Speeding up person finding using hidden Markov models.
Robotics and Autonomous Systems(2019)
摘要
The ability of mobile service robots to efficiently search for a person is needed in a vast domain of applications. The search problem is especially challenging when the user is freely moving across the environment, the robot has only a constrained field of view, and visibility constraints arise from the environment. We propose in this article a novel approach that simulates the user’s presence at different locations in the environment based on a hidden Markov model (HMM). The HMM predicts the user’s motion and computes the observability likelihood at the different locations given the predictions. Our approach then selects effective search locations that maximize the user’s expected observability. The selection criterion hereby considers the visibility constraints along the robot’s path as well as the robot’s travel time to reach the search location. We performed both real-world and extensive simulated experiments to evaluate our method. In comparison to a greedy maximum coverage approach as well as to a greedy strategy that uses background information, we show that our framework leads to a significant reduction of the time needed to find the user.
更多查看译文
关键词
Modeling human motion,Motion prediction,Hidden Markov models,Information gain based search
AI 理解论文
溯源树
样例
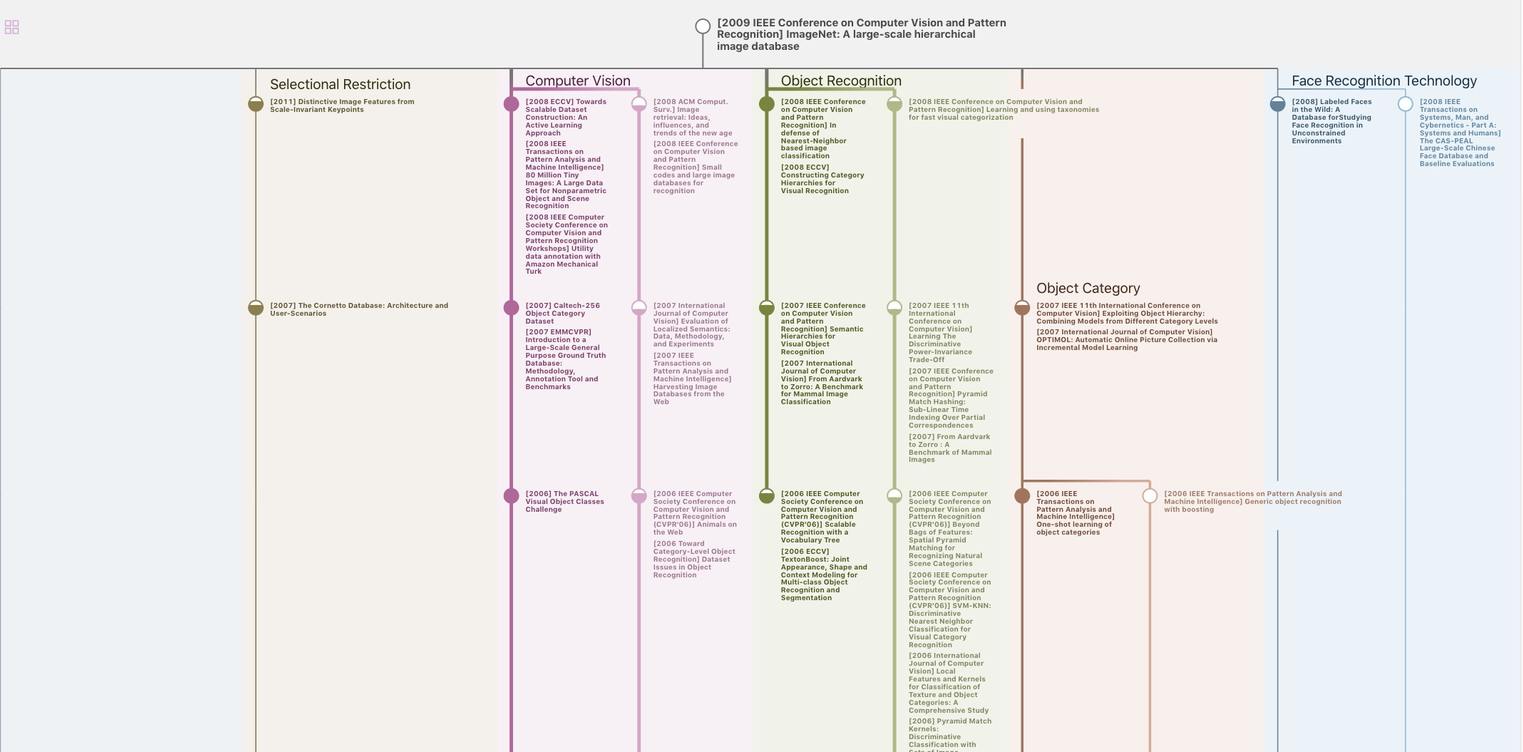
生成溯源树,研究论文发展脉络
Chat Paper
正在生成论文摘要