Irreversible samplers from jump and continuous Markov processes
Statistics and Computing(2018)
摘要
In this paper, we propose irreversible versions of the Metropolis–Hastings (MH) and Metropolis-adjusted Langevin algorithm (MALA) with a main focus on the latter. For the former, we show how one can simply switch between different proposal and acceptance distributions upon rejection to obtain an irreversible jump sampler (I-Jump). The resulting algorithm has a simple implementation akin to MH, but with the demonstrated benefits of irreversibility. We then show how the previously proposed MALA method can also be extended to exploit irreversible stochastic dynamics as proposal distributions in the I-Jump sampler. Our experiments explore how irreversibility can increase the efficiency of the samplers in different situations.
更多查看译文
关键词
Bayesian inference, Hamiltonian Monte Carlo, Irreversible samplers, Jump processes, Markov chain Monte Carlo, Metropolis–Hastings
AI 理解论文
溯源树
样例
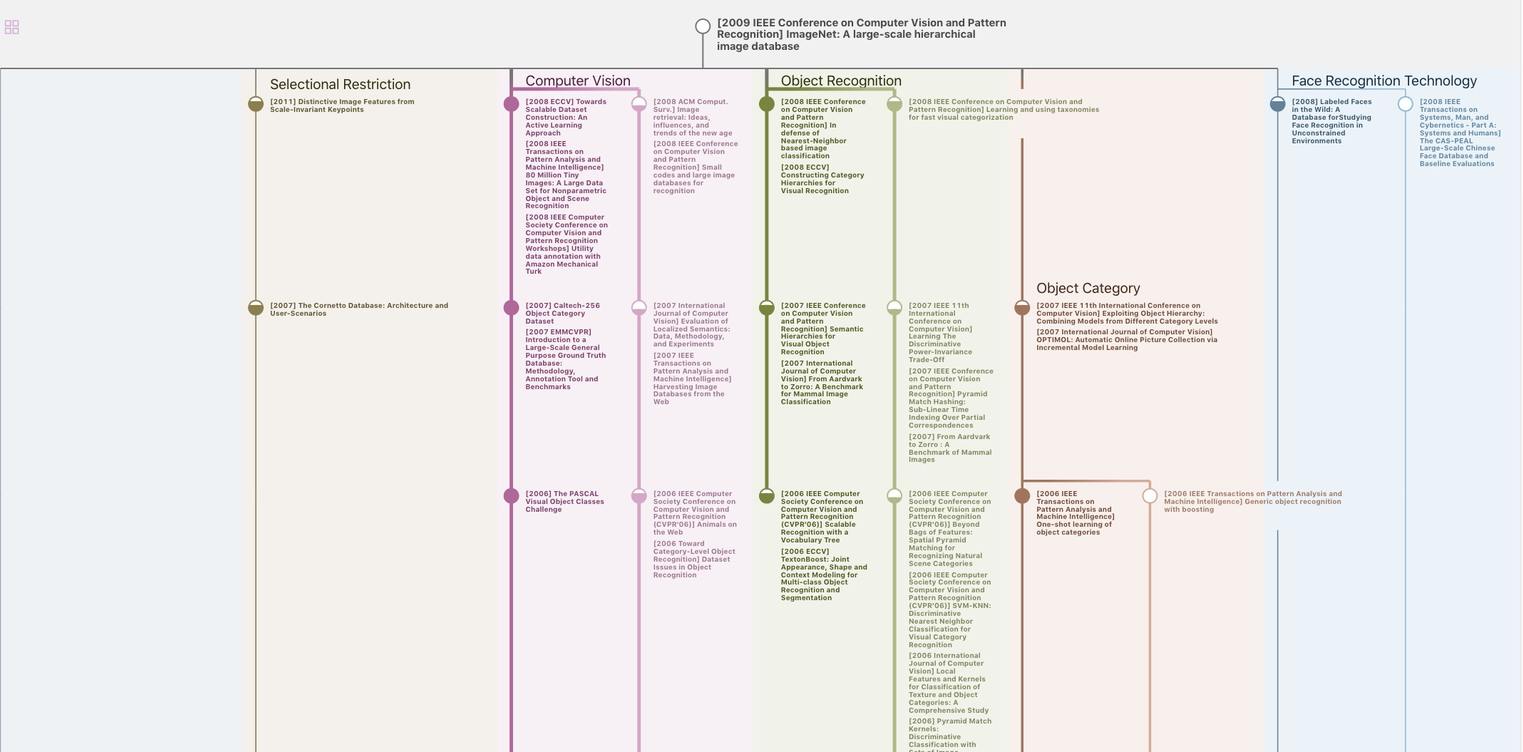
生成溯源树,研究论文发展脉络
Chat Paper
正在生成论文摘要