Accurate 3D Face Reconstruction With Weakly-Supervised Learning: From Single Image to Image Set
2019 IEEE/CVF Conference on Computer Vision and Pattern Recognition Workshops (CVPRW)(2019)
摘要
Recently, deep learning based 3D face reconstruction methods have shown promising results in both quality and efficiency. However, training deep neural networks typically requires a large volume of data, whereas face images with ground-truth 3D face shapes are scarce. In this paper, we propose a novel deep 3D face reconstruction approach that 1) leverages a robust, hybrid loss function for weakly-supervised learning which takes into account both low-level and perception-level information for supervision, and 2) performs multi-image face reconstruction by exploiting complementary information from different images for shape aggregation. Our method is fast, accurate, and robust to occlusion and large pose. We provide comprehensive experiments on MICC Florence and Facewarehouse datasets, systematically comparing our method with fifteen recent methods and demonstrating its state-of-the-art performance. Code available at https://github.com/Microsoft/Deep3DFaceReconstruction.
更多查看译文
关键词
accurate 3D face reconstruction,weakly-supervised learning,single image,image set,deep learning,3D face reconstruction methods,deep neural network training,face images,ground-truth 3D face shapes,novel deep 3D face reconstruction approach,robust loss function,hybrid loss function,perception-level information,multiimage face reconstruction,shape aggregation,low-level information,MICC Florence datasets,Facewarehouse datasets
AI 理解论文
溯源树
样例
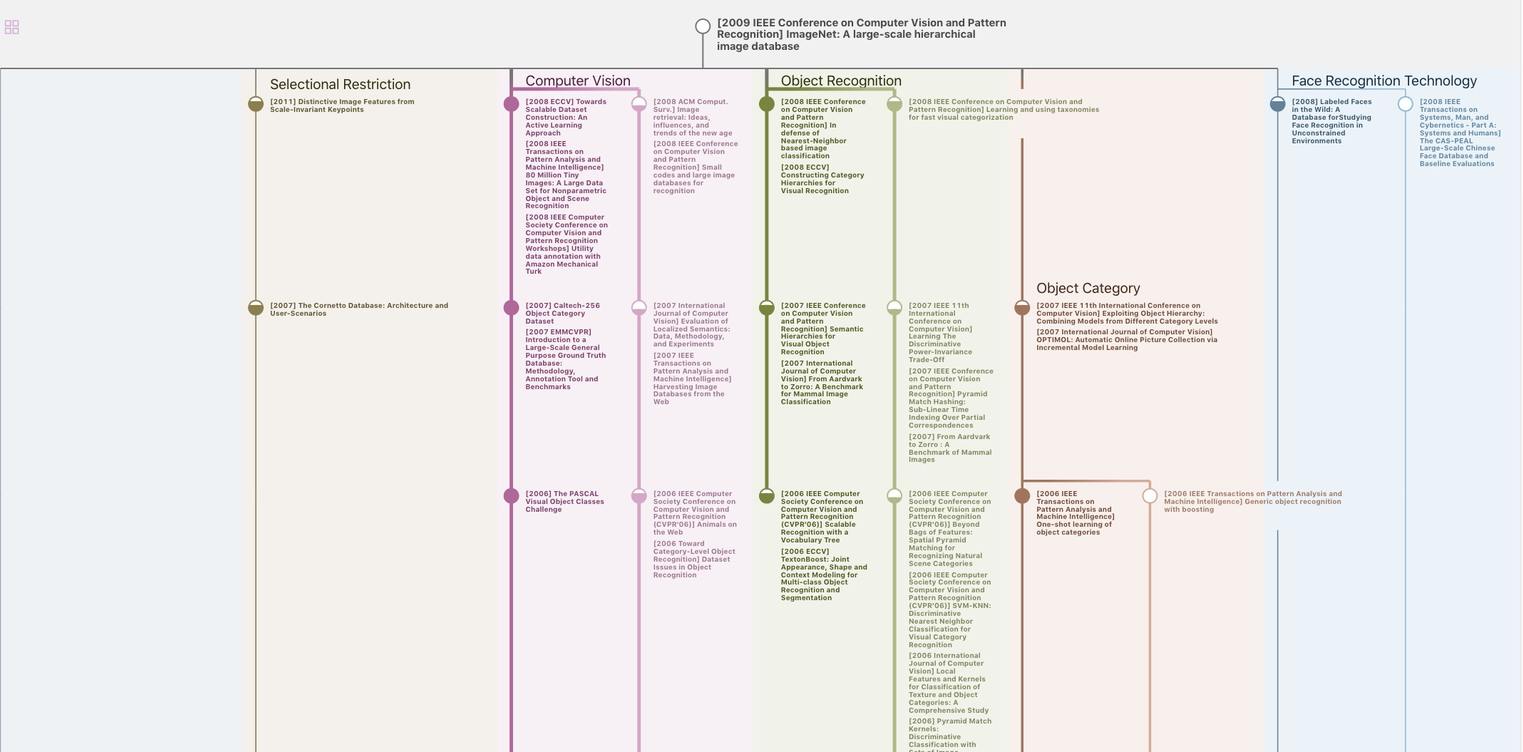
生成溯源树,研究论文发展脉络
Chat Paper
正在生成论文摘要