Theoretical Linear Convergence of Unfolded ISTA and its Practical Weights and Thresholds.
ADVANCES IN NEURAL INFORMATION PROCESSING SYSTEMS 31 (NIPS 2018)(2018)
摘要
In recent years, unfolding iterative algorithms as neural networks has become an empirical success in solving sparse recovery problems. However, its theoretical understanding is still immature, which prevents us from fully utilizing the power of neural networks. In this work, we study unfolded ISTA (Iterative Shrinkage Thresholding Algorithm) for sparse signal recovery. We introduce a weight structure that is necessary for asymptotic convergence to the true sparse signal. With this structure, unfolded ISTA can attain a linear convergence, which is better than the sublinear convergence of ISTA/FISTA in general cases. Furthermore, we propose to incorporate thresholding in the network to perform support selection, which is easy to implement and able to boost the convergence rate both theoretically and empirically. Extensive simulations, including sparse vector recovery and a compressive sensing experiment on real image data, corroborate our theoretical results and demonstrate their practical usefulness. We have made our codes publicly available.(2).
更多查看译文
关键词
neural networks,convergence rate,sublinear convergence,linear convergence,compressive sensing,sparse vector,sparse recovery
AI 理解论文
溯源树
样例
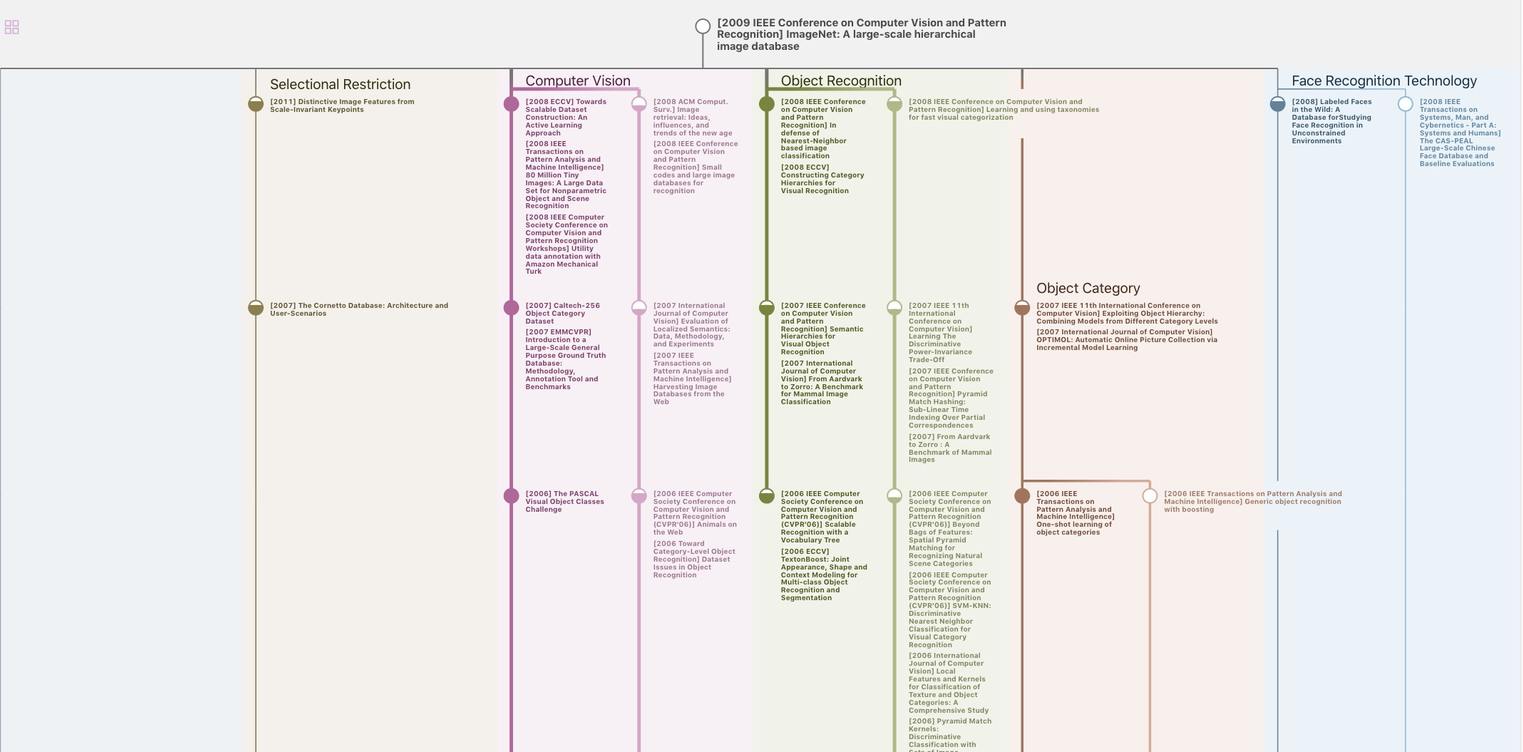
生成溯源树,研究论文发展脉络
Chat Paper
正在生成论文摘要