Efficient Stochastic Gradient Hard Thresholding.
ADVANCES IN NEURAL INFORMATION PROCESSING SYSTEMS 31 (NIPS 2018)(2018)
摘要
Stochastic gradient hard thresholding methods have recently been shown to work favorably in solving large-scale empirical risk minimization problems under sparsity or rank constraint. Despite the improved iteration complexity over full gradient methods, the gradient evaluation and hard thresholding complexity of the existing stochastic algorithms usually scales linearly with data size, which could still be expensive when data is huge and the hard thresholding step could be as expensive as singular value decomposition in rank-constrained problems. To address these deficiencies, we propose an efficient hybrid stochastic gradient hard thresholding (HSG-HT) method that can be provably shown to have sample-size-independent gradient evaluation and hard thresholding complexity bounds. Specifically, we prove that the stochastic gradient evaluation complexity of HSG-HT scales linearly with inverse of sub-optimality and its hard thresholding complexity scales logarithmically. By applying the heavy ball acceleration technique, we further propose an accelerated variant of HSG-HT which can be shown to have improved factor dependence on restricted condition number in the quadratic case. Numerical results confirm our theoretical affirmation and demonstrate the computational efficiency of the proposed methods.
更多查看译文
关键词
empirical risk minimization,singular value decomposition,efficient stochastic gradient hard thresholding
AI 理解论文
溯源树
样例
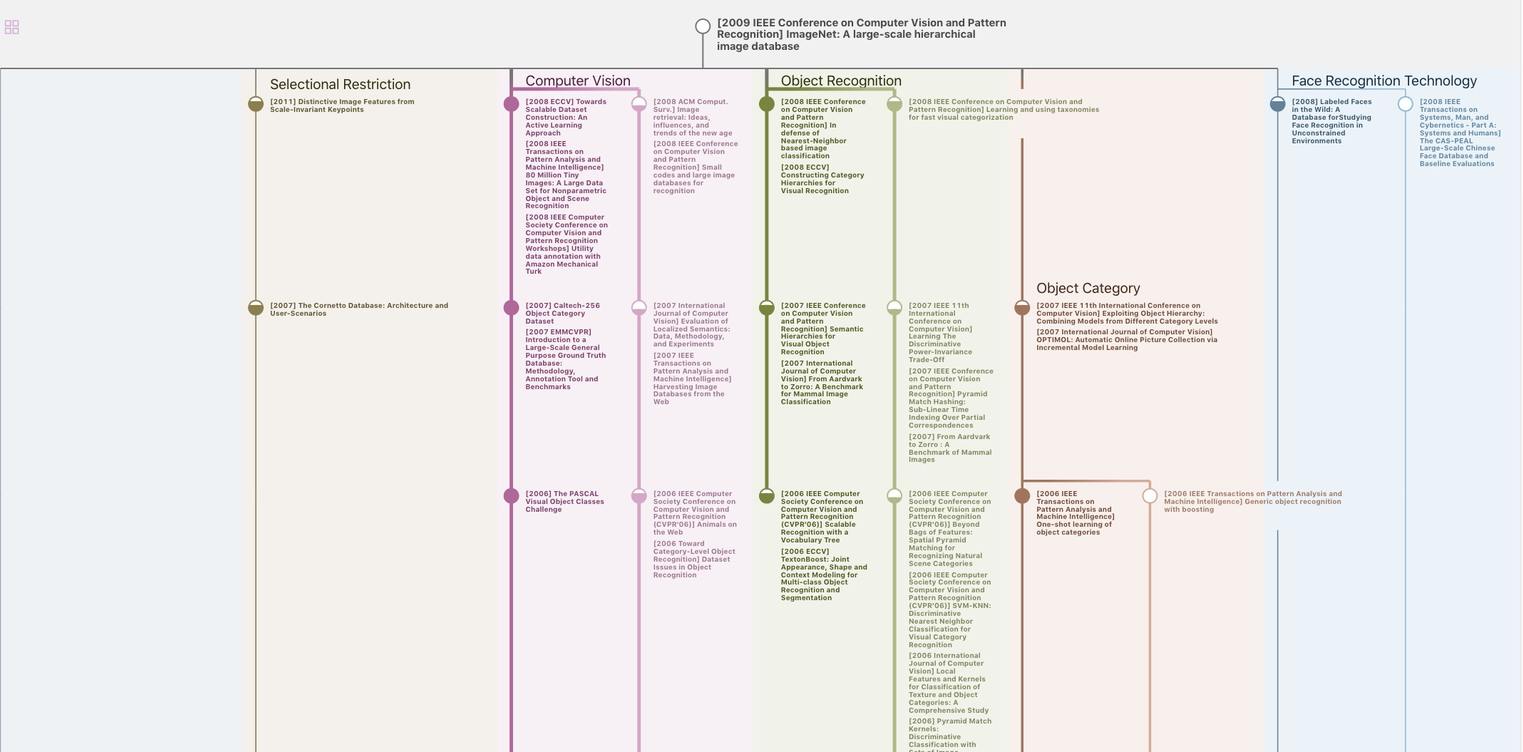
生成溯源树,研究论文发展脉络
Chat Paper
正在生成论文摘要