Speaker Adaptation for Speech Synthesis Based on Deep Neural Networks Using Hidden Semi-Markov Model Structures
2018 Asia-Pacific Signal and Information Processing Association Annual Summit and Conference (APSIPA ASC)(2018)
摘要
This paper proposes a speaker adaptation technique for speech synthesis-based deep neural networks (DNNs) using hidden semi-Markov model (HSMM) structures. Speaker adaptation techniques for DNN-based speech synthesis are based on fixed time-alignments estimated by external aligners. Therefore, the acoustic features and temporal structures of speech are separately adapted in speaker adaptation. In this work, a special type of mixture density network (MDN) called MDN-HSMM, which outputs the parameters of HSMMs, is applied. The proposed method can model not only acoustic features but also durations in a unified framework and perform speaker adaptation that considers temporal structures. Experimental results show that the proposed method improves the naturalness and speaker similarity of the synthesized speech compared to the speaker adaptation based on DNNs.
更多查看译文
AI 理解论文
溯源树
样例
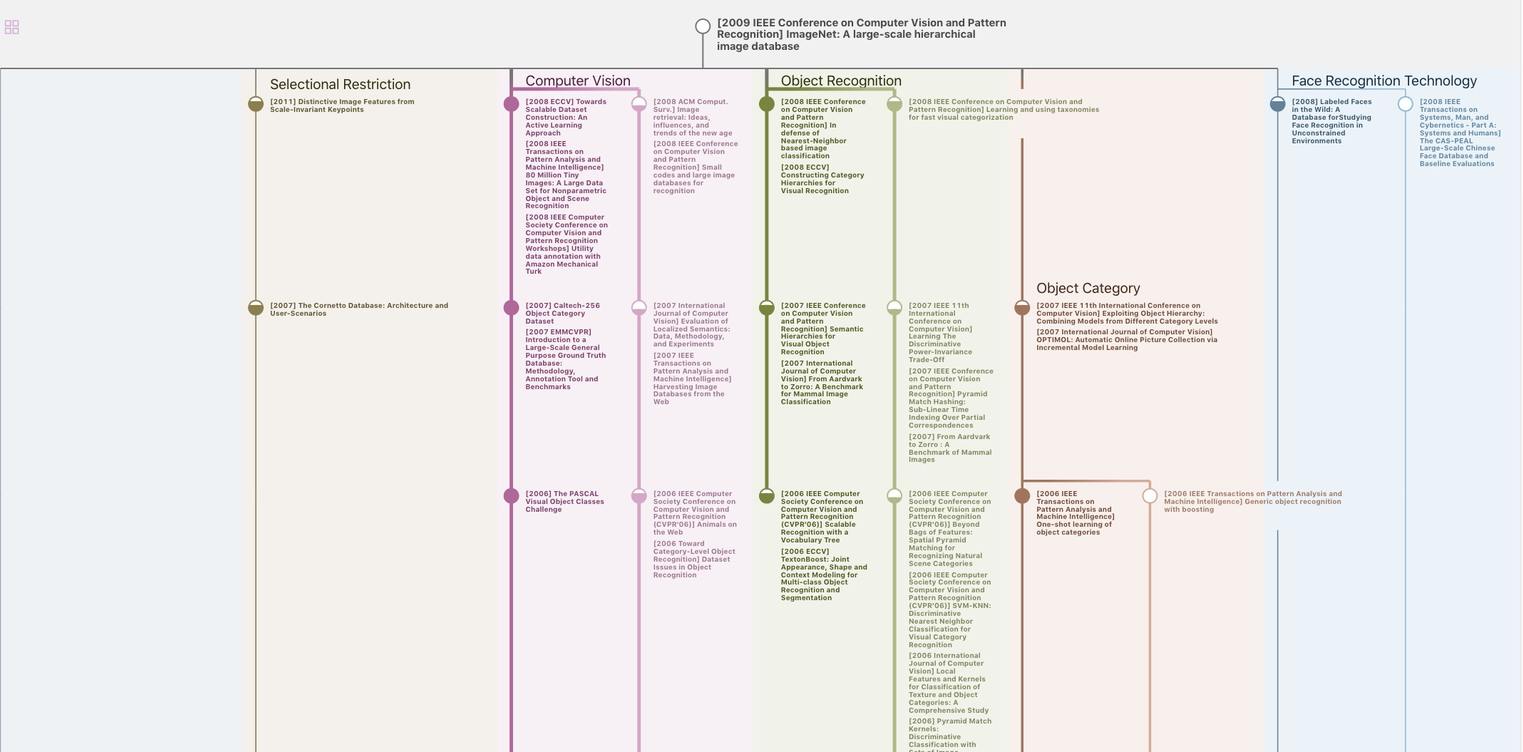
生成溯源树,研究论文发展脉络
Chat Paper
正在生成论文摘要