Stochastic trajectory optimization for mechanical systems with parametric uncertainties
arXiv: Optimization and Control(2017)
摘要
In this paper we develop a novel, discrete-time optimal control framework for mechanical systems with uncertain model parameters. We consider finite-horizon problems where the performance index depends on the statistical moments of the stochastic system. Our approach constitutes an extension of the original Differential Dynamic Programming method and handles uncertainty through generalized Polynomial Chaos (gPC) theory. The developed iterative scheme is capable of controlling the probabilistic evolution of the dynamic system and can be used in planning and control. Moreover, its scalable and fast converging nature play a key role when dealing with complex, high-dimensional problems. Based on Lagrangian mechanics principles, we also prove that Variational Integrators can be designed to properly propagate and linearize the gPC representations of stochastic, forced mechanical systems in discrete time. We utilize this benefit to further improve the efficiency of our trajectory-optimization methodology. Numerical simulations are included to validate the applicability of our approach.
更多查看译文
AI 理解论文
溯源树
样例
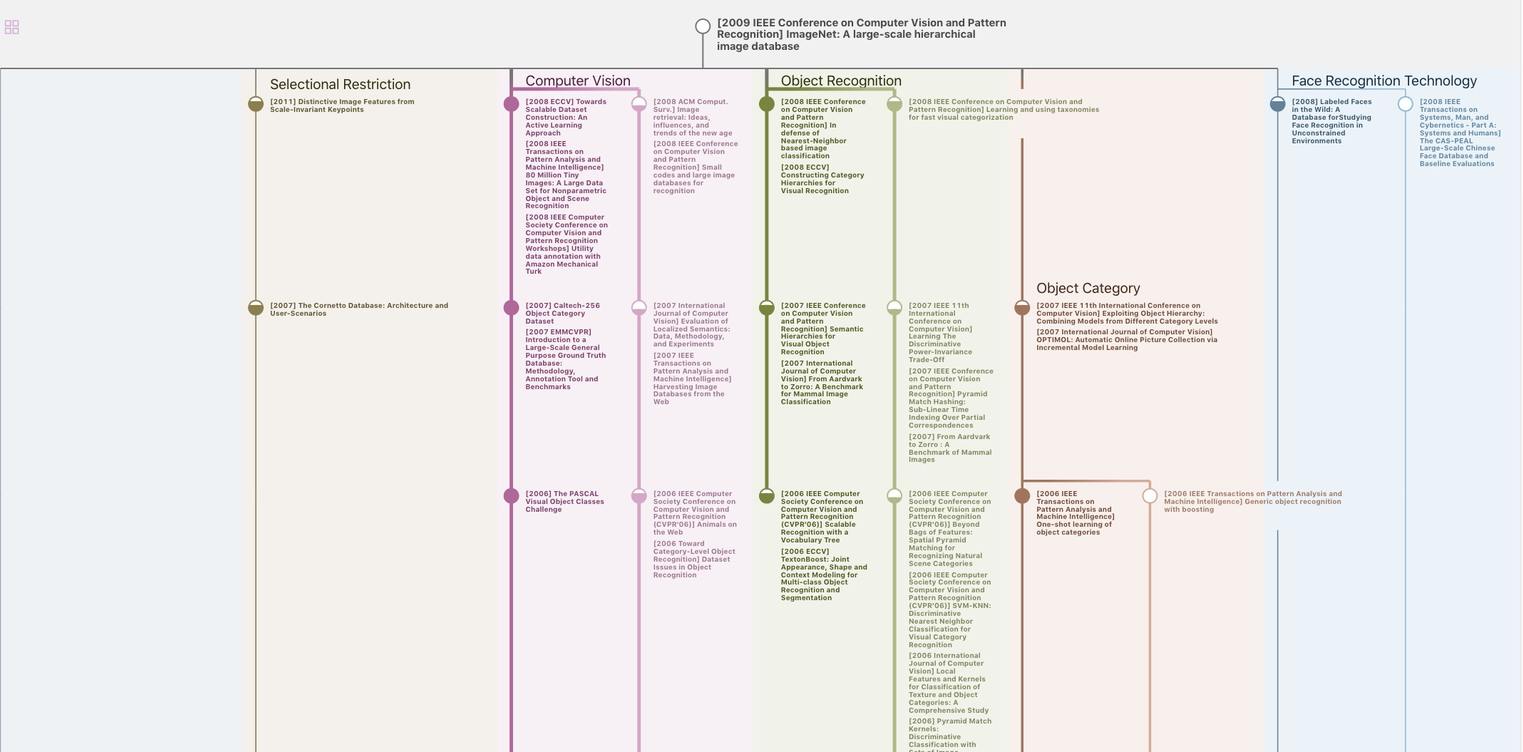
生成溯源树,研究论文发展脉络
Chat Paper
正在生成论文摘要