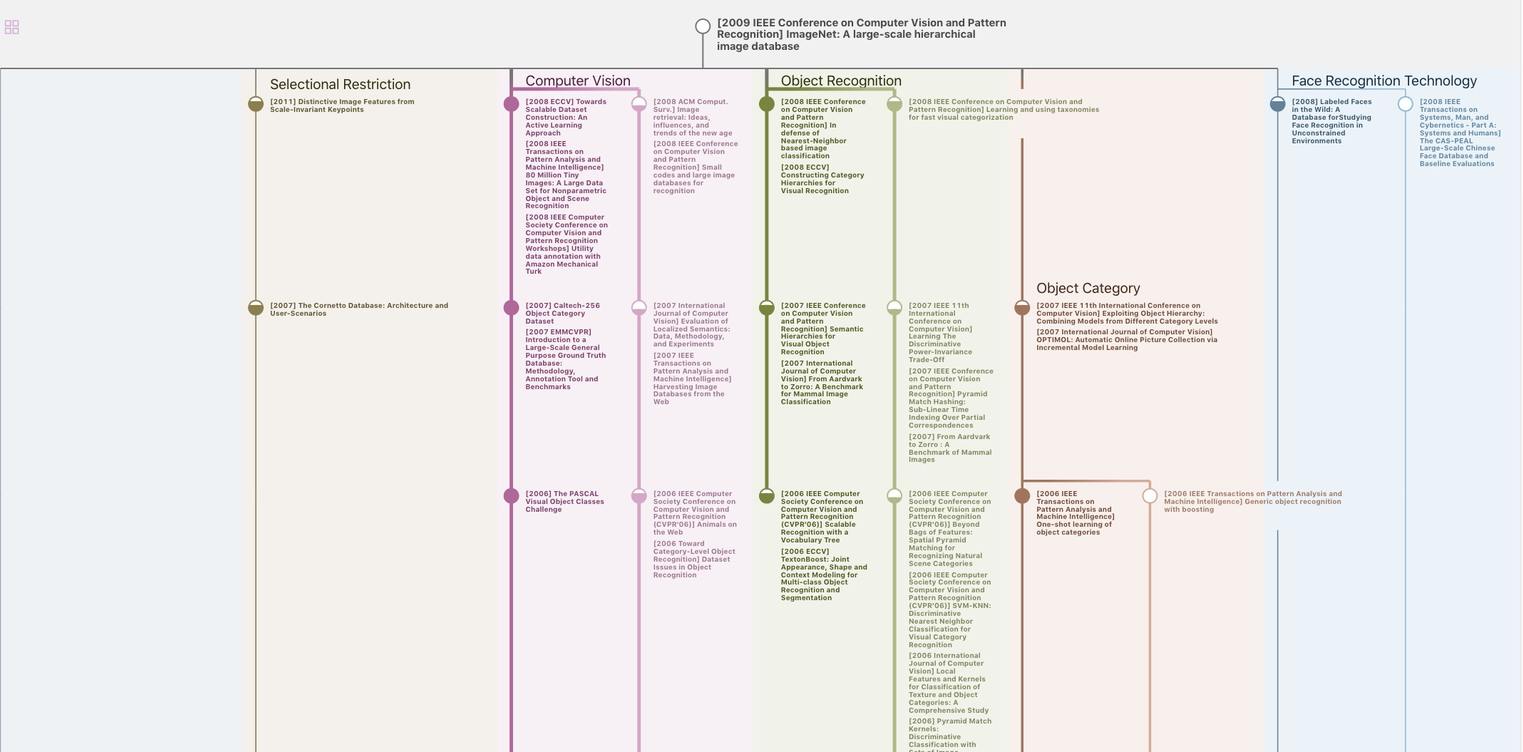
生成溯源树,研究论文发展脉络
Chat Paper
正在生成论文摘要