CMML: Contextual Modulation Meta Learning for Cold-Start Recommendation
Conference on Information and Knowledge Management(2021)
摘要
ABSTRACTPractical recommender systems experience a cold-start problem when observed user-item interactions in the history are insufficient. Meta learning, especially gradient based one, can be adopted to tackle this problem by learning initial parameters of the model and thus allowing fast adaptation to a specific task from limited data examples. Though with significant performance improvement, it commonly suffers from two critical issues: the non-compatibility with mainstream industrial deployment and the heavy computational burdens, both due to the inner-loop gradient operation. These two issues make them hard to be applied in practical recommender systems. To enjoy the benefits of meta learning framework and mitigate these problems, we propose a recommendation framework called Contextual Modulation Meta Learning (CMML). CMML is composed of fully feed-forward operations so it is computationally efficient and completely compatible with the mainstream industrial deployment. CMML consists of three components, including a context encoder that can generate context embedding to represent a specific task, a hybrid context generator that aggregates specific user-item features with task-level context, and a contextual modulation network, which can modulate the recommendation model to adapt effectively. We validate our approach on both scenario-specific and user-specific cold-start setting on various real-world datasets, showing CMML can achieve comparable or even better performance with gradient based methods yet with higher computational efficiency and better interpretability.
更多查看译文
关键词
contextual modulation meta learning,cold-start
AI 理解论文
溯源树
样例
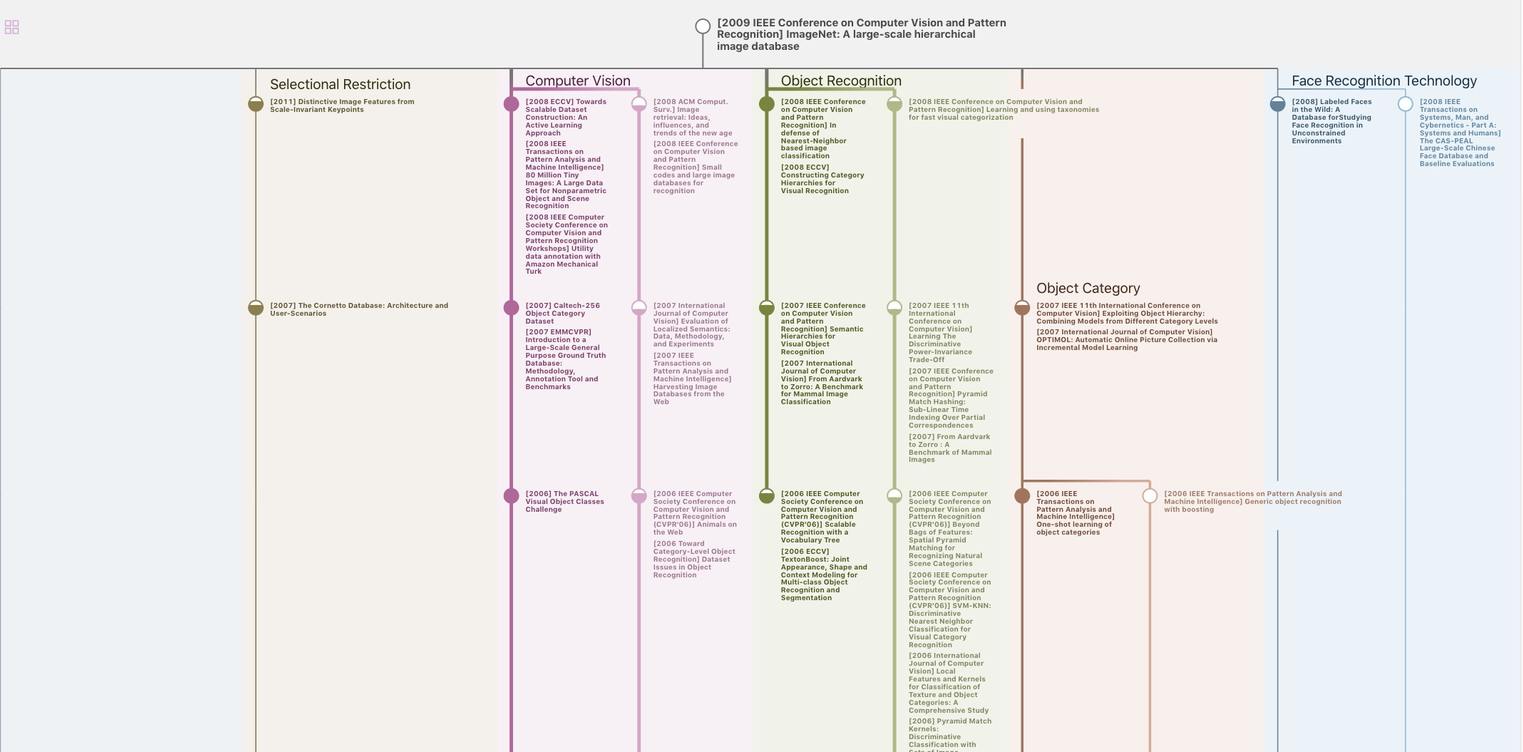
生成溯源树,研究论文发展脉络
Chat Paper
正在生成论文摘要