Robust High-Order Manifold Constrained Sparse Principal Component Analysis for Image Representation
IEEE Transactions on Circuits and Systems for Video Technology(2019)
摘要
In order to efficiently utilize the information in the data and eliminate the negative effects of outliers in the principal component analysis (PCA) method, in this paper, we propose a novel robust sparse PCA method based on maximum correntropy criterion (MCC) with high-order manifold constraints called the RHSPCA. Compared with the traditional PCA methods, the proposed RHSPCA has the following benefits: 1) the MCC regression term is more robust to outliers than the MSE-based regression term; 2) thanks to the high-order manifold constraints, the low-dimensional representations can preserve the local relations of the data and greatly improve the clustering and classification performance for image processing tasks; and 3) in order to further counteract the adverse effects of outliers, the MCC-based samples’ mean is proposed to better centralize the data. We also propose a new solver based on the half-quadratic technique and accelerated block coordinate update strategy to solve the RHSPCA model. Extensive experimental results show that the proposed method can outperform the state-of-the-art robust PCA methods on a variety of image processing tasks, including reconstruction, clustering, and classification, on outliers contaminated datasets.
更多查看译文
关键词
Principal component analysis,Robustness,Manifolds,Kernel,Image reconstruction,Task analysis
AI 理解论文
溯源树
样例
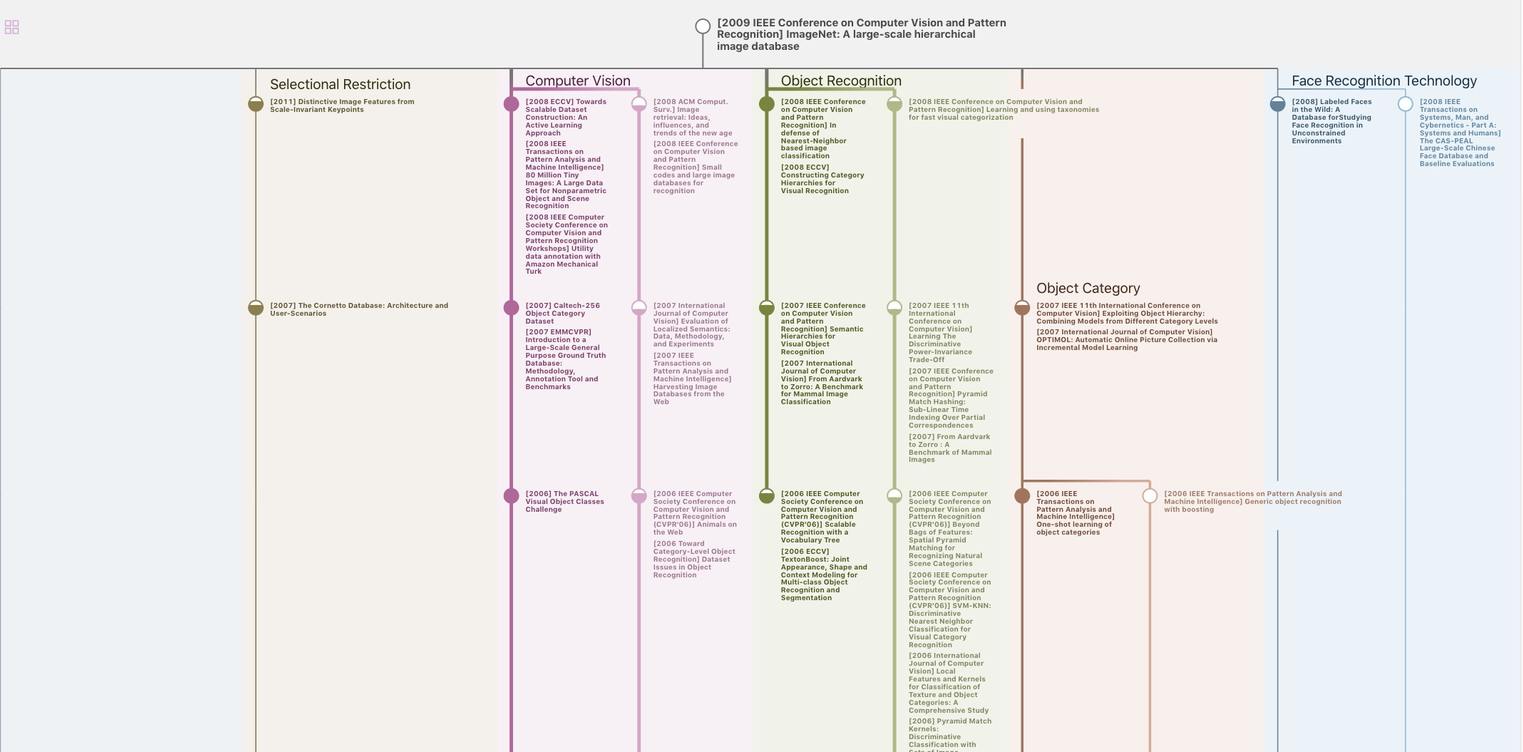
生成溯源树,研究论文发展脉络
Chat Paper
正在生成论文摘要